Sample Complexity Analysis and Self-regularization in Identification of Over-parameterized ARX Models.
CDC(2022)
摘要
AutoRegressive eXogenous (ARX) models form one of the most important model classes in control theory, econometrics, and statistics, but they are yet to be understood in terms of their finite sample identification analysis. The technical challenges come from the strong statistical dependency not only between data samples at different time instances but also between elements within each individual sample. In this work, for ARX models with potentially unknown orders, we study how ordinary least squares (OLS) estimator performs in terms of identifying model parameters from data collected from either a single length-T trajectory or N i.i.d. trajectories. Our main results show that as long as the orders of the model are chosen optimistically, i.e., we are learning an over-parameterized model compared to the ground truth ARX, the OLS will converge with the optimal rate O(1/root T) (or O(1/root N)) to the true (low-order) ARX parameters. This occurs without the aid of any regularization, thus is referred to as self-regularization. Our results imply that the oracle knowledge of the true orders and usage of regularizers are not necessary in learning ARX models - over-parameterization is all you need.
更多查看译文
关键词
ARX parameters,autoregressive exogenous models,control theory,data samples,econometrics,finite sample identification analysis,model classes,model parameters,N i.i.d. trajectories,OLS,optimal rate,oracle knowledge,ordinary least squares estimator,over-parameterized ARX models,sample complexity analysis,self-regularization,single length-T trajectory,statistics,strong statistical dependency,time instances
AI 理解论文
溯源树
样例
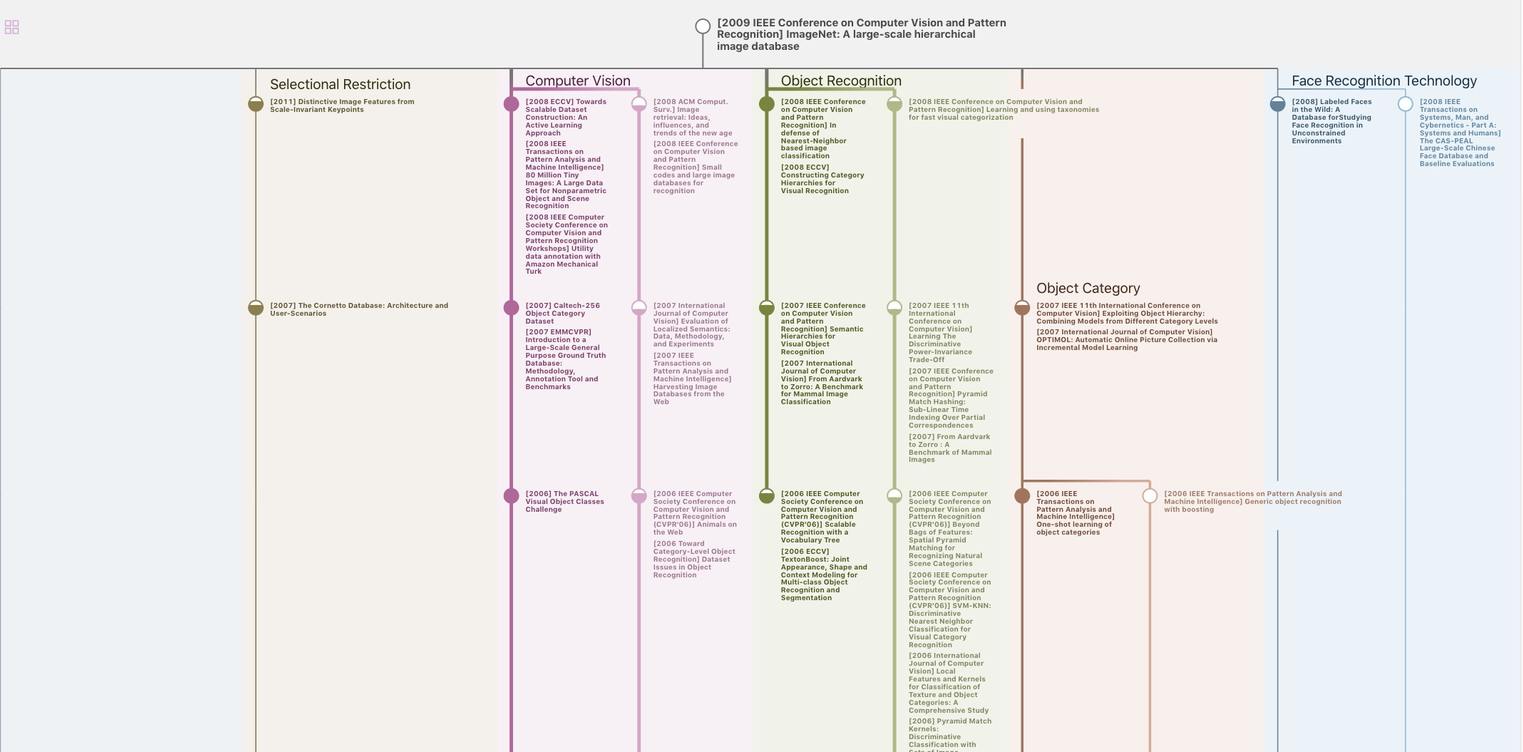
生成溯源树,研究论文发展脉络
Chat Paper
正在生成论文摘要