Retargeted Regression Methods for Multi-label Learning.
S+SSPR(2022)
摘要
In Multi-Label Classification, utilizing label relationship is a key to improve classification accuracy. Label Space Dimension Reduction or Classifier Chains utilizes the relationship explicitly however those utilization are still limited. In this paper, we propose Retargeted Regression methods for Multi-Label classification by extending Retargeted Linear Least Squares originally proposed for Multi-Class Classification. Retargeted methods not only learn classifiers but also modify targets with margin constraints. Since in Multi-Label Classification, an instance may have more than one label, large margin constraints between all pairs of positive labels and negative labels are introduced. This enables to utilize the label relationship with taking ranking of labels for each instance into consideration. We also propose a simple heuristic to determine a threshold parameter for each instance to earn zero-one classification. On nine benchmark datasets, the proposed method outperformed conventional methods in the sense of instance-wise ranking. In best cases, classification accuracy was improved at 7% on AUC metric.
更多查看译文
关键词
regression methods,learning,multi-label
AI 理解论文
溯源树
样例
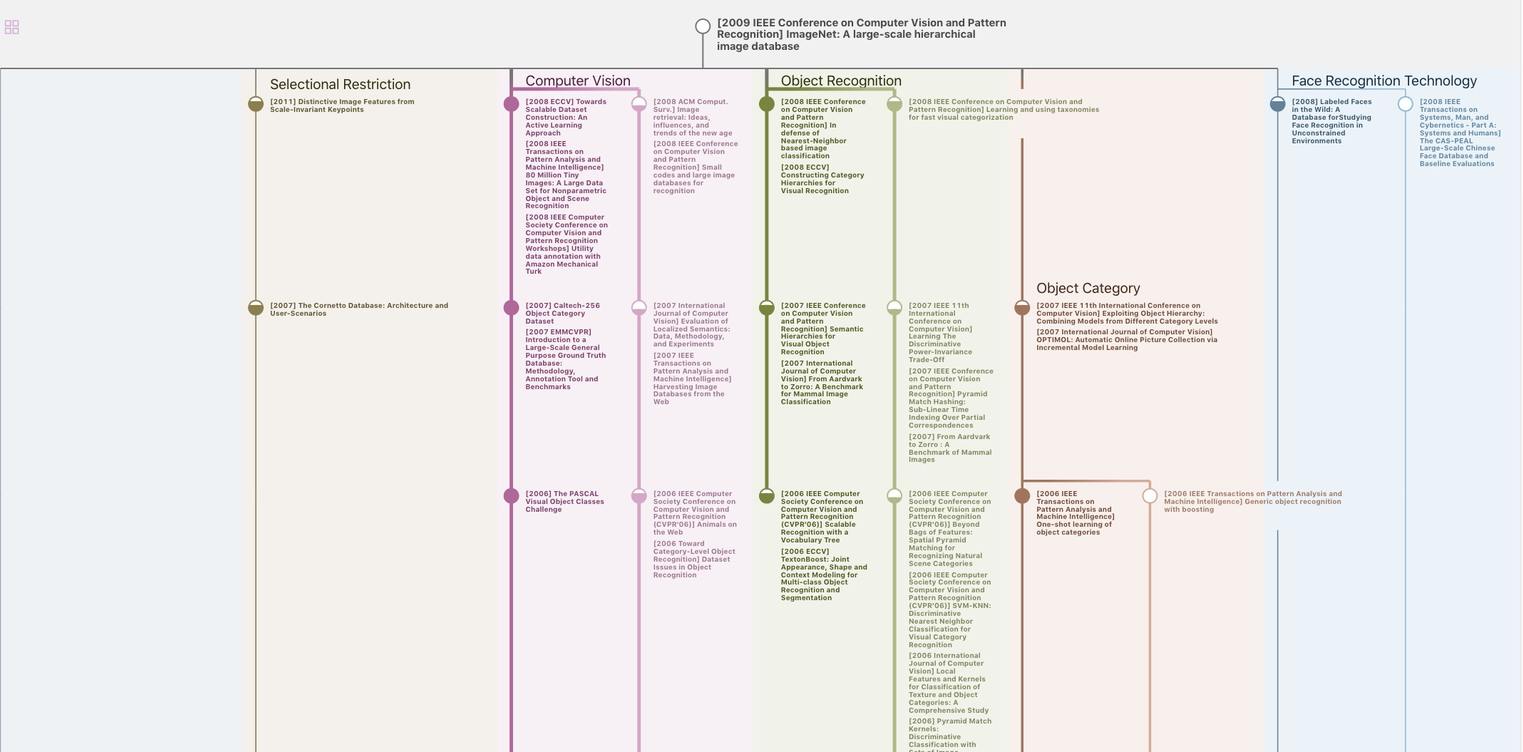
生成溯源树,研究论文发展脉络
Chat Paper
正在生成论文摘要