Reinforcement learning building control approach harnessing imitation learning
ENERGY AND AI(2023)
摘要
Reinforcement learning (RL) has shown significant success in sequential decision making in fields like autonomous vehicles, robotics, marketing and gaming industries. This success has attracted the attention to the RL control approach for building energy systems which are becoming complicated due to the need to optimize for multiple, potentially conflicting, goals like occupant comfort, energy use and grid interactivity. However, for real world applications, RL has several drawbacks like requiring large training data and time, and unstable control behavior during the early exploration process making it infeasible for an application directly to building control tasks. To address these issues, an imitation learning approach is utilized herein where the RL agents starts with a policy transferred from accepted rule based policies and heuristic policies. This approach is successful in reducing the training time, preventing the unstable early exploration behavior and improving upon an accepted rule-based policy - all of these make RL a more practical control approach for real world applications in the domain of building controls.
更多查看译文
关键词
Reinforcement learning,Building controls,Imitation learning,Artificial intelligence
AI 理解论文
溯源树
样例
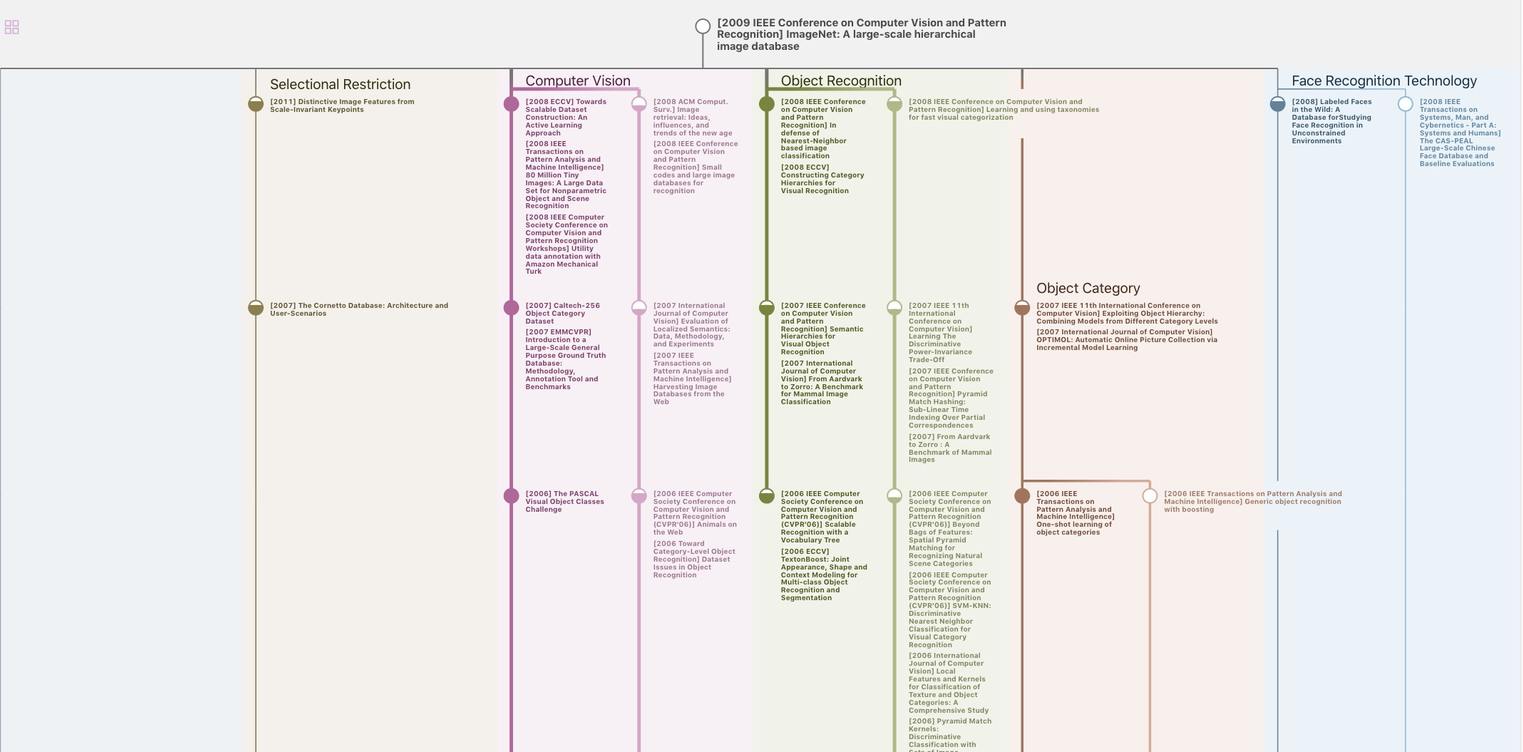
生成溯源树,研究论文发展脉络
Chat Paper
正在生成论文摘要