Learning visual question answering on controlled semantic noisy labels
Pattern Recognition(2023)
摘要
•To the best of our knowledge, we are the first to explore controlled semantic noisy labels for visual question answering task. We construct challenging benchmark datasets: VQA-Noise v1 and VQA-Noise v2 to imitate the human mislabeling behavior based on the analysis of existing datasets.•We evaluate the performance degradation of several popular VQA models on new benchmark datasets. To mitigate this issue, we propose a simple yet effective solution, namely Semantic Noisy Label Correction (SNLC), to enhance the robustness of VQA under noisy labels.•Extensive experiments are conducted on new benchmark datasets and demonstrate that the proposed SNLC improves the performance of all tested VQA models cross all noise rates.
更多查看译文
关键词
Visual question answering,Noisy datasets,Semantic labels,Contrastive learning
AI 理解论文
溯源树
样例
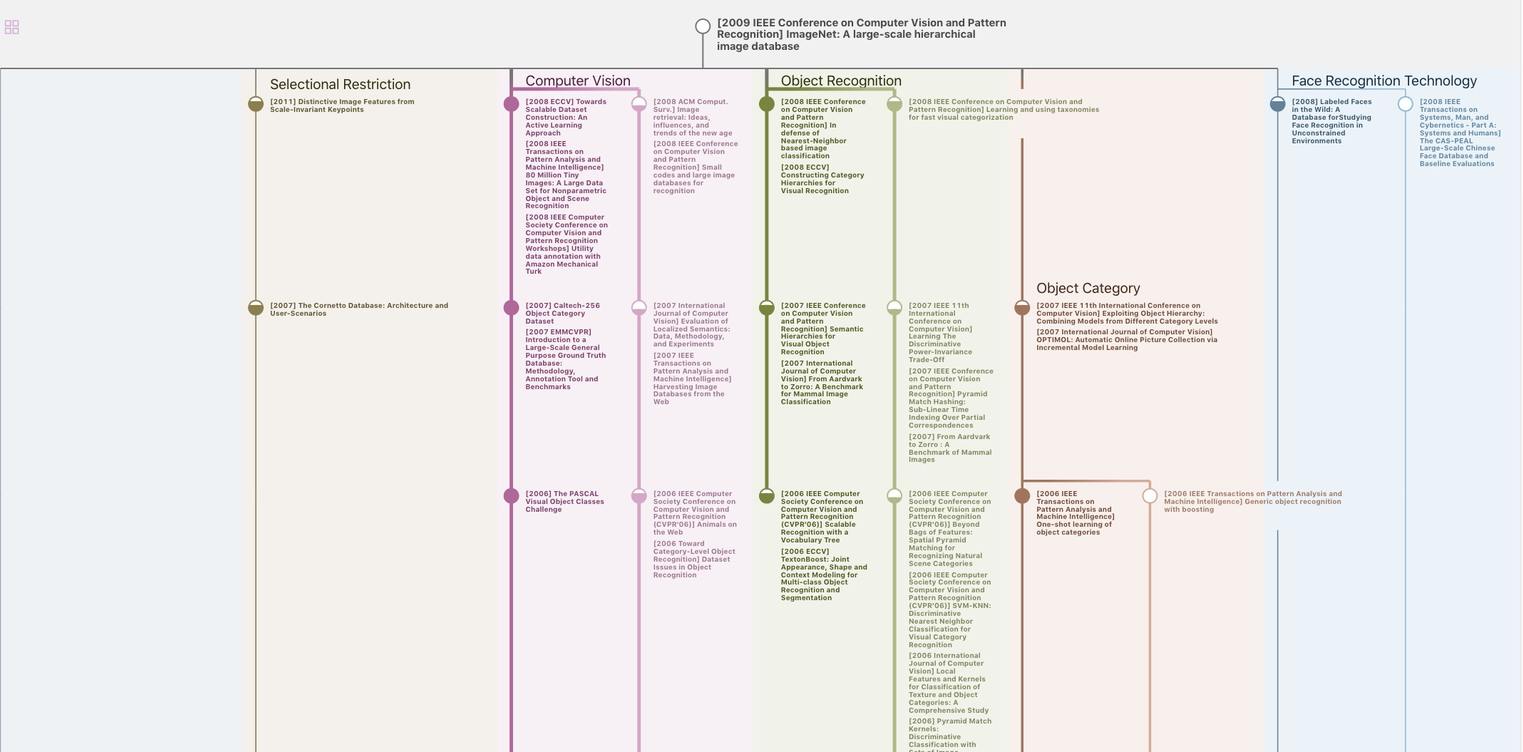
生成溯源树,研究论文发展脉络
Chat Paper
正在生成论文摘要