Fine-Grained Head Pose Estimation Based on a 6D Rotation Representation with Multiregression Loss.
CollaborateCom (2)(2022)
摘要
Estimating the head pose is vital in action evaluation since it has extensive applications such as in automobile driver-assistance systems, performance evaluations of athletes and customers' attention in retail stores. It is difficult to predict the head orientation from an RGB image by deep learning more accurately. We propose 6DHPENet, a fine-grained 6D head pose estimation network, to estimate the 3D rotations of the head. First, the model adopts a 6D rotation representation for 3D rotations as training objective to guarantee effective learning. 6D rotation representation is a continuous and one-to-one mapping function for 3D rotations. Second, achieving 3D facial landmarks from real-time activities consumes more time and is subject to frontal views. We drop the 3D facial landmarks to enhance the adaptability and generalization ability in various application scenes. Third, after the last convolution extraction layer, a squeeze-and-excitation module is introduced to construct both the local spatial and global channel-wise facial feature information by explicitly modeling the interdependencies between the feature channels. Finally, a multiregression loss function is presented to improve the accuracy and stability for a full-range viewof the head pose estimation. In addition, our method is compact and efficient for mobile devices because of the lightweightCNN backbone. The quantitative experiment results trained on 300W-LP datasets show the superior performance of our 6D rotation representation-based multiregression fine-grained method on the AFLW2000 and BIWI datasets.
更多查看译文
关键词
Head pose estimation, 6D rotation, Fine-grained image analysis, Multiregression loss, Landmark-free method
AI 理解论文
溯源树
样例
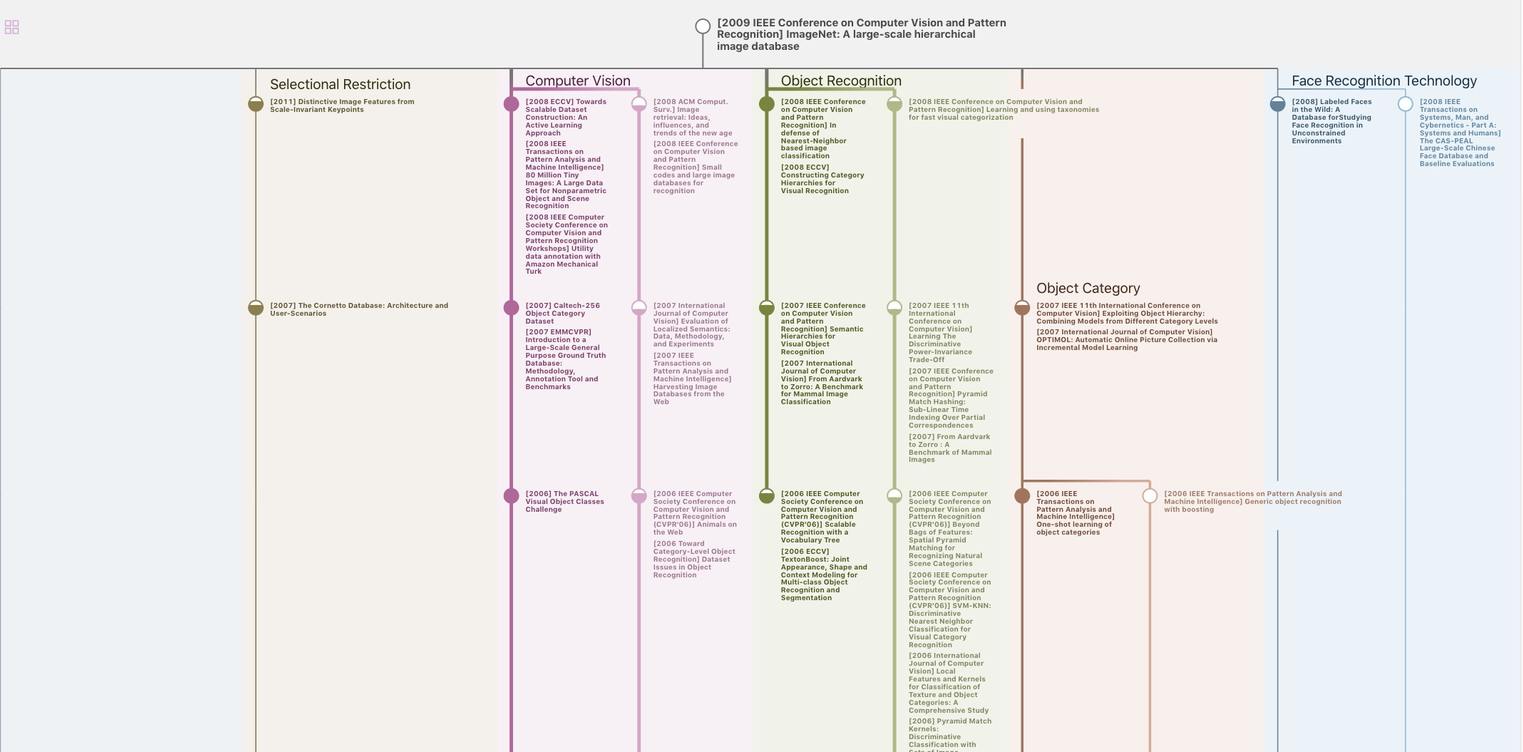
生成溯源树,研究论文发展脉络
Chat Paper
正在生成论文摘要