Improving generalization of double low-rank representation using Schatten- p norm
Pattern Recognition(2023)
摘要
Low-rank representation reveals a highly-informative entailment of sparse matrices, where double low-rank representation (DLRR) presents an effective solution by adopting nuclear norm. However, it is a spe-cial constraint of Schatten-p norm with p = 1 which equally treats all singular values, deviating from the optimal low-rank representation that considers p = 0 . Thus, this paper improves the DLRR generalization of DLRR by relaxing p = 1 into 0 < p < 1 to tighten the low-rank constraint of the Schatten-p norm. With such a relaxation, low-rank optimization is then accelerated, resulting in a lower bound on the calcu-lation complexity. Experiments on unsupervised feature extraction and subspace clustering demonstrate that our low-rank optimization taking 0 < p < 1 achieves a superior performance against state-of-the-art methods.(c) 2023 Elsevier Ltd. All rights reserved.
更多查看译文
关键词
Low-rank representation,Schatten,p norm,Feature extraction,Subspace clustering
AI 理解论文
溯源树
样例
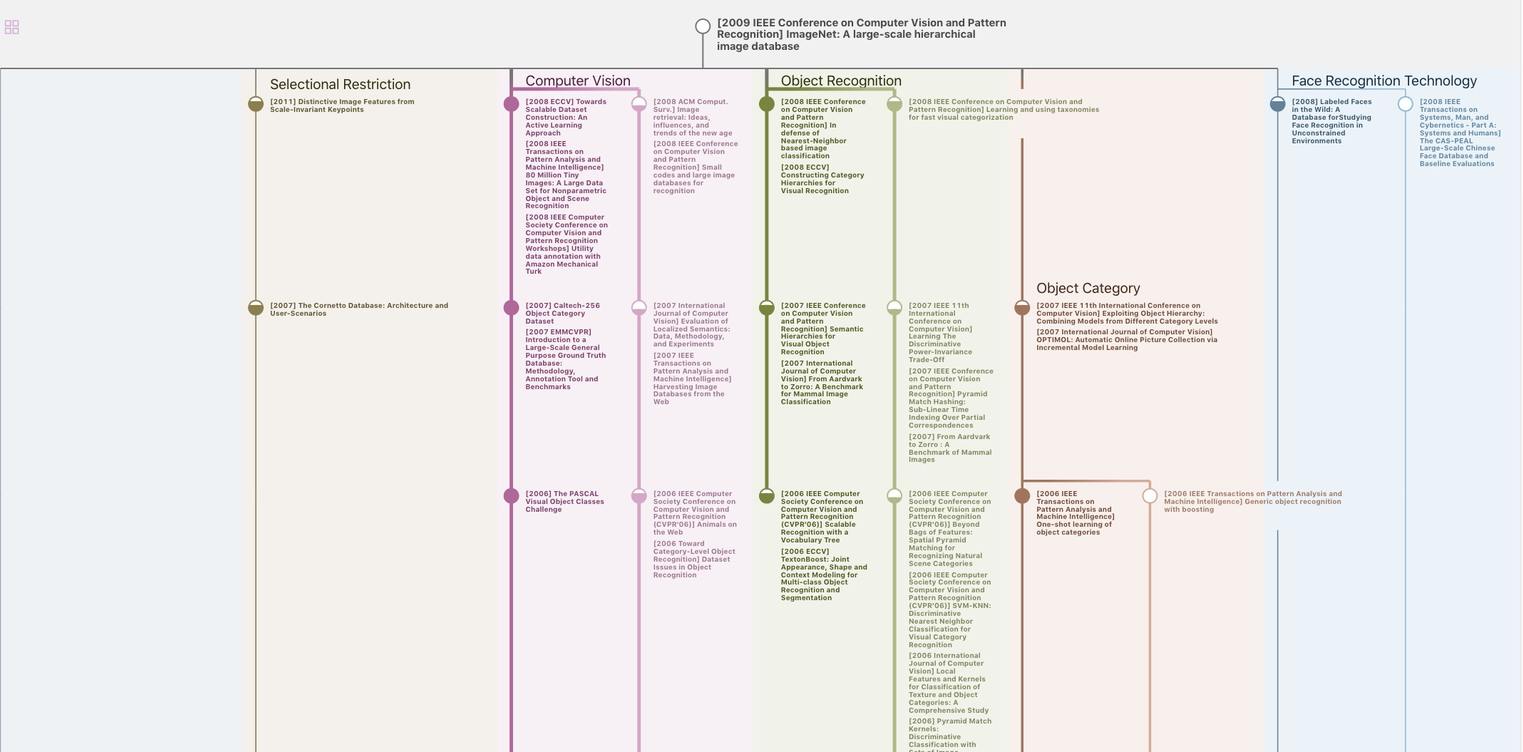
生成溯源树,研究论文发展脉络
Chat Paper
正在生成论文摘要