A Novel Clustering Based on Consensus Knowledge for Cross-Domain Fault Diagnoses.
IEEE Trans. Instrum. Meas.(2023)
摘要
In recent years, cross-domain fault diagnosis problems based on knowledge transfer have attracted considerable attention from researchers, some of whom have adopted domain adaptation algorithms for model transfer under various working conditions. Such algorithms typically assume the samples of the target and source domains share the same fault mode sets and that the source and target domains are interrelated. However, such prior knowledge is difficult to obtain in actual industrial fault diagnoses. To address this issue, we propose a universal domain adaptation network model based on consensus knowledge (CKUAN). This model adopts cluster matching and domain consensus scores to mine effective knowledge from the class and sample levels, thus eliminating the dependence on prior knowledge of the label set. Furthermore, we introduced a contrastive domain discrepancy (CDD) to jointly optimize clustering. Experimental results on two diagnostic datasets demonstrate the proposed CKUAN method to be robust and effective, and it yields higher performance than existing state-of-the-art cross-domain fault diagnosis methods.
更多查看译文
关键词
Consensus knowledge,contrastive domain discrepancy (CDD),domain adaptation,fault diagnosis,unsupervised clustering
AI 理解论文
溯源树
样例
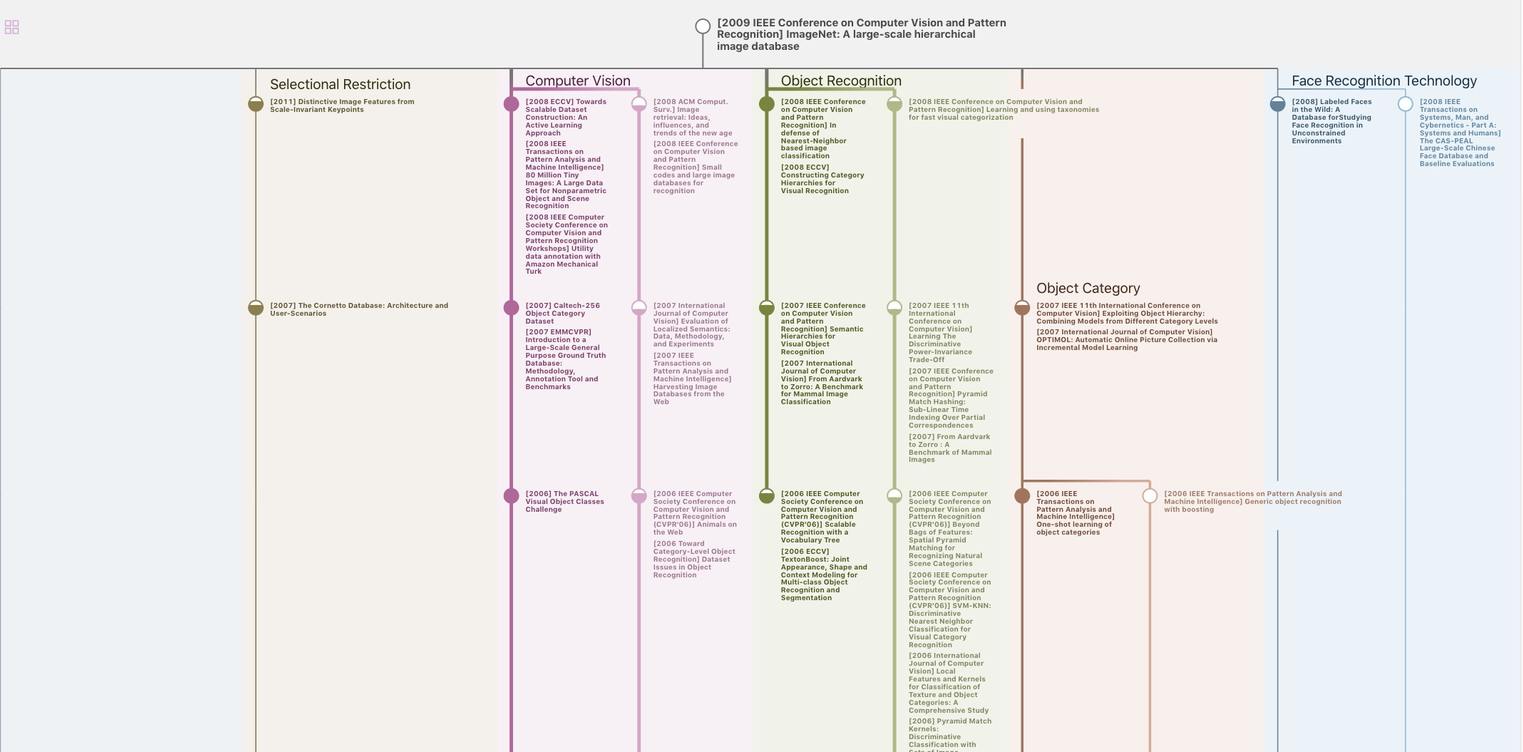
生成溯源树,研究论文发展脉络
Chat Paper
正在生成论文摘要