Surrogate-assisted Parameter Re-initialization for Differential Evolution.
SSCI(2022)
摘要
With respect to parameter adaptation in metaheuristic algorithms, a kind of feedback usually exists between parameter adaptation and individuals' survival. It is known that parameters in reproduction operator largely determine the quality of offspring. As feedback, successful survival of individuals can guide the direction in which parameters adapt. However, parameter adaptation only based on the preference of survivors would easily get premature convergence. To alleviate this issue, a parameter re-initialization strategy is proposed to regenerate more reasonable starting points at different stages for parameter adaptation. In this study, support vector regression with radial basis function is used to estimate potential parameter combinations for JADE. The IEEE CEC2014 test suite including 30 benchmark test functions is used to evaluate our proposed strategy. Obtained results are compared with six state-of-theart optimization algorithms. Statistical analysis demonstrates the effectiveness of the proposed surrogate-assisted parameter reinitialization strategy.
更多查看译文
关键词
global optimization, differential evolution, surrogate model, parameter re-initialization
AI 理解论文
溯源树
样例
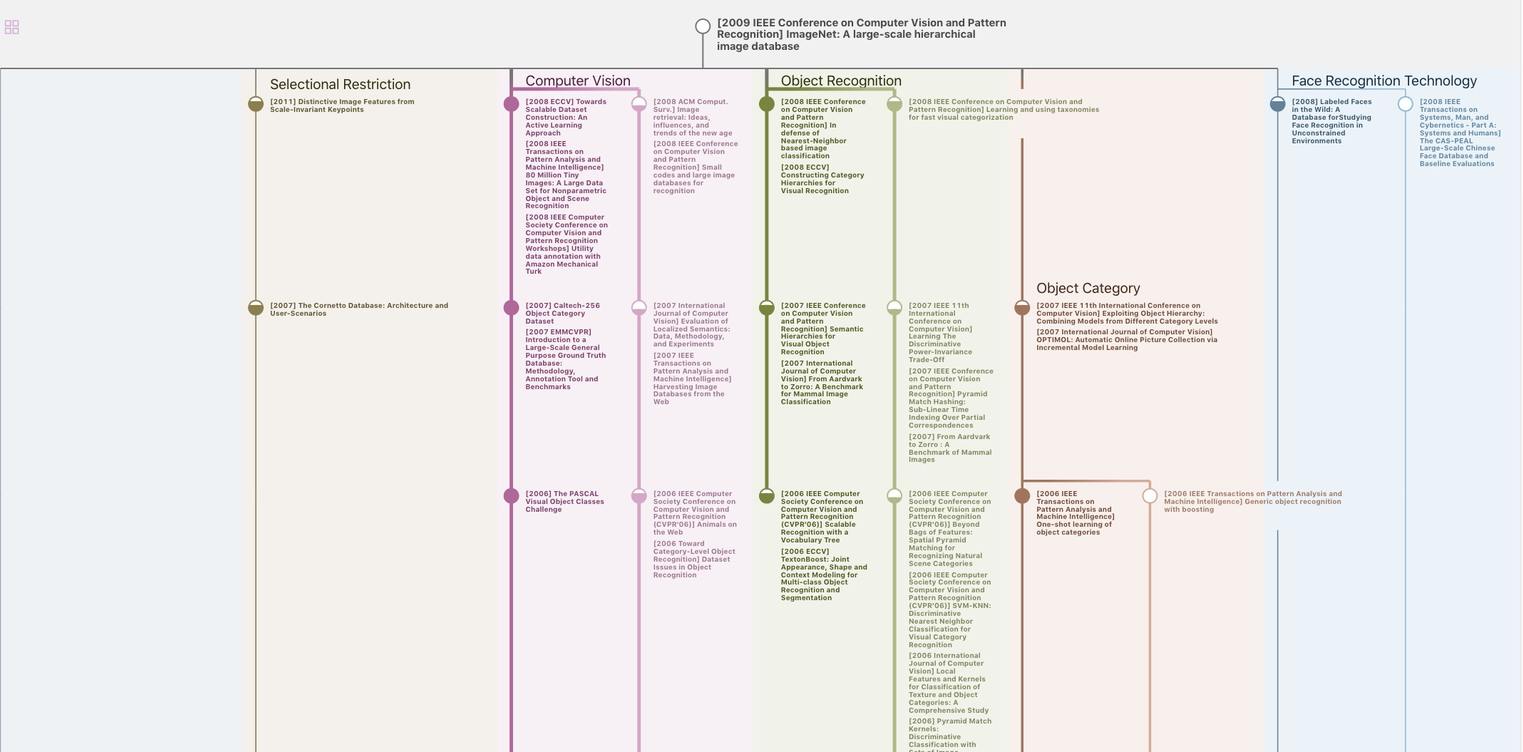
生成溯源树,研究论文发展脉络
Chat Paper
正在生成论文摘要