ChaLearn LAP Seasons in Drift Challenge: Dataset, Design and Results.
ECCV Workshops (5)(2022)
摘要
In thermal video security monitoring the reliability of deployed systems rely on having varied training data that can effectively generalize and have consistent performance in the deployed context. However, for security monitoring of an outdoor environment the amount of variation introduced to the imaging system would require extensive annotated data to fully cover for training and evaluation. To this end we designed and ran a challenge to stimulate research towards alleviating the impact of concept drift on object detection performance. We used an extension of the Long-Term Thermal Imaging Dataset, composed of thermal data acquired from 14th May 2020 to 30th of April 2021, with a total of 1689 2-min clips with bounding-box annotations for 4 different categories. The data covers a wide range of different weather conditions and object densities with the goal of measuring the thermal drift over time, from the coldest day/week/month of the dataset. The challenge attracted 184 registered participants, which was considered a success from the perspective of the organizers. While participants managed to achieve higher mAP when compared to a baseline, concept drift remains a strongly impactful factor. This work describes the challenge design, the adopted dataset and obtained results, as well as discuss top-winning solutions and future directions on the topic.
更多查看译文
关键词
drift challenge,dataset
AI 理解论文
溯源树
样例
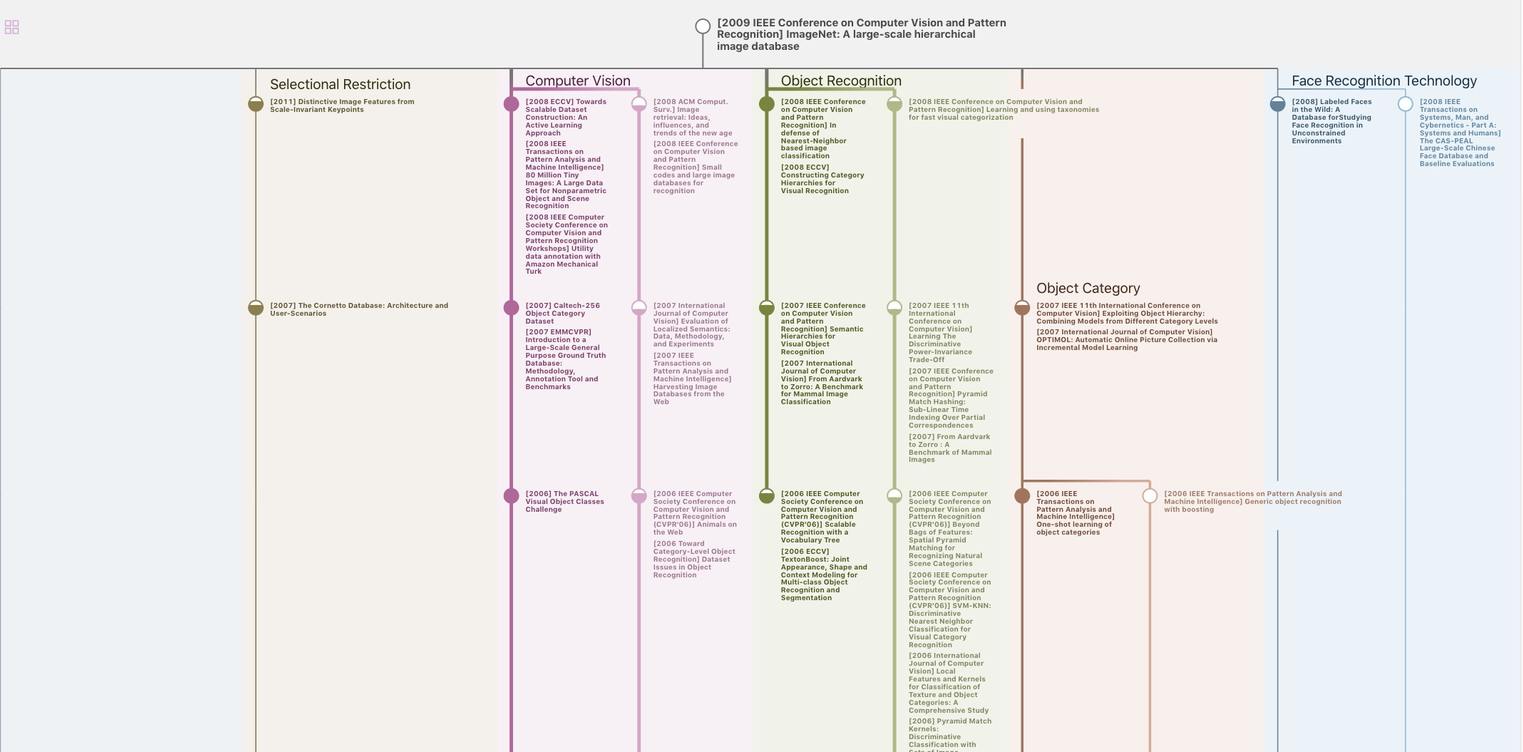
生成溯源树,研究论文发展脉络
Chat Paper
正在生成论文摘要