Continuous mapping of aboveground biomass using Landsat time series
Remote Sensing of Environment(2023)
摘要
Aboveground biomass (AGB) is an essential variable in the study of the carbon cycle, and remote sensing provides the only viable means for mapping and monitoring biomass at large scales in time and space. Still, inconsistencies in biomass estimates are large which necessitates methods to achieve consistent mapping of biomass over time and space. In this study, we combine Lidar-derived biomass-estimates from GLAS measurements, time series analysis of 20 years of Landsat data, and machine learning algorithms to map biomass for a study area in the Amazon basin. The results show that we are able to map AGB consistently over space and time at annual intervals between 1999 and 2019, with a root-mean-square error (RMSE) of 64 to 92 Mg AGB ha−1 (depending on the evaluation methodology used) for the best performing models, with AGB estimates ranging between 0 to 600 Mg AGB ha−1. Models run with the Extreme gradient boosting (XGBoost) resulted in the lowest errors. The presented methodological approach makes it possible to map biomass over time in large areas with wide biomass ranges, and without saturation below 400 Mg AGB ha−1.
更多查看译文
关键词
Biomass,Landsat,Time series,GLAS,Amazon basin,CCDC,Machine learning,Lidar
AI 理解论文
溯源树
样例
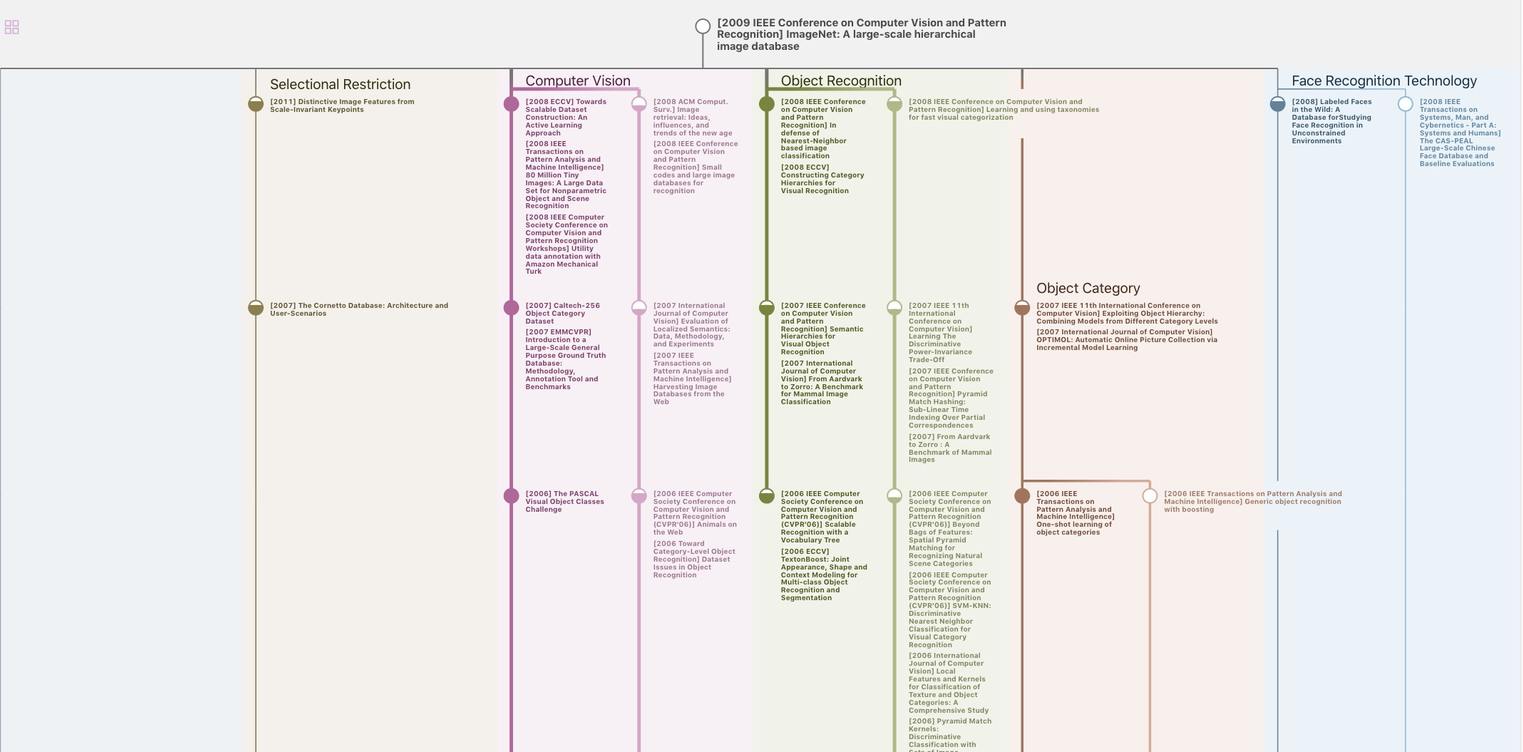
生成溯源树,研究论文发展脉络
Chat Paper
正在生成论文摘要