Transformer Based Self-Context Aware Prediction for Few-Shot Anomaly Detection in Videos.
ICIP(2022)
摘要
Anomaly detection in videos is a challenging task as anomalies in different videos are of different kinds. Therefore, a promising way to approach video anomaly detection is by learning the non-anomalous nature of the video at hand. To this end, we propose a one-class few-shot learning driven transformer based approach for anomaly detection in videos that is self-context aware. Features from the first few consecutive non-anomalous frames in a video are used to train the transformer in predicting the non-anomalous feature of the subsequent frame. This takes place under the attention of a self-context learned from the input features themselves. After the learning, given a few previous frames, the video-specific transformer is used to infer if a frame is anomalous or not by comparing the feature predicted by it with the actual. The effectiveness of the proposed method with respect to the state-of-the-art is demonstrated through qualitative and quantitative results on different standard datasets. We also study the positive effect of the self-context used in our approach.
更多查看译文
关键词
Anomaly detection, feature prediction, transformer network, self-context
AI 理解论文
溯源树
样例
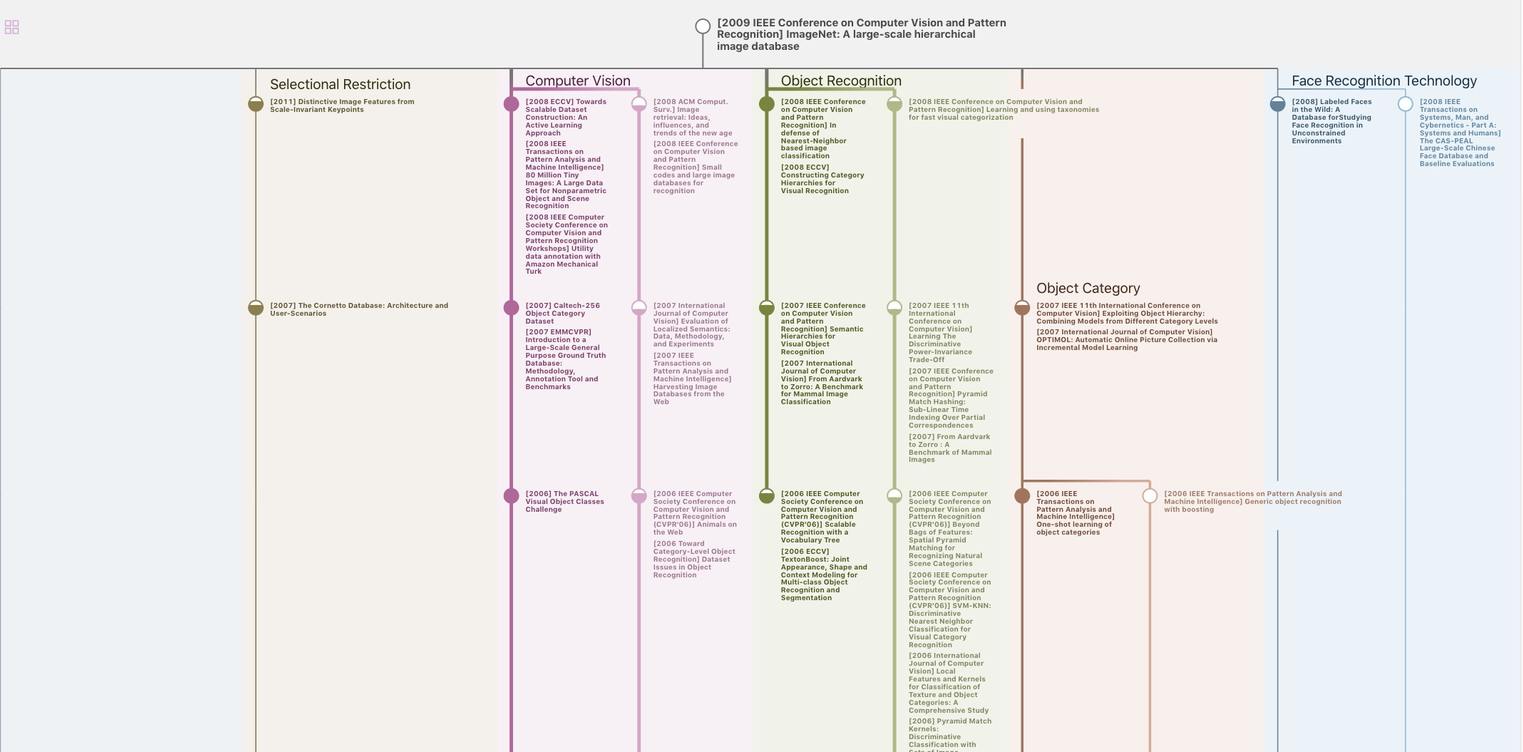
生成溯源树,研究论文发展脉络
Chat Paper
正在生成论文摘要