Which Expert Knows Best? Modulating Soft Learning with Online Batch Confidence for Domain Adaptive Person Re-Identification.
ECCV Workshops (5)(2022)
摘要
Deploying a person re-identification (Re-ID) system in a real scenario requires adapting a model trained on one labeled dataset to a different environment, with no person identity information. This poses an evident challenge that can be faced by unsupervised domain adaptation approaches. Recent state-of-the-art methods adopt architectures composed of multiple models (a.k.a. experts), and transfer the learned knowledge from the source domain by clustering and assigning hard pseudo-labels to unlabeled target data. While this approach achieves outstanding accuracy, the clustering procedure is typically sub-optimal, and the experts are simply combined to learn in a collaborative way, thus limiting the final performance. In order to mitigate the effects of noisy pseudo-labels and better exploit experts’ knowledge, we propose to combine soft supervision techniques in a novel multi-expert domain adaptation framework. We introduce a novel weighting mechanism for soft supervisory learning, named Online Batch Confidence, which takes into account expert reliability in an online per-batch basis. We conduct experiments across popular cross-domain Re-ID benchmarks proving that our model outperforms the current state-of-the-art results.
更多查看译文
关键词
Human re-identification, Unsupervised domain adaptation, Clustering, Soft learning, Mutual mean teaching
AI 理解论文
溯源树
样例
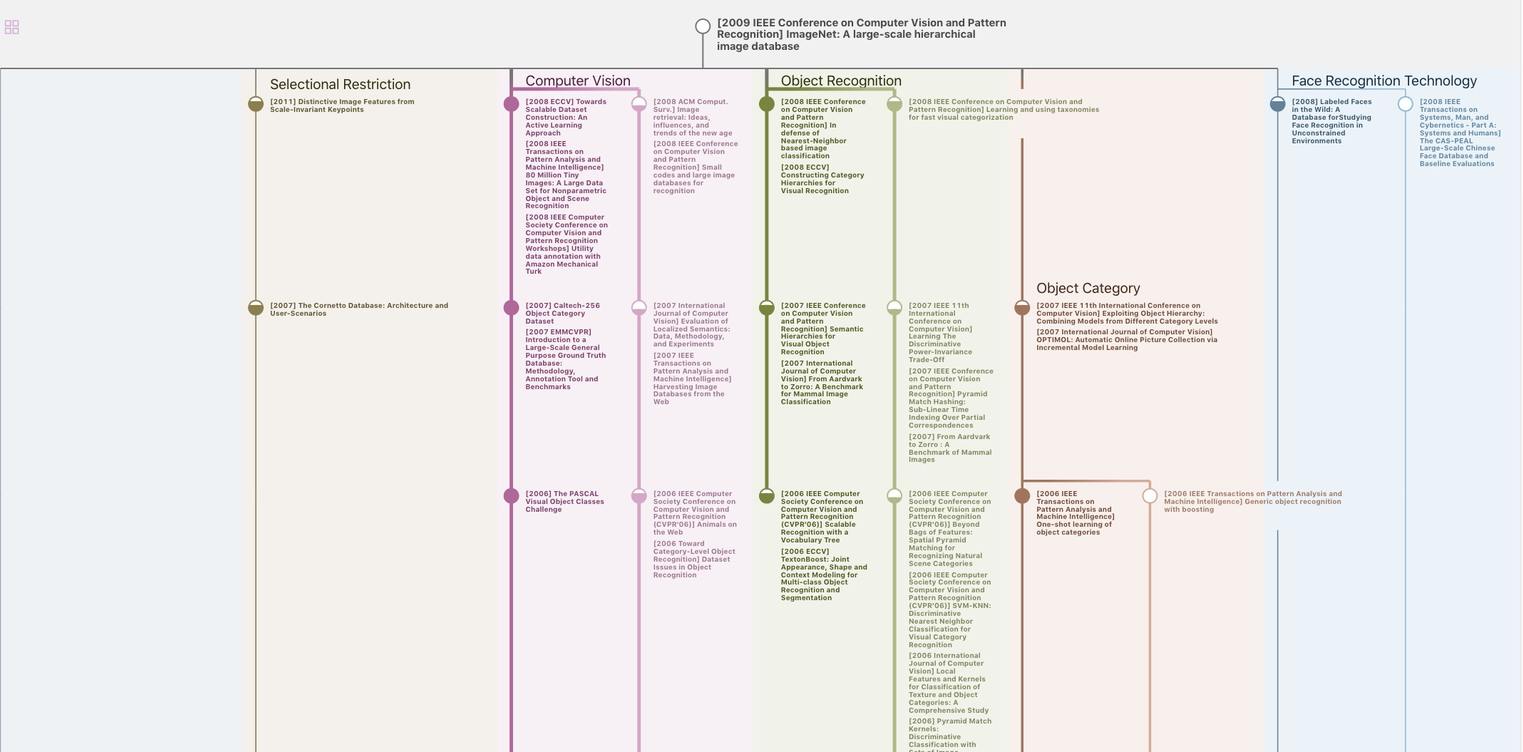
生成溯源树,研究论文发展脉络
Chat Paper
正在生成论文摘要