Stochastic Approximation for Identification of Non-Linear Differential-Algebraic Equations with Process Disturbances.
CDC(2022)
摘要
Differential-algebraic equations, commonly used to model physical systems, are the basis for many equation-based object-oriented modeling languages. When systems described by such equations are influenced by unknown process disturbances, estimating unknown parameters from experimental data becomes difficult. This is because of problems with the existence of well-defined solutions and the computational tractability of estimators. In this paper, we propose a way to minimize a cost function-whose minimizer is a consistent estimator of the true parameters-using stochastic gradient descent. This approach scales significantly better with the number of unknown parameters than other currently available methods for the same type of problem. The performance of the method is demonstrated through a simulation study with three unknown parameters. The experiments show a significantly reduced variance of the estimator, compared to an output error method neglecting the influence of process disturbances, as well as an ability to reduce the estimation bias of parameters that the output error method particularly struggles with.
更多查看译文
关键词
consistent estimator,cost function-whose minimizer,currently available methods,equation-based object-oriented,nonlinear differential-algebraic equations,output error method,parameters-using stochastic gradient descent,physical systems,stochastic approximation,unknown parameters,unknown process disturbances,well-defined solutions
AI 理解论文
溯源树
样例
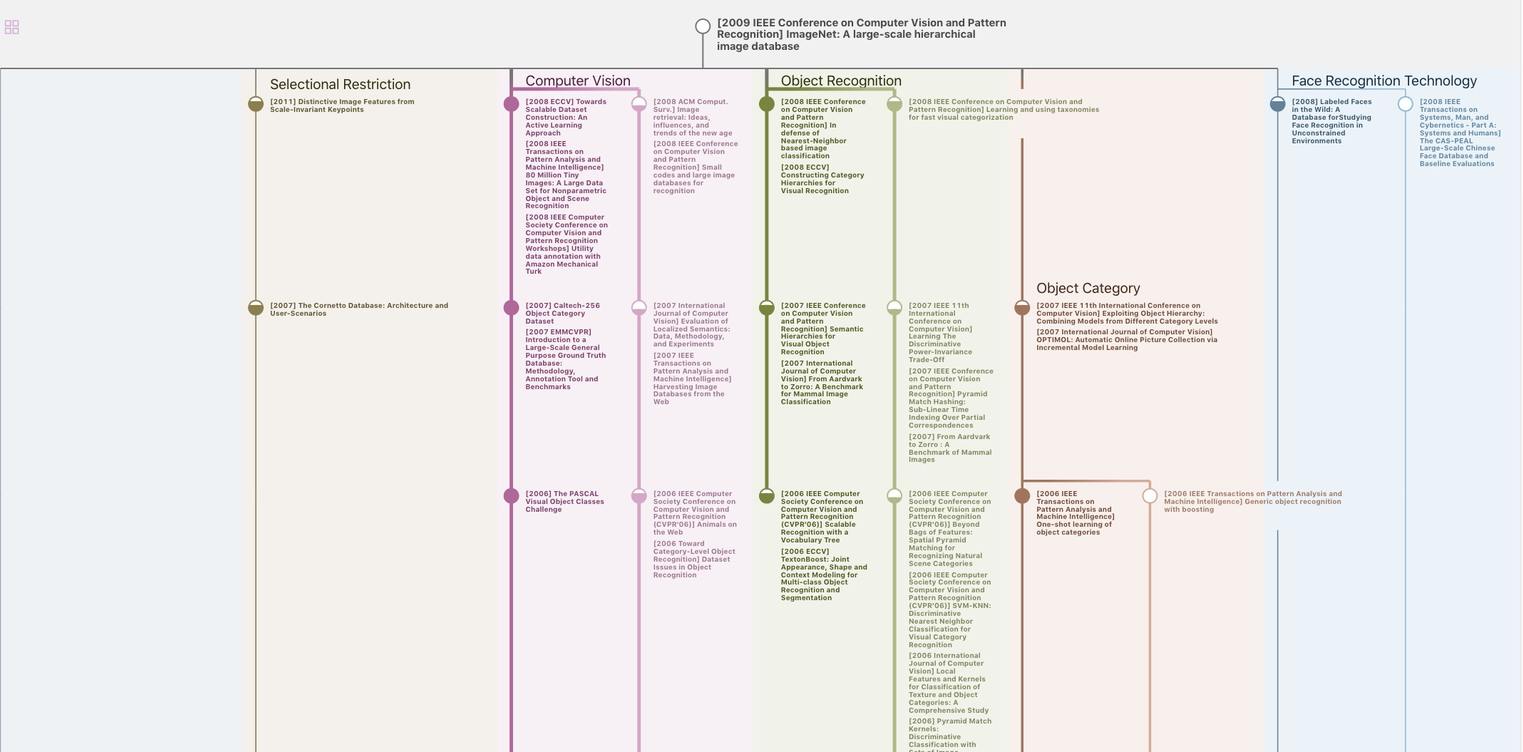
生成溯源树,研究论文发展脉络
Chat Paper
正在生成论文摘要