Generating Explainable Product Comparisons for Online Shopping.
WSDM(2023)
摘要
An essential part of making shopping purchase decisions is to compare and contrast products based on key differentiating features, but doing this manually can be overwhelming. Prior methods offer limited product comparison capabilities, e.g., via pre-defined common attributes that may be difficult to understand, or irrelevant to a particular product or user. Automatically generating an informative, natural-sounding, and factually consistent comparative text for multiple product and attribute types is a challenging research problem. We describe HCPC (Human Centered Product Comparison), to tackle two kinds of comparisons for online shopping: (i) product-specific, to describe and compare products based on their key attributes; and (ii) attribute-specific comparisons, to compare similar products on a specific attribute. To ensure that comparison text is faithful to the input product data, we introduce a novel multi-decoder, multi-task generative language model. One decoder generates product comparison text, and a second one generates supportive, explanatory text in the form of product attribute names and values. The second task imitates a copy mechanism, improving the comparison generator, and its output is used to justify the factual accuracy of the generated comparison text, by training a factual consistency model to detect and correct errors in the generated comparative text. We release a new dataset (https://registry.opendata.aws/) of ~15K human generated sentences, comparing products on one or more attributes (the first such data we know of for product comparison). We demonstrate on this data that HCPC significantly outperforms strong baselines, by ~10% using automatic metrics, and ~5% using human evaluation.
更多查看译文
AI 理解论文
溯源树
样例
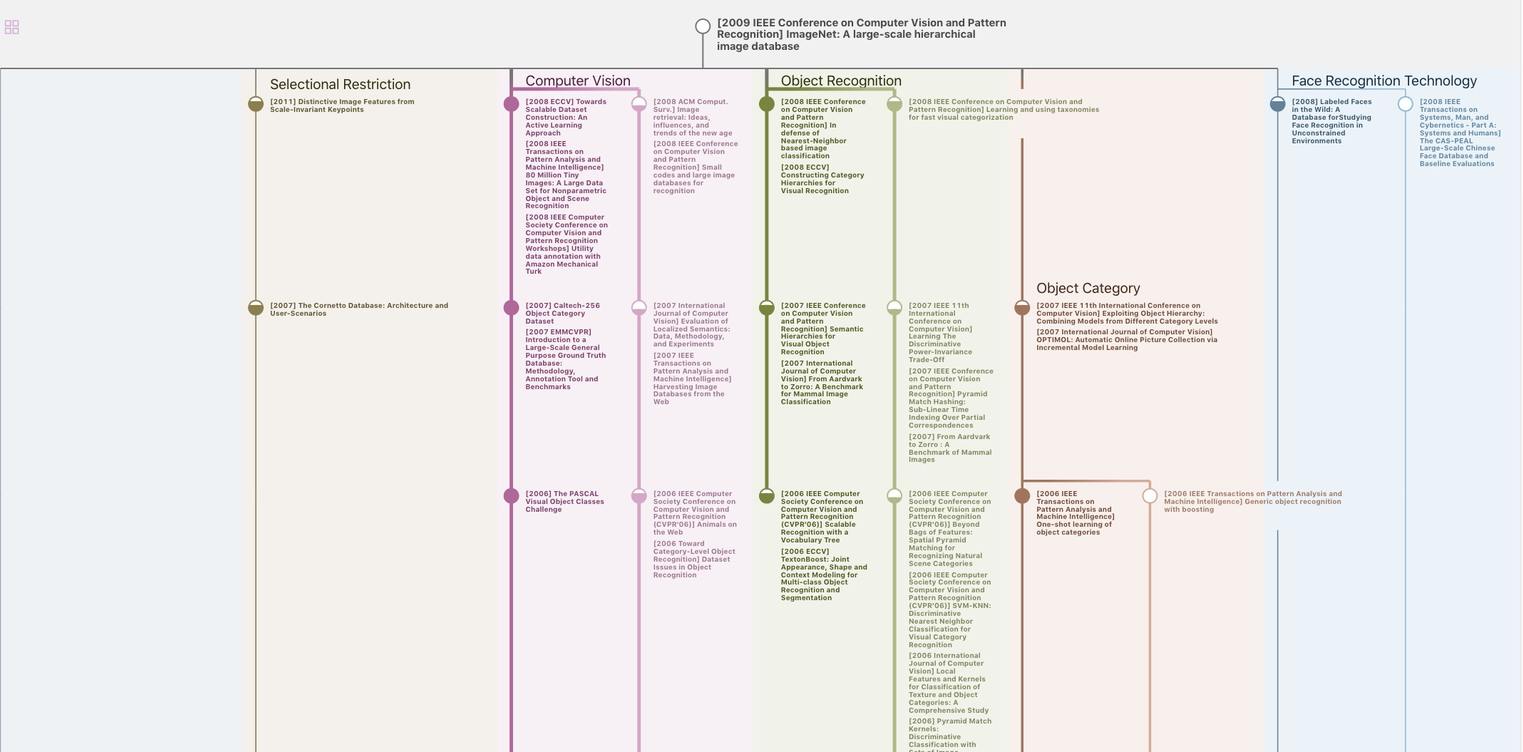
生成溯源树,研究论文发展脉络
Chat Paper
正在生成论文摘要