ToSA: A Top-Down Tree Structure Awareness Model for Hierarchical Text Classification.
APWeb/WAIM (2)(2022)
摘要
Hierarchical text classification (HTC) is a challenging task that classifies textual descriptions with a taxonomic hierarchy. Existing methods have difficulties in modeling the hierarchical label structure. They focus on using the graph embedding methods to encode the hierarchical structure, ignoring that the HTC labels are based on a tree structure. There is a difference between tree and graph structure: in the graph structure, message passing is undirected, which will lead to the imbalance of message transmission between nodes when applied to HTC. As the nodes in different layers have inheritance relationships, the information transmission between nodes should be directional and hierarchical in the HTC task. In this paper, we propose a Top-Down Tree Structure Awareness Model to extract the hierarchical structure features, called ToSA. We regard HTC as a sequence generation task and introduce a priori hierarchical information in the decoding process. We block the information flow in one direction to ensure the graph embedding method is more suitable for the HTC task, then get the enhanced tree structure representation. Experiment results show that our model can achieve the best results on both the public WOS dataset and a collected E-commerce user intent classification dataset 3 .
更多查看译文
关键词
hierarchical text classification,tree structure awareness model,text classification,top-down
AI 理解论文
溯源树
样例
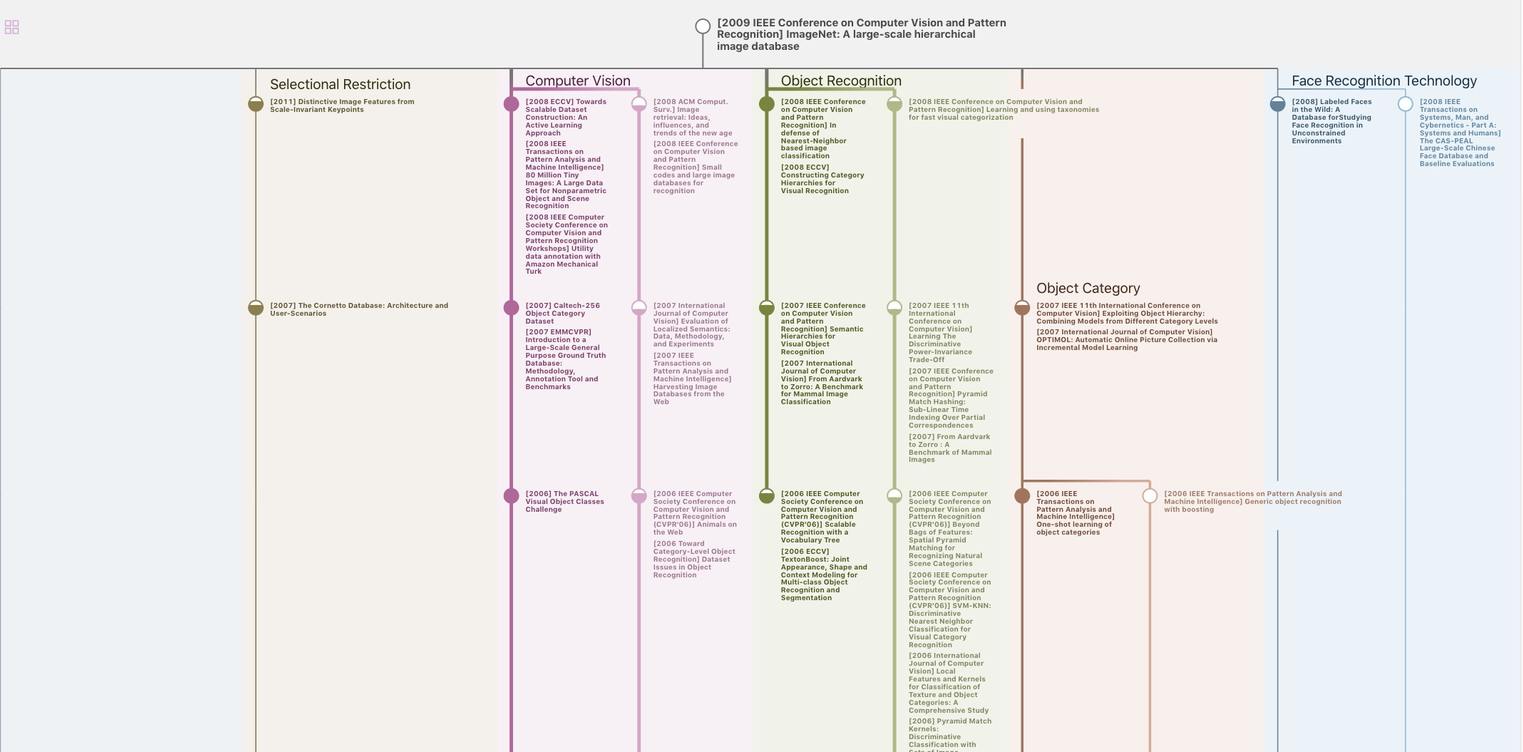
生成溯源树,研究论文发展脉络
Chat Paper
正在生成论文摘要