Specific Energy Consumption Prediction Framework in Hydrogen Liquefaction Process Using Deep Neural Network Based on Quantum Mechanics.
SCIS/ISIS(2022)
摘要
Energy supply methods are being diversified due to competition for resources due to depletion of fossil fuels and continued high oil prices. Hydrogen energy has the advantage of low carbon dioxide emission and the ability to generate a lot of energy per weight. However, gaseous hydrogen has a low hydrogen storage density per unit volume, so a liquefaction process is required. Liquid hydrogen has a lower pressure than hydrogen in gaseous state, so the risk of explosion is low and transportation cost is reduced because it is easy to transport in large quantities. Therefore, the hydrogen liquefaction process is important and can improve energy efficiency. Specific Energy Consumption (SEC), commonly used as a performance indicator in the hydrogen liquefaction process, is the sum of energy consumed in the entire process to liquefy 1 kg of hydrogen. However, since the uncertainty of the attribute values lowers the accuracy of prediction, in order to overcome this issue, this study proposes a framework for predicting the SEC value according to the attribute value using a Deep Neural Network (DNN) based on quantum mechanics. The attribute values corrected through quantum mechanics more closely simulate the attribute of the data and contribute to improving the prediction accuracy.
更多查看译文
关键词
Deep Neural Network,Stochastic Processes,Quantum Mechanics,Specific Energy Consumption Prediction,Hydrogen Liquefaction Process
AI 理解论文
溯源树
样例
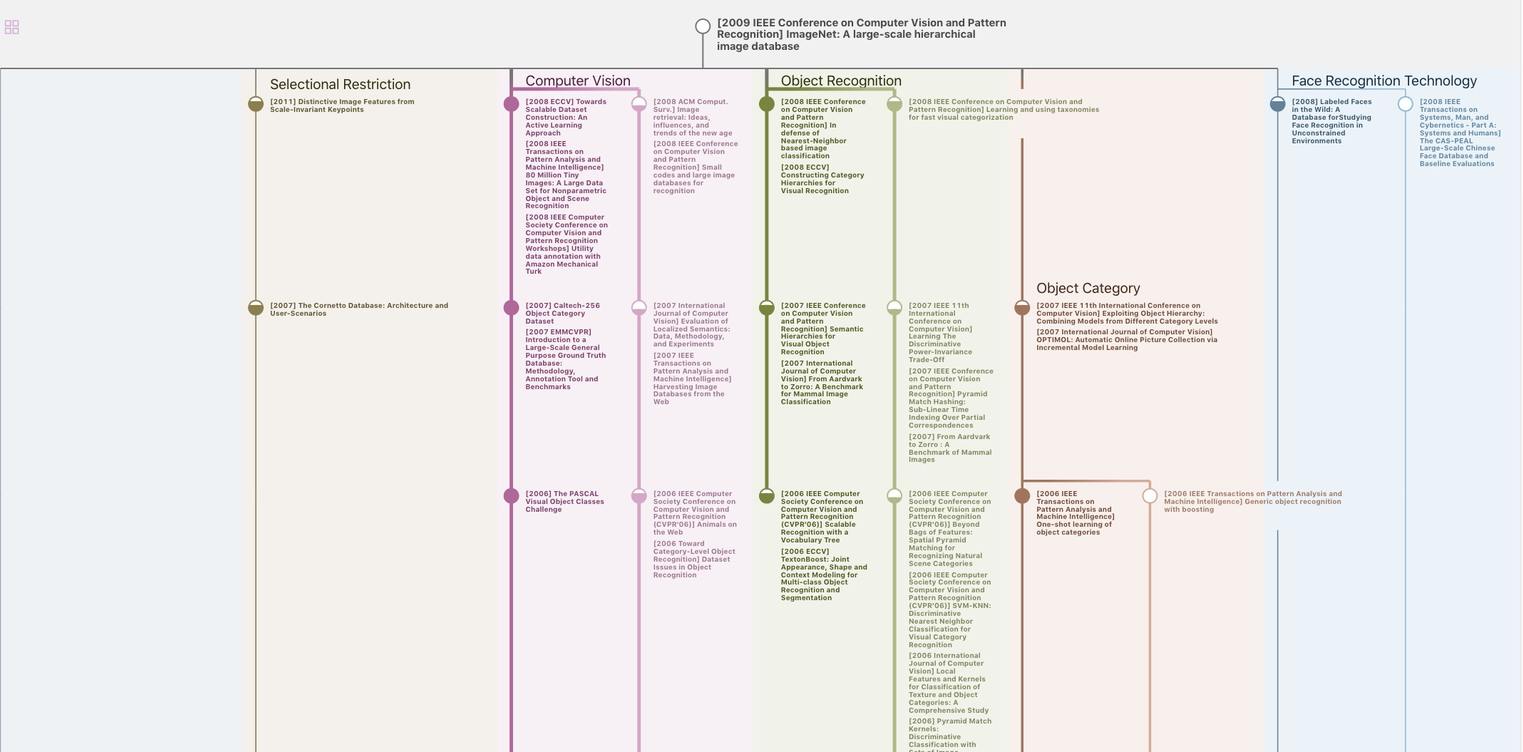
生成溯源树,研究论文发展脉络
Chat Paper
正在生成论文摘要