Multi-Agent Learning via Markov Potential Games in Marketplaces for Distributed Energy Resources.
CDC(2022)
摘要
Much change is happening in electricity markets due to the entrance of small-scale prosumers that both generate and consume electricity. Both large and small consumers can also be incentivized to reduce their demand during peak load periods, referred to as demand-response. The net effect of such distributed energy resources (DERs) on the grid can be quite substantial, and designing secondary markets wherein such DERs can participate repeatedly over time has become important. Many such marketplaces have a so-called potential game structure, in that a unilateral change in the strategy of an agent causes equivalent changes in both its own reward and a global potential function. We consider a dynamic setting in which each stage is a potential game, but is accompanied by Markovian state transitions, which we call Markov Potential Games (MPG). It is well known that it is formidably challenging to compute or learn Nash Equilibria (NE) in Markov Games. We develop a key concept that we term as the potential value function that ties together the potential function in the stage game with the value function in a Markov Decision Process. We first show that an NE can be computed in a centralized manner by maximizing the potential value function. We also show NE can also be obtained in a multi-agent manner via asynchronous better (not necessarily best) response updates that are consistent with a simple multi-agent reinforcement learning algorithm. Finally, we show several examples wherein the MPG framework applies to DER dynamics in an electricity marketplace, and numerically study the efficiency of the equilibria attained.
更多查看译文
关键词
demand-response,DER dynamics,distributed energy resources,dynamic setting,electricity marketplace,electricity markets,game structure,global potential function,Markov decision process,Markov potential games,Markovian state transitions,MPG,multiagent learning,multiagent reinforcement learning algorithm,Nash Equilibria,peak load periods,potential value function,secondary markets,small-scale prosumers
AI 理解论文
溯源树
样例
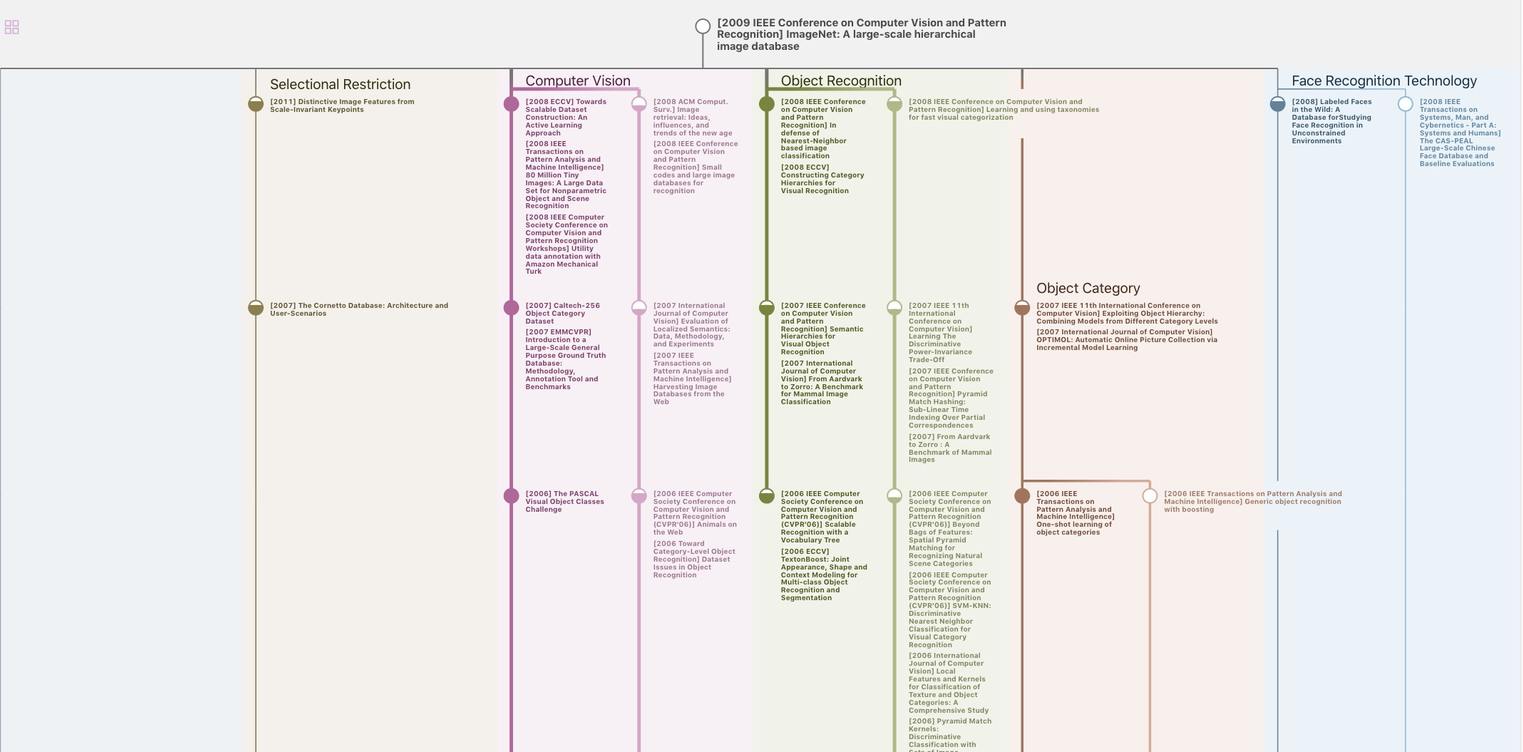
生成溯源树,研究论文发展脉络
Chat Paper
正在生成论文摘要