Learning to Jointly Segment the Liver, Lesions and Vessels from Partially Annotated Datasets.
ICIP(2022)
摘要
The segmentation of the liver, lesions, and vessels from pre-operative CT scans is of major importance in hepatic surgery planning. However, large databases with reference segmentations for these regions of interest remain unavailable, a challenge often encountered in medical image segmentation. In this work, we propose the FuSe loss, a novel loss function for multi-task learning on datasets with partial annotations. By employing the nnU-Net's 3D self-configuring pipeline to calibrate and train a deep network for the joint segmentation of the liver, lesions, and vessels, we show how the FuSe loss allows to learn from the differently annotated IRCAD and LiTS datasets, improving the overall baseline segmentation performance. With the FuSe loss, the dice scores reached up to 95.9%, 70.6% and 60.0% for the liver, lesions, and vessels respectively.
更多查看译文
关键词
Semantic segmentation, multi-task learning, partially labeled data, weighted loss function
AI 理解论文
溯源树
样例
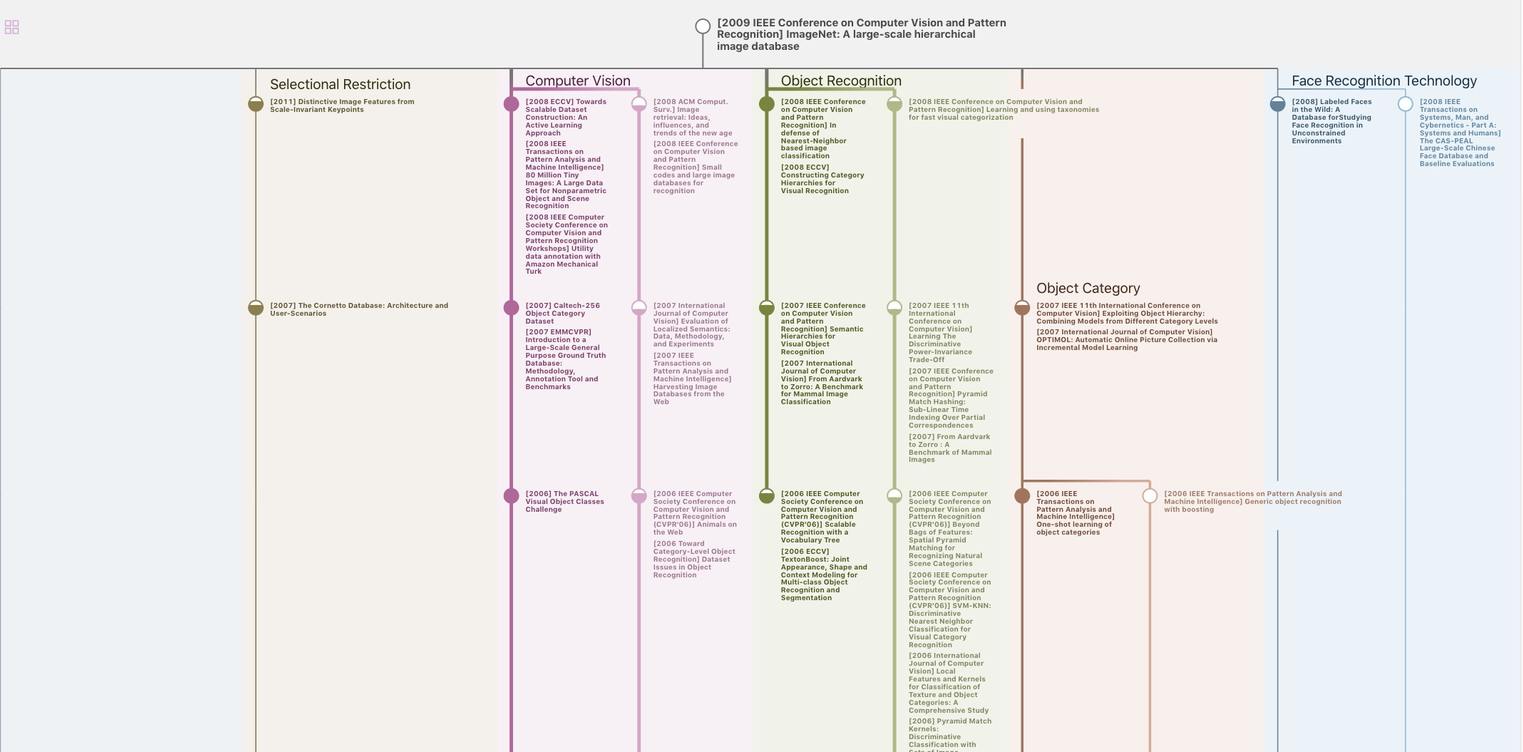
生成溯源树,研究论文发展脉络
Chat Paper
正在生成论文摘要