Reduced Precision Research of a GAN Image Generation Use-case.
ICPRAM (Revised Selected Papers)(2022)
摘要
In this research a deep convolutional Generative Adversarial Network (GAN) model is post-training quantized to a reduced precision arithmetic for a complex High Energy Physics (HEP) use-case. This research is motivated by the aim to decrease the necessary model size and computing time for reducing the required hardware resources for future Large Hadron Collider (LHC) detector simulations at CERN. However, in order to interpret the measured physics results, the detector simulations have to maintain the highest possible accuracy. Therefore, the quantized model is not only in detail analyzed in terms of hardware resource consumption but additionally comprehensively evaluated in terms of the achieved physics accuracy. We report that we achieve with the quantized model a 3.0x speed-up versus the initial model on modern CPUs. Furthermore, we investigate several new physics accuracy metrics to demonstrate that the accuracy does not significantly decrease due to the quantization process. Reduced precision computing for classification problems is already adequately studied, however, this is not the case for more complex image generation problems as we require for our use-case of detector simulations in this research. By using the Intel Neural Compressor, the quantization is performed in an iterative process. Neural Compressor automatically quantizes only the parameters of the neural network which do not decrease the accuracy of the model regarding a predefined accuracy metric. In our research we post-training quantize the GAN model from the 32-bit format down to 8-bit format.
更多查看译文
关键词
Reduced precision computing, Generative Adversarial Networks (GAN), Neural Compressor, HEP simulation, Quantization
AI 理解论文
溯源树
样例
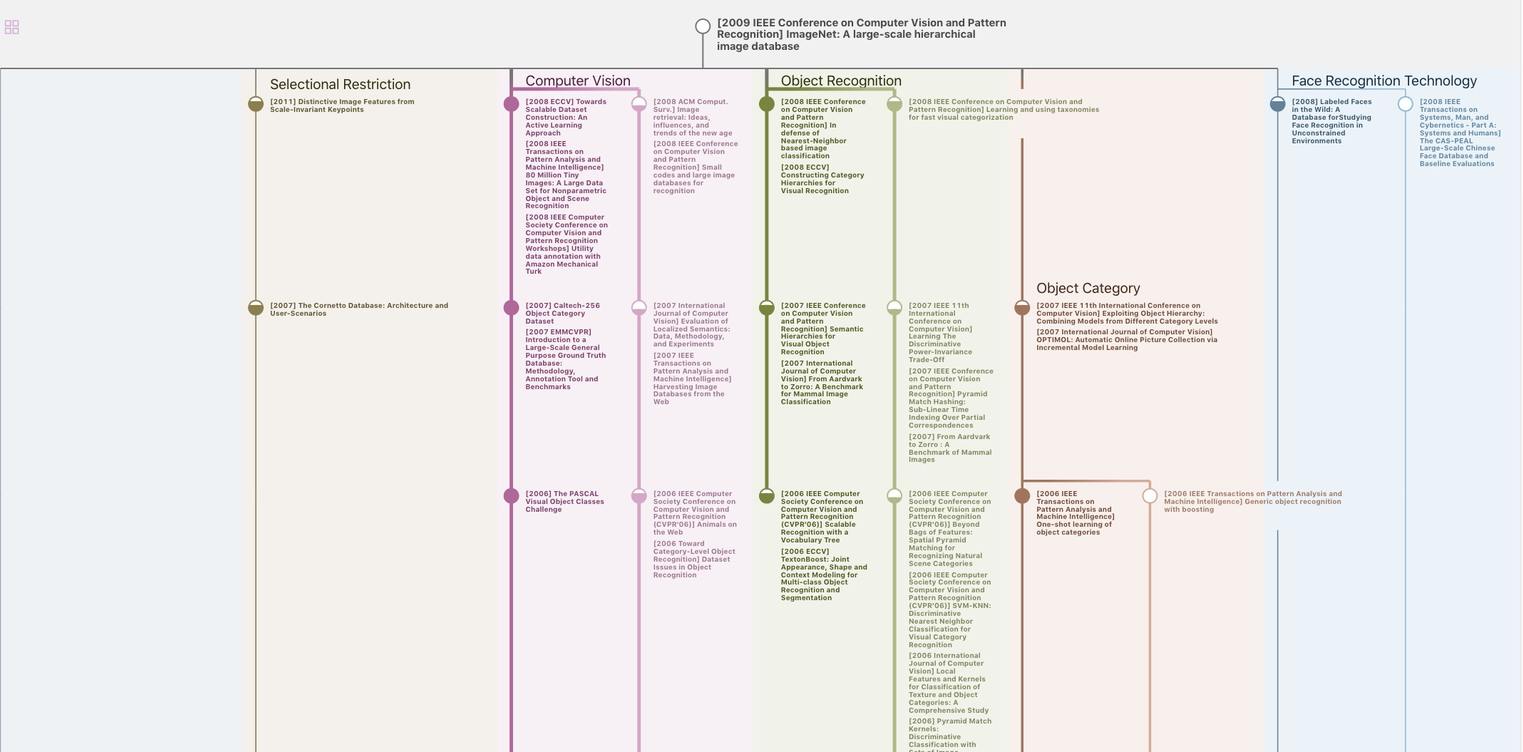
生成溯源树,研究论文发展脉络
Chat Paper
正在生成论文摘要