Towards Real-Time Optimization of Left Atrial Appendage Occlusion Device Placement Through Physics-Informed Neural Networks.
STACOM@MICCAI(2022)
摘要
The adoption of patient-specific computational fluid dynamics (CFD) simulations has been instrumental toward a better understanding of the mechanisms underlying thrombogenesis in the left atrial appendage. Such simulations can help optimize the placement of left atrial appendage occlusion (LAAO) devices in atrial fibrillation patients and avoid the generation of device-related thrombosis. However, integrating conventional solvers into clinical practice is cumbersome, as even the slightest change in model geometry involves computing the entire simulation from scratch. In contrast, neural networks can entirely circumvent this issue by transferring knowledge across models targeted at similar physical domains. Thus, in the present study, we introduced a neural network capable of predicting left atrial hemodynamics under different occlusion device configurations, relying solely on a single finite element simulation for training. To this end, we leveraged physics-informed neural networks (PINN), which embed the physical laws governing the domain of interest into the model, exhibiting far superior generalization capabilities than conventional data-driven models. Several device types and positions have been tested in two distinct left atrial geometries. By employing a single reference simulation per patient the network can predict the updated hemodynamics for a variety of device types and positions, orders of magnitude faster than with conventional CFD solvers.
更多查看译文
关键词
neural networks,occlusion,real-time,physics-informed
AI 理解论文
溯源树
样例
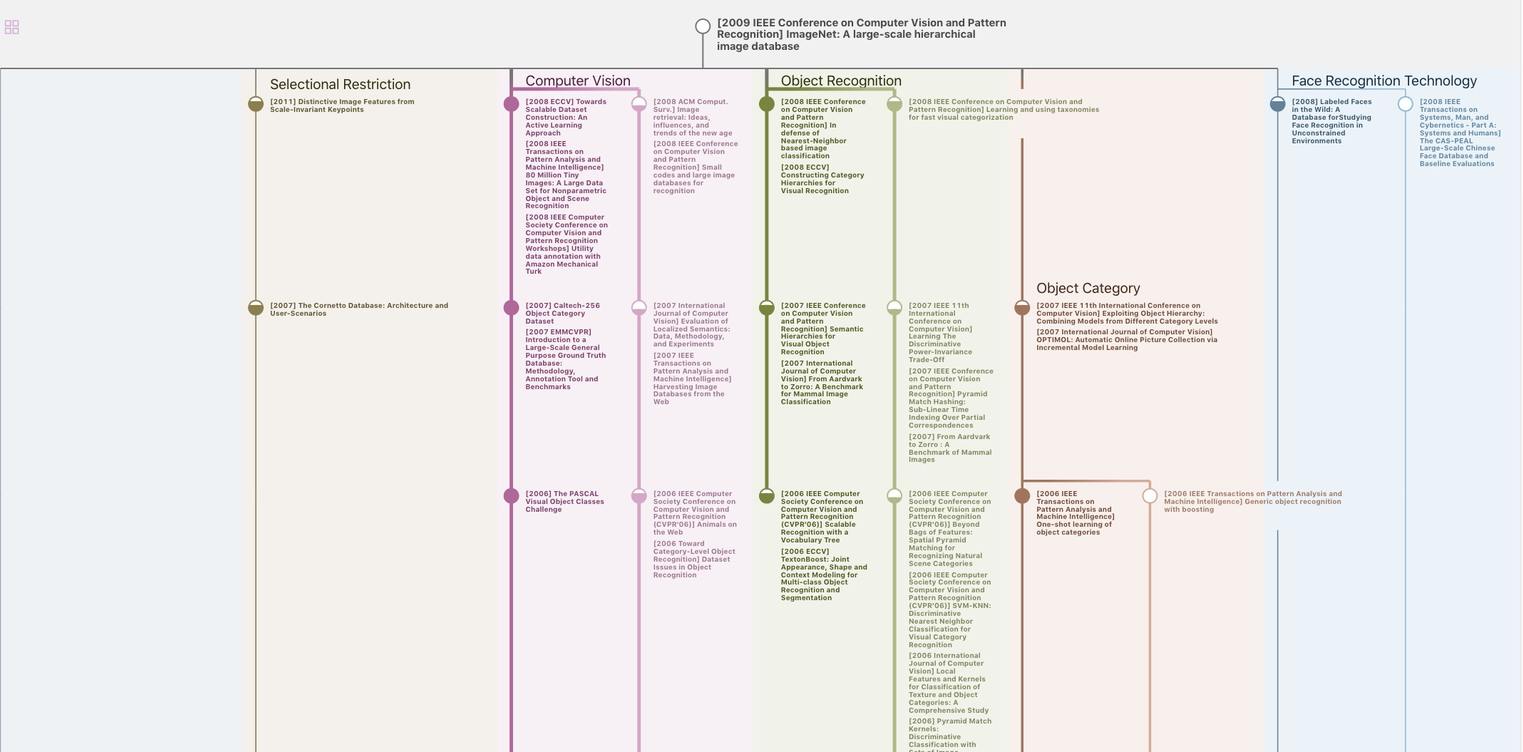
生成溯源树,研究论文发展脉络
Chat Paper
正在生成论文摘要