Symmetric Rank-$k$ Methods
arXiv (Cornell University)(2023)
摘要
This paper proposes a novel class of block quasi-Newton methods for convex optimization which we call symmetric rank-$k$ (SR-$k$) methods. Each iteration of SR-$k$ incorporates the curvature information with $k$ Hessian-vector products achieved from the greedy or random strategy. We prove SR-$k$ methods have the local superlinear convergence rate of $\mathcal{O}\big((1-k/d)^{t(t-1)/2}\big)$ for minimizing smooth and strongly self-concordant function, where $d$ is the problem dimension and $t$ is the iteration counter. This is the first explicit superlinear convergence rate for block quasi-Newton methods and it successfully explains why block quasi-Newton methods converge faster than standard quasi-Newton methods in practice.
更多查看译文
关键词
methods
AI 理解论文
溯源树
样例
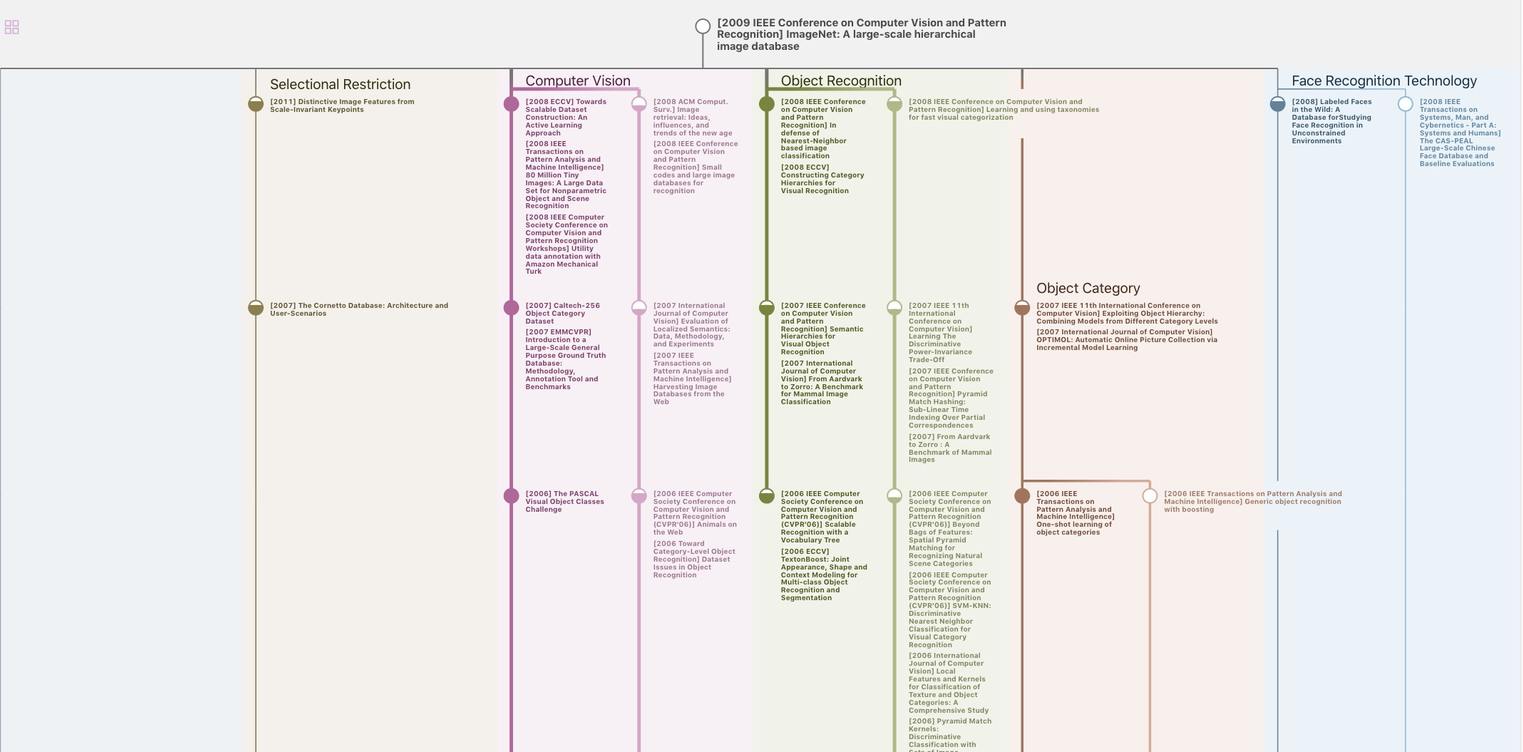
生成溯源树,研究论文发展脉络
Chat Paper
正在生成论文摘要