Empirical comparison and recent advances of computational prediction of hormone binding proteins using machine learning methods
Computational and Structural Biotechnology Journal(2023)
摘要
Hormone binding proteins (HBPs) belong to the group of soluble carrier proteins. These proteins selectively and non-covalently interact with hormones and promote growth hormone signaling in human and other animals. The HBPs are useful in many medical and commercial fields. Thus, the identification of HBPs is very important because it can help to discover more details about hormone binding proteins. Meanwhile, the experimental methods are time-consuming and expensive for hormone binding proteins recognition. Computational prediction methods have played significant roles in the correct recognition of hormone binding proteins with the use of sequence information and ML algorithms. In this review, we compared and assessed the implementation of ML-based tools in recognition of HBPs in a unique way. We hope that this study will give enough awareness and knowledge for research on HBPs. (c) 2023 The Authors. Published by Elsevier B.V. on behalf of Research Network of Computational and Structural Biotechnology. This is an open access article under the CC BY-NC-ND license (http://creativecommons.org/licenses/by-nc-nd/4.0/).
更多查看译文
关键词
Hormone binding proteins,Feature extraction,Feature selection,Machine learning,Computational tools
AI 理解论文
溯源树
样例
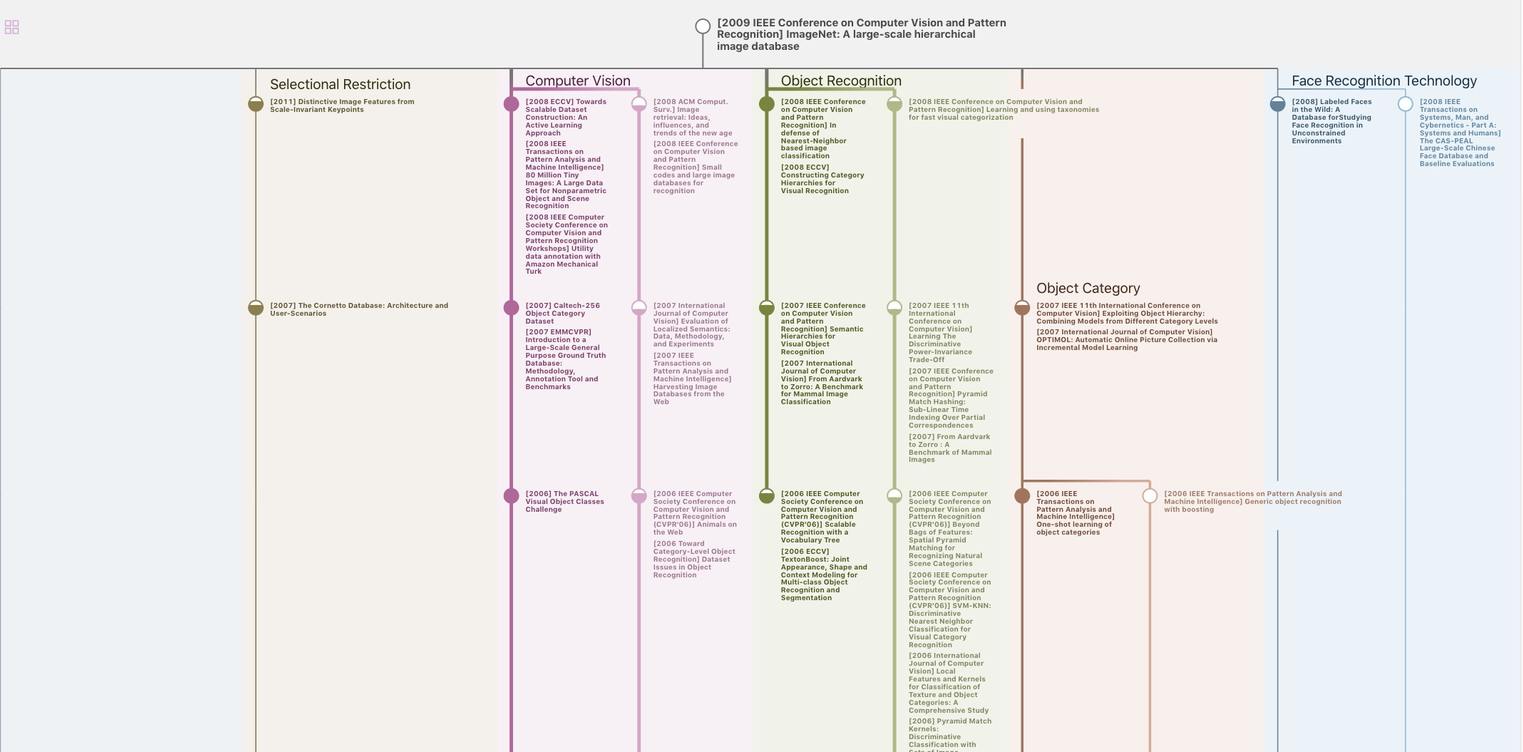
生成溯源树,研究论文发展脉络
Chat Paper
正在生成论文摘要