Heterogeneous Graph Based Long- And Short-Term Preference Learning Model for Next POI Recommendation.
ICA3PP(2022)
摘要
The next POI recommendation aiming at recommending the venues that people are likely interested in has become a popular service provided by location-based social networks such as Foursquare and Gowalla. Many existing methods attempt to improve the recommendation accuracy by modeling the long- and short-term preferences of people. However, these methods learn users’ preferences only from their own historical check-in records, which leads to bad recommendation performance in sparse dataset. To this end, we propose a novel approach named long- and short-term preference learning model based on heterogeneous graph convolution network and attention mechanism (LSPHGA) for next POI recommendation. Specifically, we design a heterogeneous graph convolution network to learn the higher-order structural relations between User-POI-Categories and obtain the long-term preferences of users. As for the short-term preference, we encode the recent check-in records of users through self-attention mechanism and aggregate the short-term preference by spatio-temporal attention. Finally, the long- and short-term preference is linearly combined into a unified preference with personalized weights for different users. Extensive experiments on two real-world datasets consistently validate the effectiveness of the proposed method for improving recommendation.
更多查看译文
关键词
POI recommendation, Long- and short-term preference, Graph neural network, Attention mechanism, Spatio-temporal context
AI 理解论文
溯源树
样例
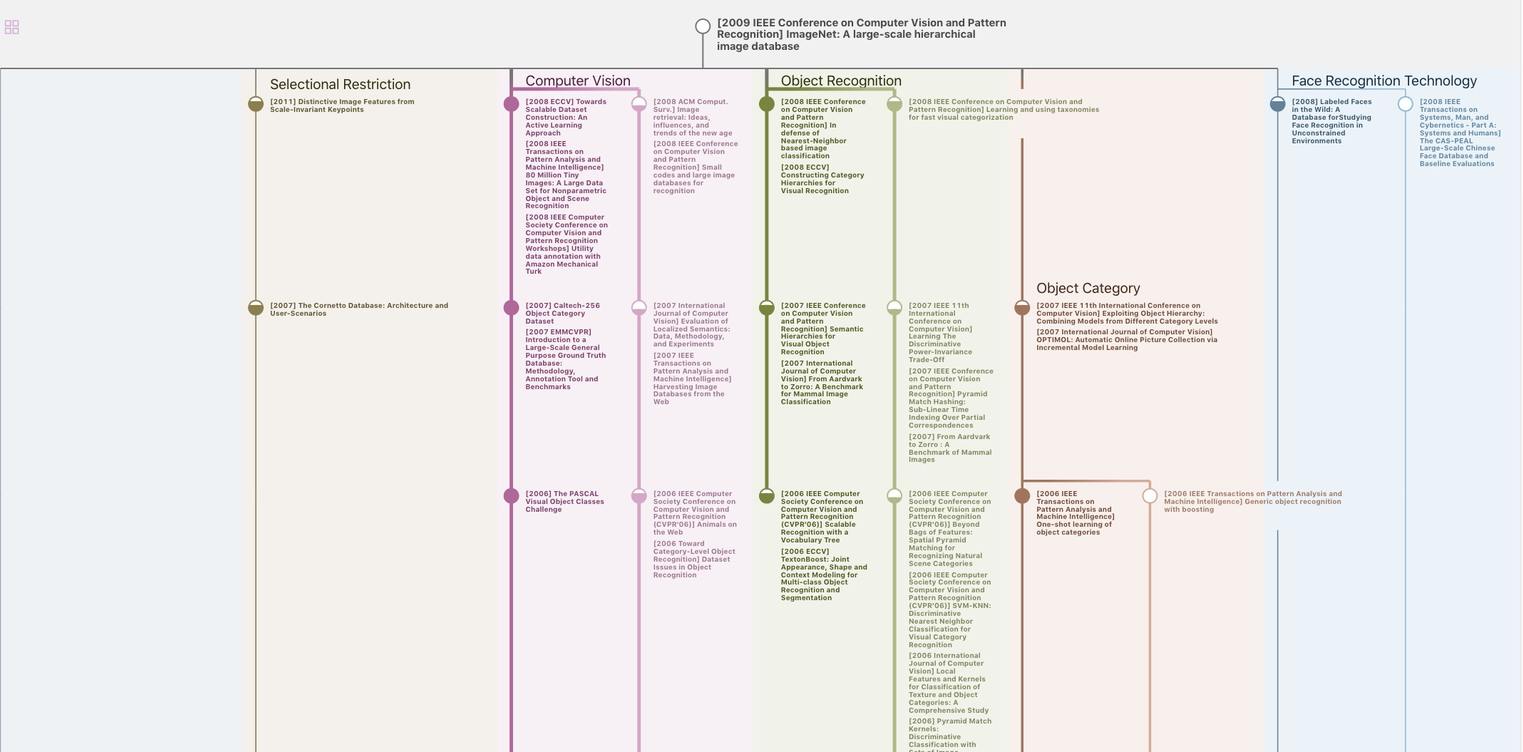
生成溯源树,研究论文发展脉络
Chat Paper
正在生成论文摘要