Explainable Pulmonary Disease Diagnosis with Prompt-Based Knowledge Extraction.
BIBM(2022)
摘要
Recent studies show that deep learning models perform well in many medical tasks such as medical imaging and automated diagnosis. With qualified training datasets, some models can achieve or even surpass expert-level performance on some tasks. However, as a typical black-box-style approach, deep learning lacks theoretical interpretability, which is especially important for medical tasks. On the other hand, there are many sources of domain knowledge for medical diagnosis from human experts, such as clinical guidelines. How to sufficiently integrate human knowledge in the model is crucial for explainable diagnosis. In this paper, we propose a novel framework for explainable automated diagnosis that leverages explicit medical knowledge. We automate the knowledge extraction from textual clinical guidelines with prompt-based learning, train a set of weighted first-order logical rules with constructed evidence database, and finally infer the diagnosis result with integrated knowledge and multi-sourced data. We instantiate the framework for pulmonary disease diagnosis, and our experiments on a real dataset show that our method outperforms the state-of-the-art baselines in accuracy and interpretability.
更多查看译文
关键词
knowledge extraction,explainable diagnosis
AI 理解论文
溯源树
样例
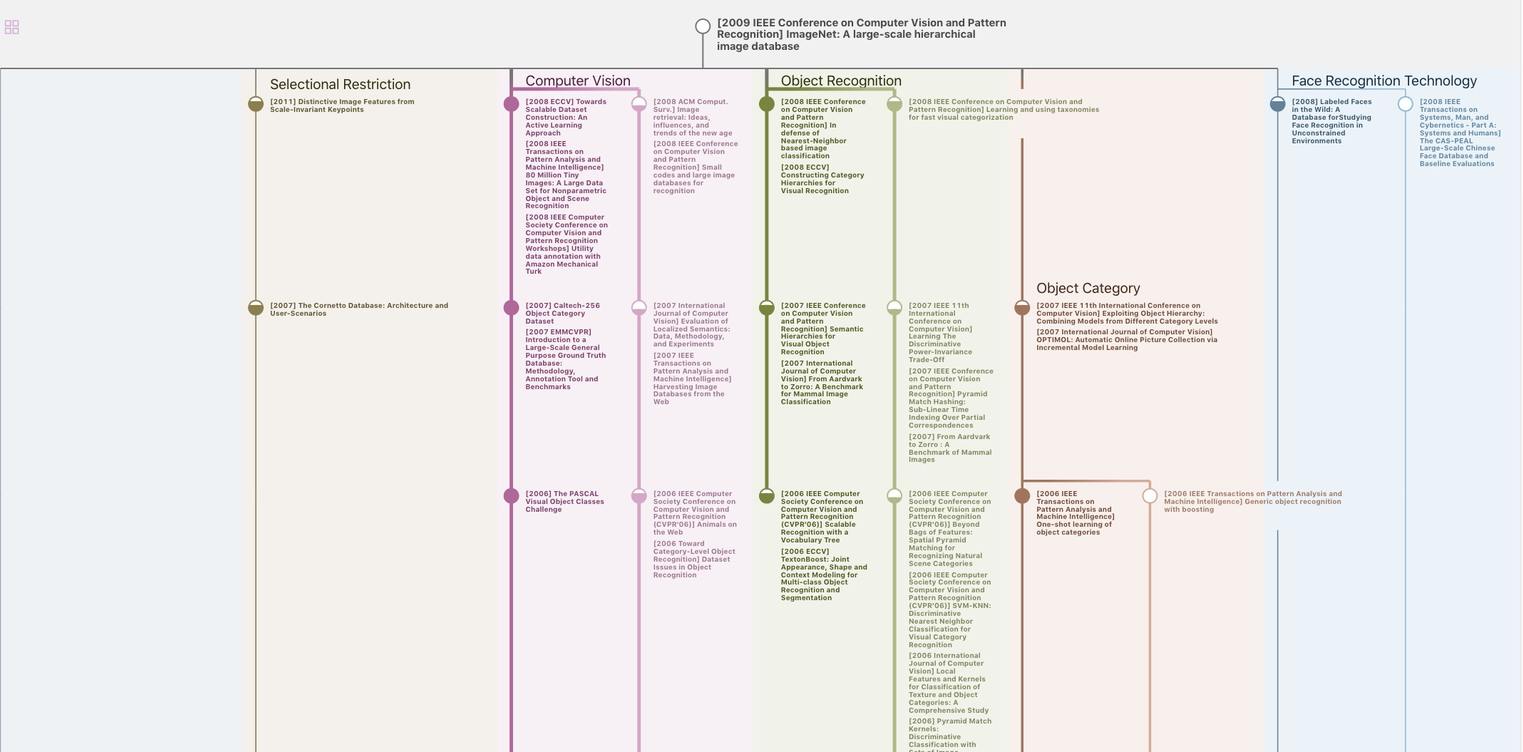
生成溯源树,研究论文发展脉络
Chat Paper
正在生成论文摘要