Names, Nicknames, and Spelling Errors: Protecting Participant Identity in Learning Analytics of Online Discussions
LAK2023: LAK23: 13th International Learning Analytics and Knowledge Conference(2023)
摘要
Messages exchanged between participants in online discussion forums often contain personal names and other details that need to be redacted before the data is used for research purposes in learning analytics. However, removing the names entirely makes it harder to track the exchange of ideas between individuals within a message thread and across threads, and thereby reduces the value of this type of conversational data. In contrast, the consistent use of pseudonyms allows contributions from individuals to be tracked across messages, while also hiding the real identities of the contributors. Several factors can make it difficult to identify all instances of personal names that refer to the same individual, including spelling errors and the use of shortened forms. We developed a semi-automated approach for replacing personal names with consistent pseudonyms. We evaluated our approach on a data set of over 1,700 messages exchanged during a distance-learning course, and compared it to a general-purpose pseudonymisation tool that used deep neural networks to identify names to be redacted. We found that our tailored approach out-performed the general-purpose tool in both precision and recall, correctly identifying all but 31 substitutions out of 2,888.
更多查看译文
AI 理解论文
溯源树
样例
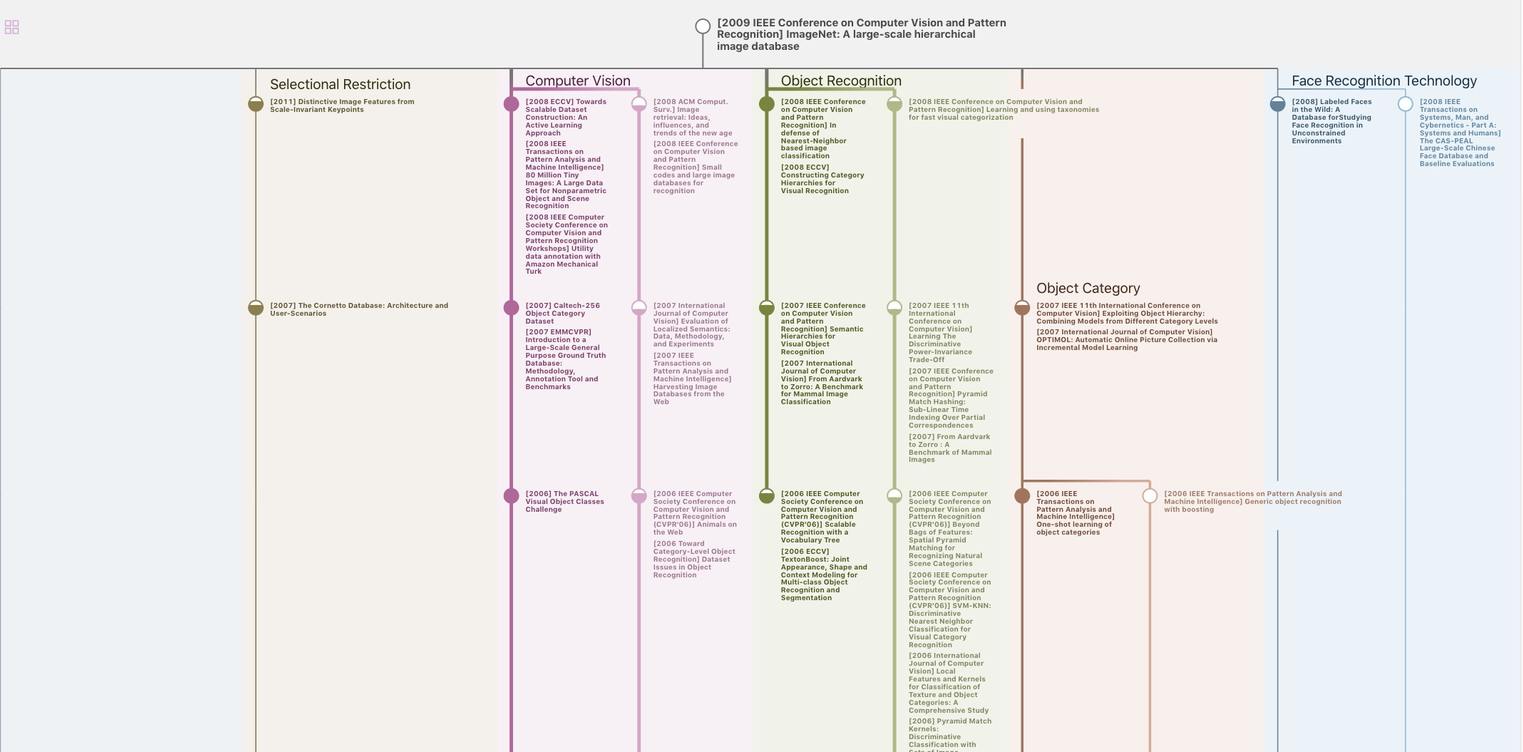
生成溯源树,研究论文发展脉络
Chat Paper
正在生成论文摘要