Variational Depth Networks: Uncertainty-Aware Monocular Self-supervised Depth Estimation.
ECCV Workshops (8)(2022)
摘要
Using self-supervised learning, neural networks are trained to predict depth from a single image without requiring ground-truth annotations. However, they are susceptible to input ambiguities and it is therefore important to express the corresponding depth uncertainty. While there are a few truly monocular and self-supervised methods modelling uncertainty, none correlates well with errors in depth. To this end we present Variational Depth Networks (VDN): a probabilistic extension of the established monocular depth estimation framework, MonoDepth2, in which we leverage variational inference to learn a parametric, continuous distribution over depth, whose variance is interpreted as uncertainty. The utility of the obtained uncertainty is then assessed quantitatively in a 3D reconstruction task, using the ScanNet dataset, showing that the accuracy of the reconstructed 3D meshes highly correlates with the precision of the predicted distribution. Finally, we benchmark our results using 2D depth evaluation metrics on the KITTI dataset.
更多查看译文
关键词
variational depth networks,uncertainty-aware,self-supervised
AI 理解论文
溯源树
样例
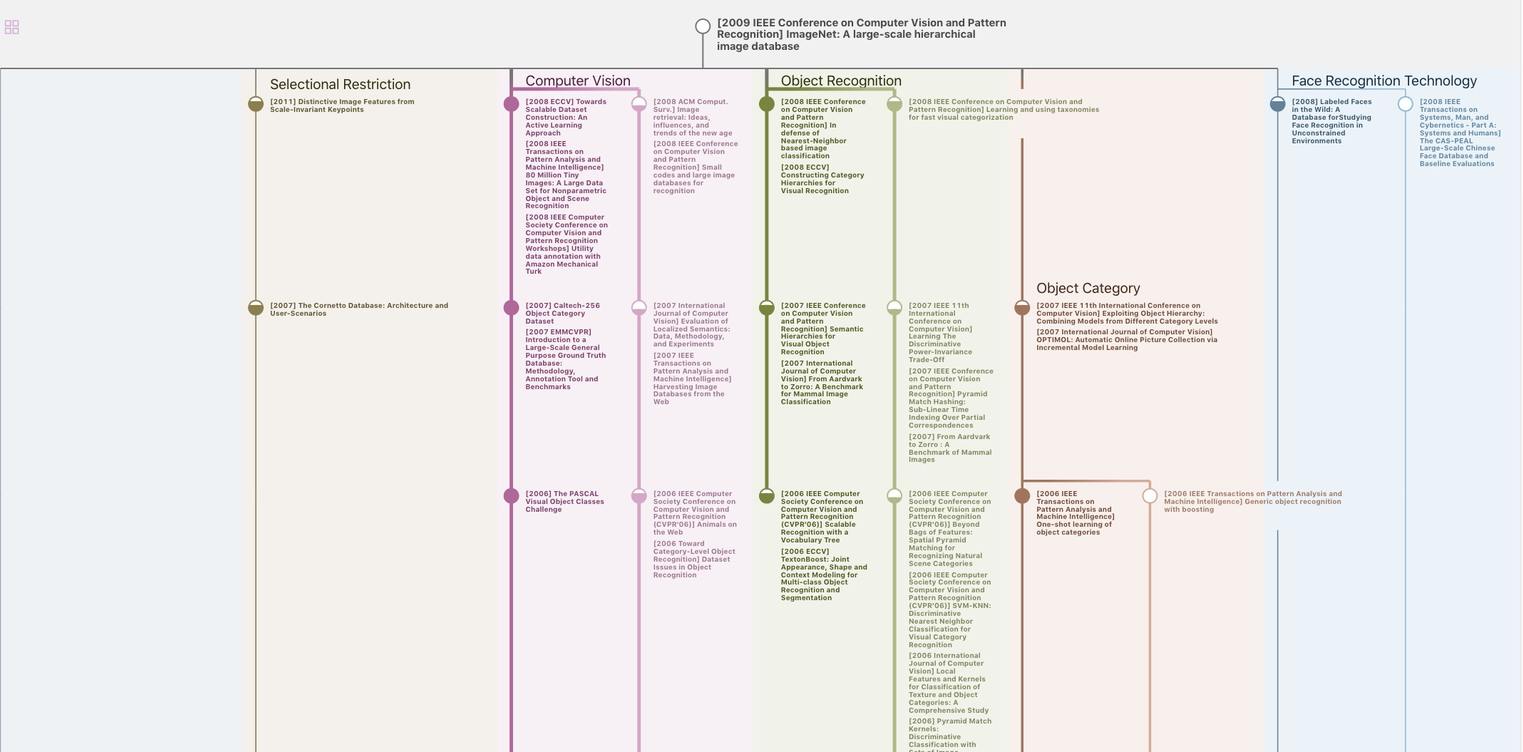
生成溯源树,研究论文发展脉络
Chat Paper
正在生成论文摘要