Discovering unknown response patterns in progress test data to improve the estimation of student performance
BMC Medical Education(2023)
摘要
Background The Progress Test Medizin (PTM) is a 200-question formative test that is administered to approximately 11,000 students at medical universities (Germany, Austria, Switzerland) each term. Students receive feedback on their knowledge (development) mostly in comparison to their own cohort. In this study, we use the data of the PTM to find groups with similar response patterns. Methods We performed k-means clustering with a dataset of 5,444 students, selected cluster number k = 5, and answers as features. Subsequently, the data was passed to XGBoost with the cluster assignment as target enabling the identification of cluster-relevant questions for each cluster with SHAP. Clusters were examined by total scores, response patterns, and confidence level. Relevant questions were evaluated for difficulty index, discriminatory index, and competence levels. Results Three of the five clusters can be seen as “performance” clusters: cluster 0 ( n = 761) consisted predominantly of students close to graduation. Relevant questions tend to be difficult, but students answered confidently and correctly. Students in cluster 1 ( n = 1,357) were advanced, cluster 3 ( n = 1,453) consisted mainly of beginners. Relevant questions for these clusters were rather easy. The number of guessed answers increased. There were two “drop-out” clusters: students in cluster 2 ( n = 384) dropped out of the test about halfway through after initially performing well; cluster 4 ( n = 1,489) included students from the first semesters as well as “non-serious” students both with mostly incorrect guesses or no answers. Conclusion Clusters placed performance in the context of participating universities. Relevant questions served as good cluster separators and further supported our “performance” cluster groupings.
更多查看译文
关键词
Boosting algorithm,Classification,Clustering,Ensemble learning,Explainer,Progress test,Student groups,Supervised machine learning,Unsupervised machine learning,k-means
AI 理解论文
溯源树
样例
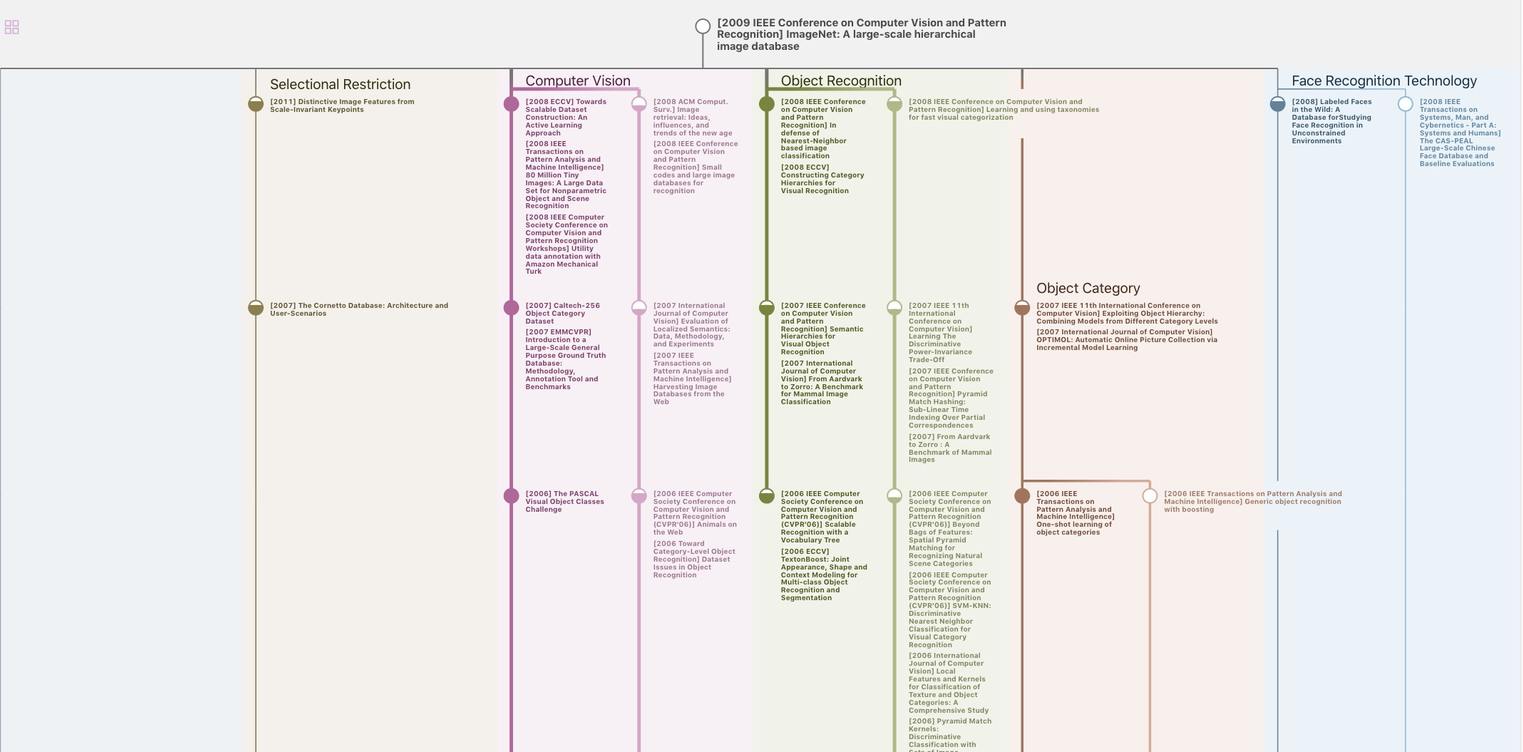
生成溯源树,研究论文发展脉络
Chat Paper
正在生成论文摘要