Defensive Adversarial Training for Enhancing Robustness of ECG based User Identification.
BIBM(2022)
摘要
Electrocardiogram (ECG) based user identification has received considerable attention with the advent of wearable devices. It provides emerging applications including personal healthcare a convenient way to authenticate users as the process can be performed at the moment the user makes contact with the device. However, a recent study discovered that injecting noise into the signal transmitted from the user to an application can effectively hinder the classification process. Many efforts have been made to deal with this noise injection attack, but most approaches have focused on noise removal. In contrast, this paper proposes Defensive Adversarial Training (DAT), which involves training a model with various noisy data to enhance the robustness of deep learning-based identification algorithms. We used two types of noise, Gaussian and Laplacian, to create noisy data. In addition, a sliding-window technique was used to effectively extract useful features and to achieve better accuracy. Our simulation results demonstrate that the proposed approach is highly robust to noise injection attacks and even against random noise. A comparative analysis with noise removal schemes also shows that the proposed DAT significantly enhances the robustness of ECG-based user identification.
更多查看译文
关键词
defensive adversarial training,ecg,robustness,identification
AI 理解论文
溯源树
样例
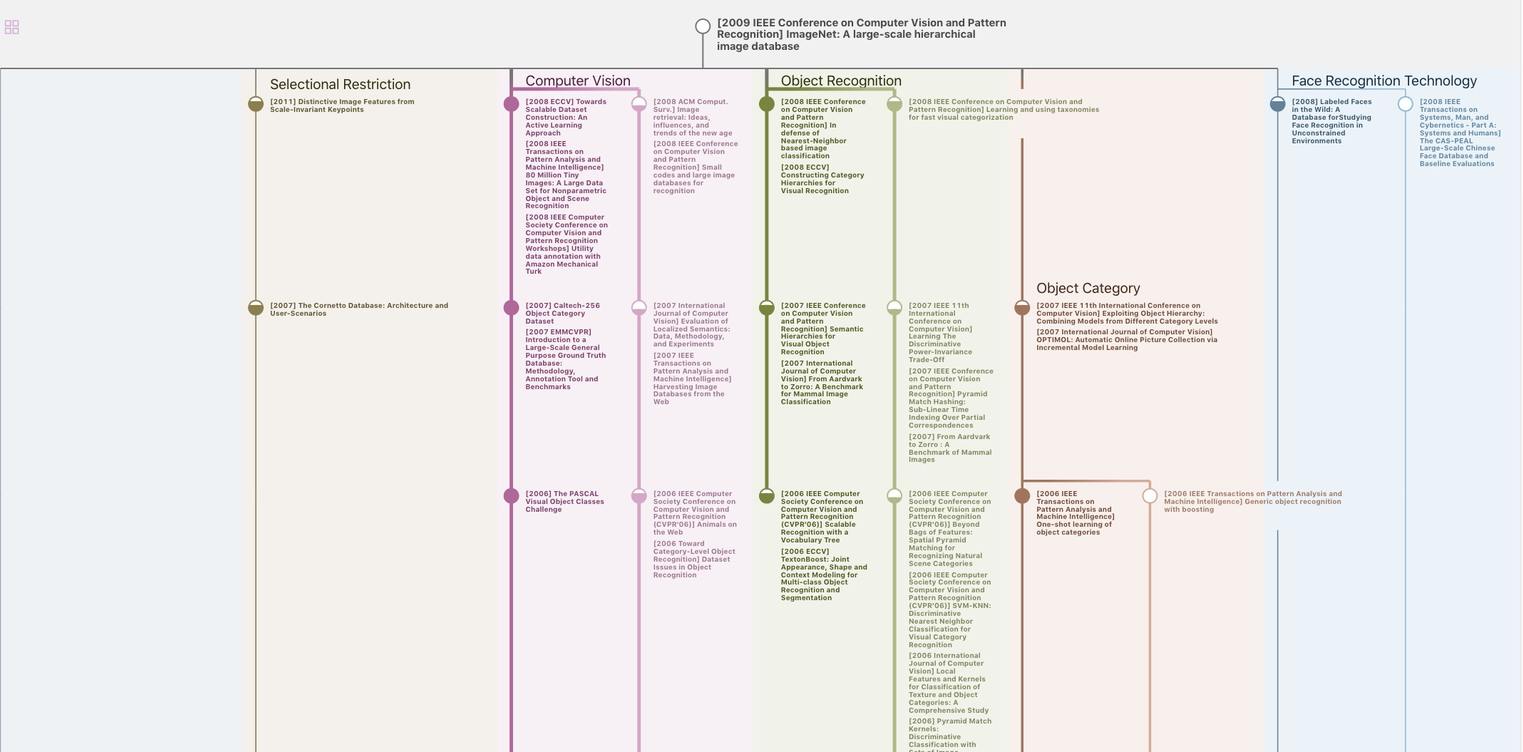
生成溯源树,研究论文发展脉络
Chat Paper
正在生成论文摘要