Slack-Factor-Based Fuzzy Support Vector Machine for Class Imbalance Problems
ACM Transactions on Knowledge Discovery from Data(2023)
摘要
Class imbalance and noisy data widely exist in real-world problems, and the support vector machine (SVM) is hard to construct good classifiers on these data. Fuzzy SVMs (FSVMs), as variants of SVM, use a fuzzy membership function both to reflect the samples’ importance and to remove the impact of noises, and employ cost-sensitive technology to address the class imbalance. They can handle the noise and class imbalance problems in many cases; however, the fuzzy membership functions are often affected by the class imbalance data, leading to inaccurate measures for samples’ performance and affecting the performance of FSVMs. To solve this problem, we design a new fuzzy membership function and combine it with cost-sensitive learning to deal with the class imbalance problem with noisy data, named Slack-Factor-based FSVM (SFFSVM). In SFFSVM, the relative distances between samples and an estimated hyperplane, called slack factors, are used to define the fuzzy membership function. To eliminate the impact of class imbalance on the function and gain more accurate samples’ importance, we rectify the importance according to the positional relationship between the estimated hyperplane and the optimal hyperplane of the problem, and the slack factors of samples. Comprehensive experiments on artificial and real-world datasets demonstrate that SFFSVM outperforms other comparative methods on F1, MCC, and AUC-PR metrics.
更多查看译文
关键词
Cost-sensitive learning,class imbalance,fuzzy support vector machine,decision hyperplane,fuzzy membership function
AI 理解论文
溯源树
样例
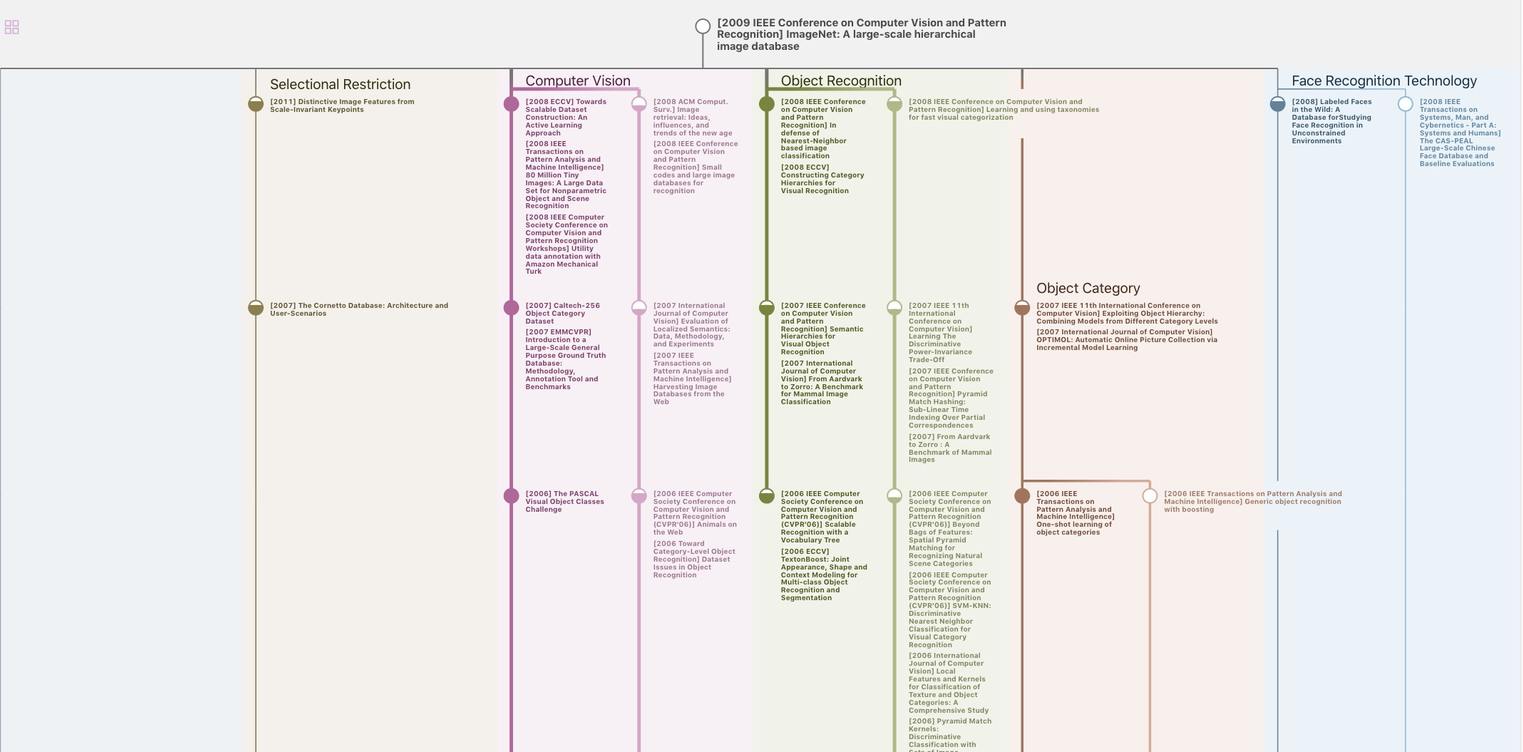
生成溯源树,研究论文发展脉络
Chat Paper
正在生成论文摘要