CFDA-M: Coarse-to-Fine Domain Adaptation for Mitochondria Segmentation via Patch-wise Image Alignment and Online Self-training.
BIBM(2022)
摘要
Accurate and robust segmentation of mitochondria from electron microscopy images plays a critical role in understanding cellular functions. While effective, learning-based approaches require vast quantities of expert annotations. Manual efforts can be alleviated by transferring the knowledge learned from the source domain to unseen target domains, as known as unsupervised domain adaptation. In this work, we propose a two-stage pipeline for cross-dataset mitochondria segmentation, aiming to mitigate domain shift in a coarse-to-fine manner. In the first stage, we integrate the style transfer block and segmentation network into an end-to-end image alignment framework. Specifically, patch-wise contrastive learning is employed to guarantee the semantic fidelity of mitochondria, providing more reliable translated images for the segmentation network. In the second stage, a novel online self-training network updated with target images and co-evolving pseudo labels is proposed to fine-tune the segmentation network trained beforehand. Furthermore, to effectively utilize unlabeled data, consistency regularization is introduced in both stages to enforce stable predictions under various perturbations. Experimental results on public datasets demonstrate that the proposed approach outperforms the existing methods by a large margin in bidirectional adaptation for mitochondria segmentation and achieves comparable results with supervised methods.
更多查看译文
关键词
mitochondria segmentation,adaptation,coarse-to-fine,patch-wise,self-training
AI 理解论文
溯源树
样例
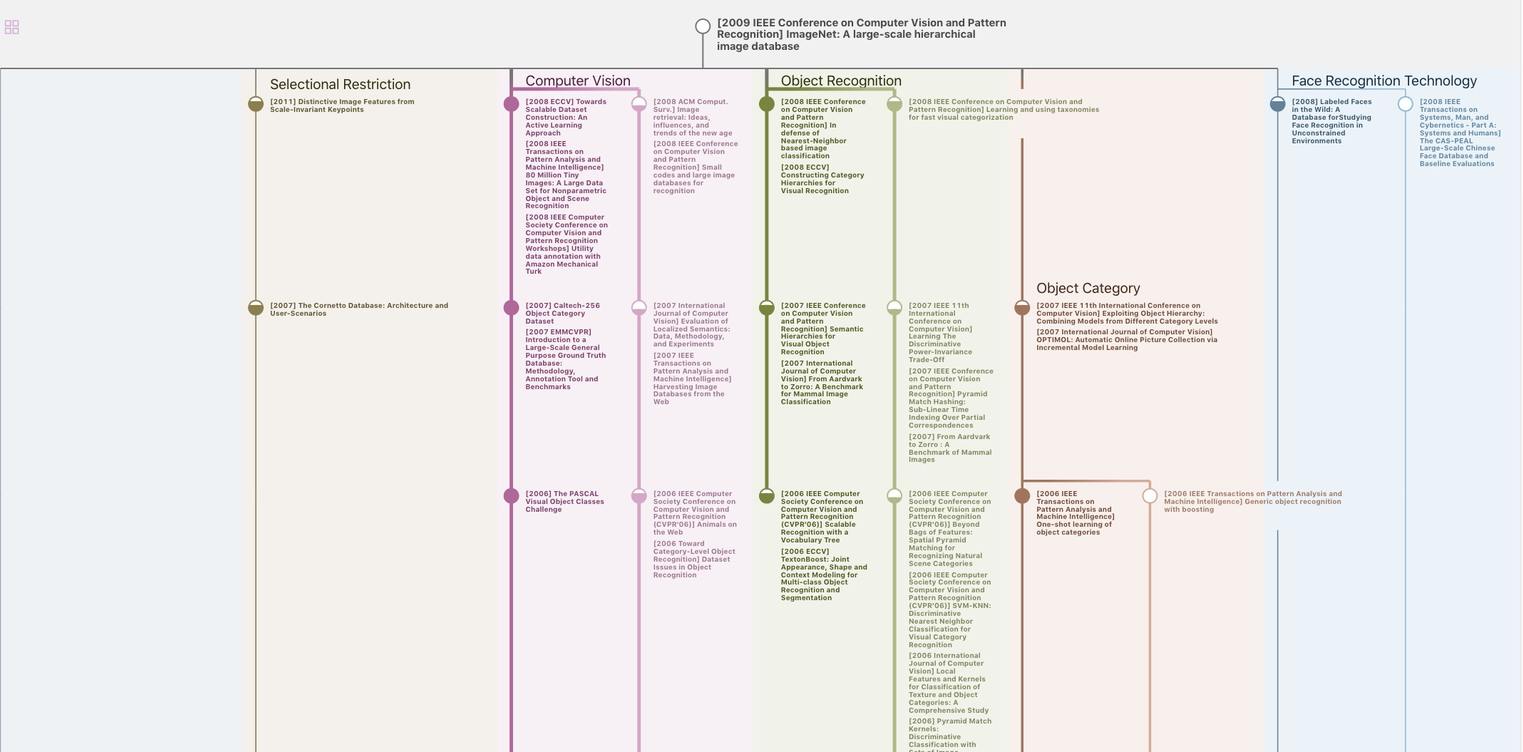
生成溯源树,研究论文发展脉络
Chat Paper
正在生成论文摘要