Multi-Agent DRL-Based Computation Offloading in Multiple RIS-Aided IoV Networks
MILCOM(2022)
摘要
This paper considers an internet of vehicles (IoV) network consisting of vehicle-to-vehicle (V2V) and vehicle-to-infrastructure (V2I) architecture aided by multi-access edge computing (MEC) servers deployed at base stations (BSs). The V2I communication is also assisted by multiple reconfigurable intelligent surfaces (RISs) for both uplink and downlink transmission. An intelligent task offloading methodology is designed to optimize the resource allocation scheme in the vehicular network which is based on the condition of the network and the priority and size of tasks. Furthermore, we also propose a multi-agent deep reinforcement learning (MA-DRL) algorithm for optimizing task offloading decision strategy and then compare its performance with benchmark DRL algorithms such as soft actor-critic (SAC), deep deterministic policy gradient (DDPG), twin delayed DDPG (TD3). Additionally, we compare the performance of the vehicle-to-BS or V2I offloading system with and without the presence of RIS in the proposed framework. Extensive numerical results are performed that demonstrate that the proposed MA-DRL-based RIS-assisted IoV network achieves higher utility, while improving the offloading rate of the tasks as well as ensuring that a higher percentage of offloaded tasks are completed compared to that of other DRL based and non-RIS assisted IoV frameworks.
更多查看译文
关键词
computation offloading,multi-agent,drl-based,ris-aided
AI 理解论文
溯源树
样例
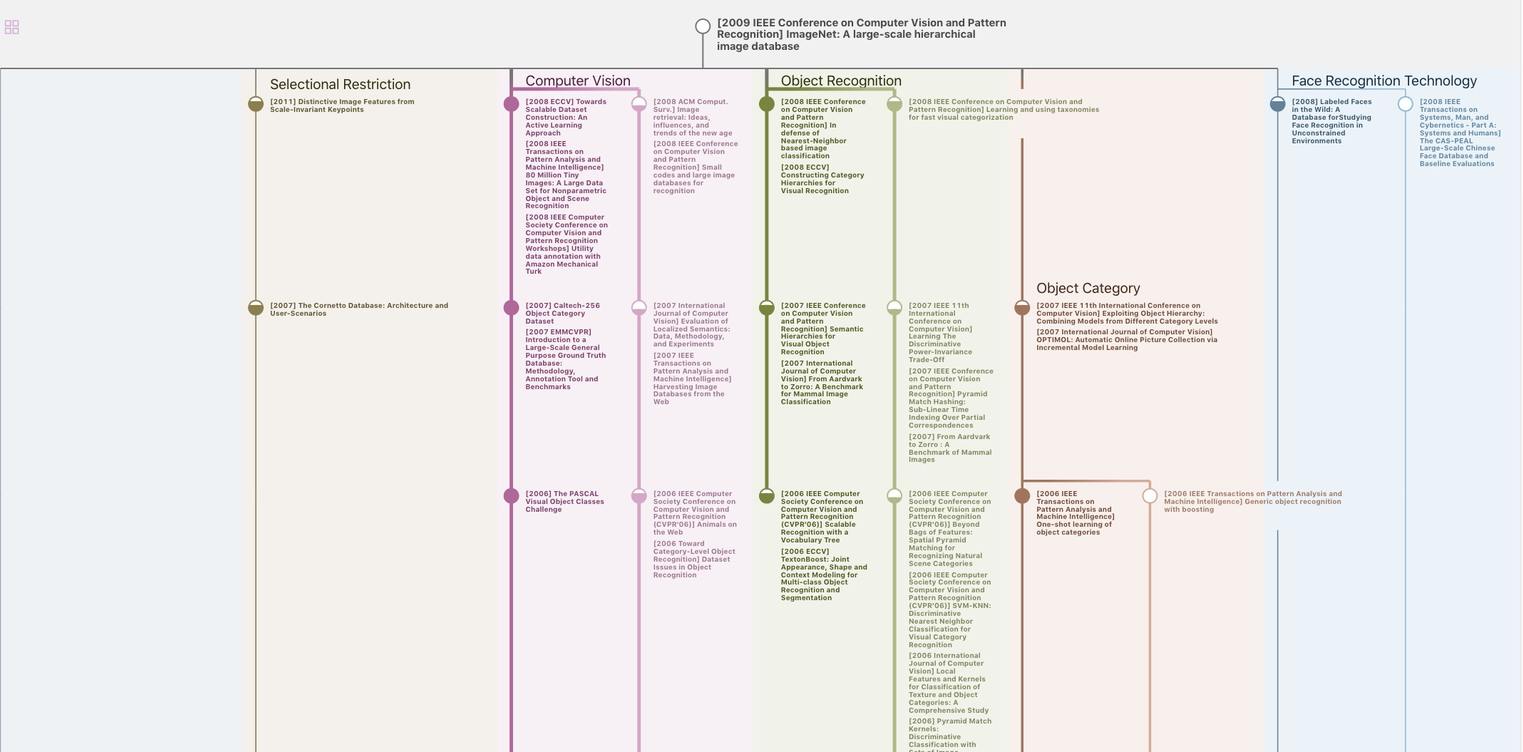
生成溯源树,研究论文发展脉络
Chat Paper
正在生成论文摘要