Beyond Local Processing: Adapting CNNs for CT Reconstruction.
ECCV Workshops (3)(2022)
摘要
Convolutional neural networks (CNNs) are well suited for image restoration tasks, like super resolution, deblurring, and denoising, in which the information required for restoring each pixel is mostly concentrated in a small neighborhood around it in the degraded image. However, they are less natural for highly non-local reconstruction problems, such as computed tomography (CT). To date, this incompatibility has been partially circumvented by using CNNs with very large receptive fields. Here, we propose an alternative approach, which relies on the rearrangement of the CT projection measurements along the CNN’s 3rd (channels’) dimension. This leads to a more local inverse problem, which is suitable for CNNs. We demonstrate our approach on sparse-view and limited-view CT, and show that it significantly improves reconstruction accuracy for any given network model. This allows achieving the same level of accuracy with significantly smaller models, and thus induces shorter training and inference times.
更多查看译文
关键词
cnns,ct,local processing,reconstruction
AI 理解论文
溯源树
样例
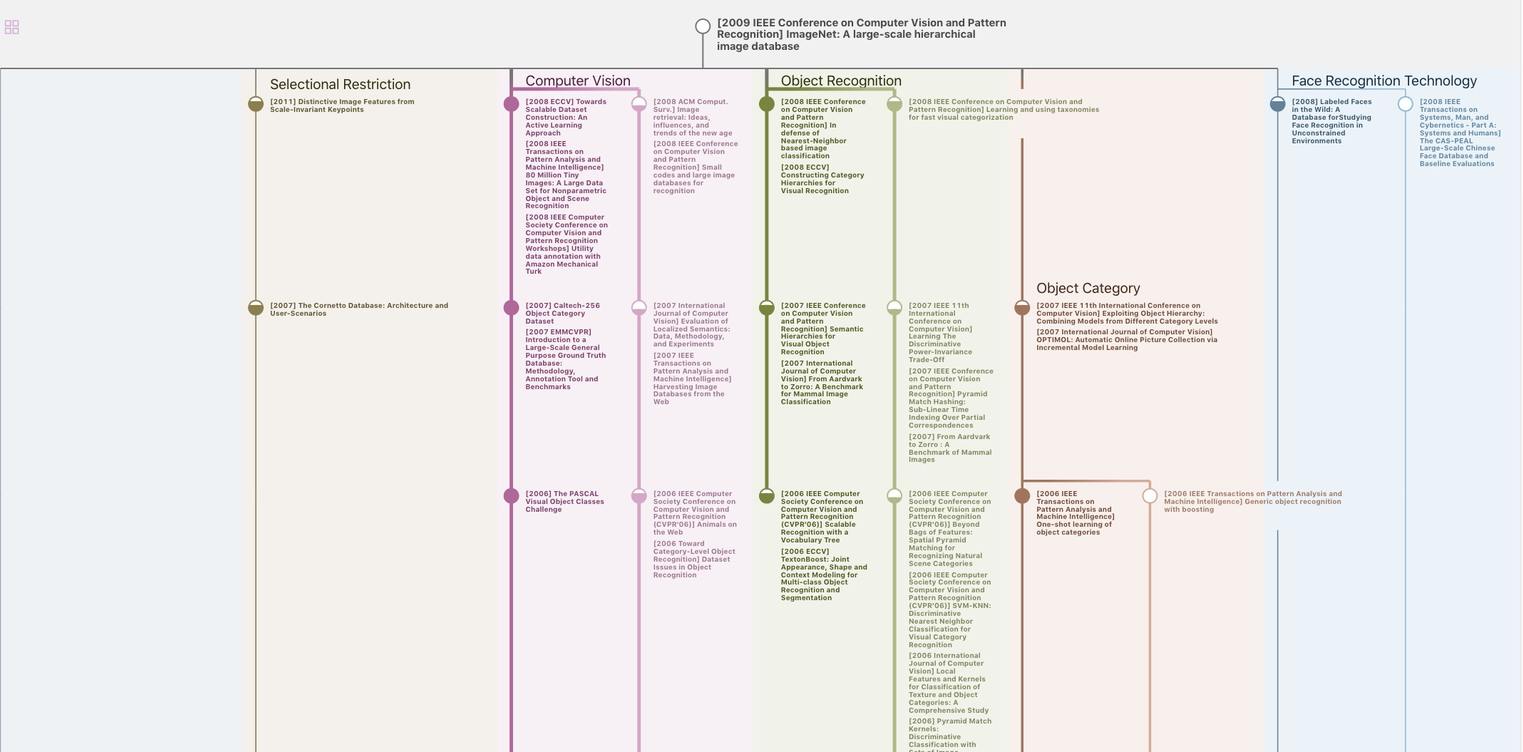
生成溯源树,研究论文发展脉络
Chat Paper
正在生成论文摘要