Geometrical Deep Learning for the Estimation of Residence Time in the Left Atria.
STACOM@MICCAI(2022)
摘要
Atrial fibrillation (AF) is the most common clinically significant arrhythmia, which can lead to clot formation in the left atrial appendage (LAA). Computational fluid dynamics simulations can provide advanced hemodynamic descriptors to help in the stratification of patient thrombogenic risk. For instance, the residence time measures the degree of fluid entrapment inside the domain of interest, which can be related to the risk of thrombus formation. However, classic fluid modeling approaches are notoriously time-consuming and resource intensive, preventing their use in large patient cohorts. Therefore, in this study, we leveraged deep learning (DL) methods to predict the residence time, solely based on the patient-specific left atrial geometry at inference time. To this end, we used graph neural networks since they are well-suited to non-structured data such as meshes, in which medical data and simulation results are often best represented. Two slightly different neural network architectures were trained on a cohort of 106 patients, with velocity fields derived from computational fluid dynamics simulations as training data. The average RT values were accurately predicted, with a mean normalized root mean squared error of 0.08, in less than 5 s, demonstrating the potential of DL-based surrogates of fluid simulation outcomes to save computational time and resources without compromising result accuracy.
更多查看译文
关键词
residence time,left atria,deep learning,estimation
AI 理解论文
溯源树
样例
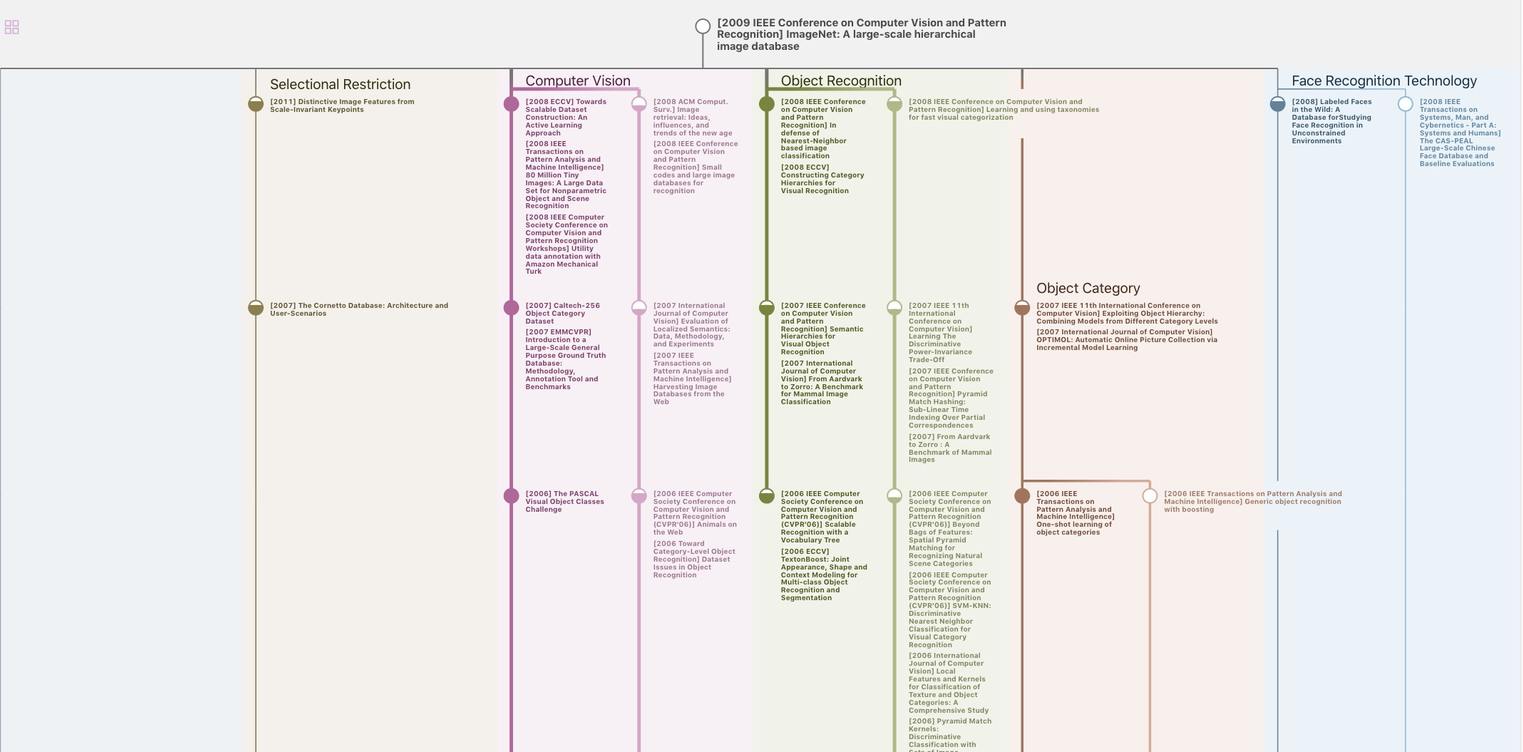
生成溯源树,研究论文发展脉络
Chat Paper
正在生成论文摘要