Charged particle reconstruction in CLAS12 using Machine Learning
Computer Physics Communications(2023)
摘要
In this work, we present studies of track parameter reconstruction from raw information in CLAS12 detector's Drift Chambers, using Machine Learning (ML). We study the resolution of tracks reconstructed with different types of ML models/algorithms, including Multi-Layer Perceptron (MLP), Extremely Randomized Trees (ERT) and Gradient Boosting Trees (GBT) using simulated data. The resulting ML model is capable of reconstructing track parameters (particle momentum, and polar and azimuthal angles) with accuracy similar to Hit Based (HB) tracking code, but 150 times faster. Moreover, physics reactions can be identified using the particles reconstructed by the neural network in real-time (with a rate of about 34 kHz) during experimental data collection. The developed model can be used in numerous applications, such as triggering specific physics reactions in real-time, detector performance monitoring, and real-time detector calibration.(c) 2023 Elsevier B.V. All rights reserved.
更多查看译文
关键词
Machine Learning,Jefferson Lab,Tracking,Drift Chambers,CLAS12,Particle accelerators
AI 理解论文
溯源树
样例
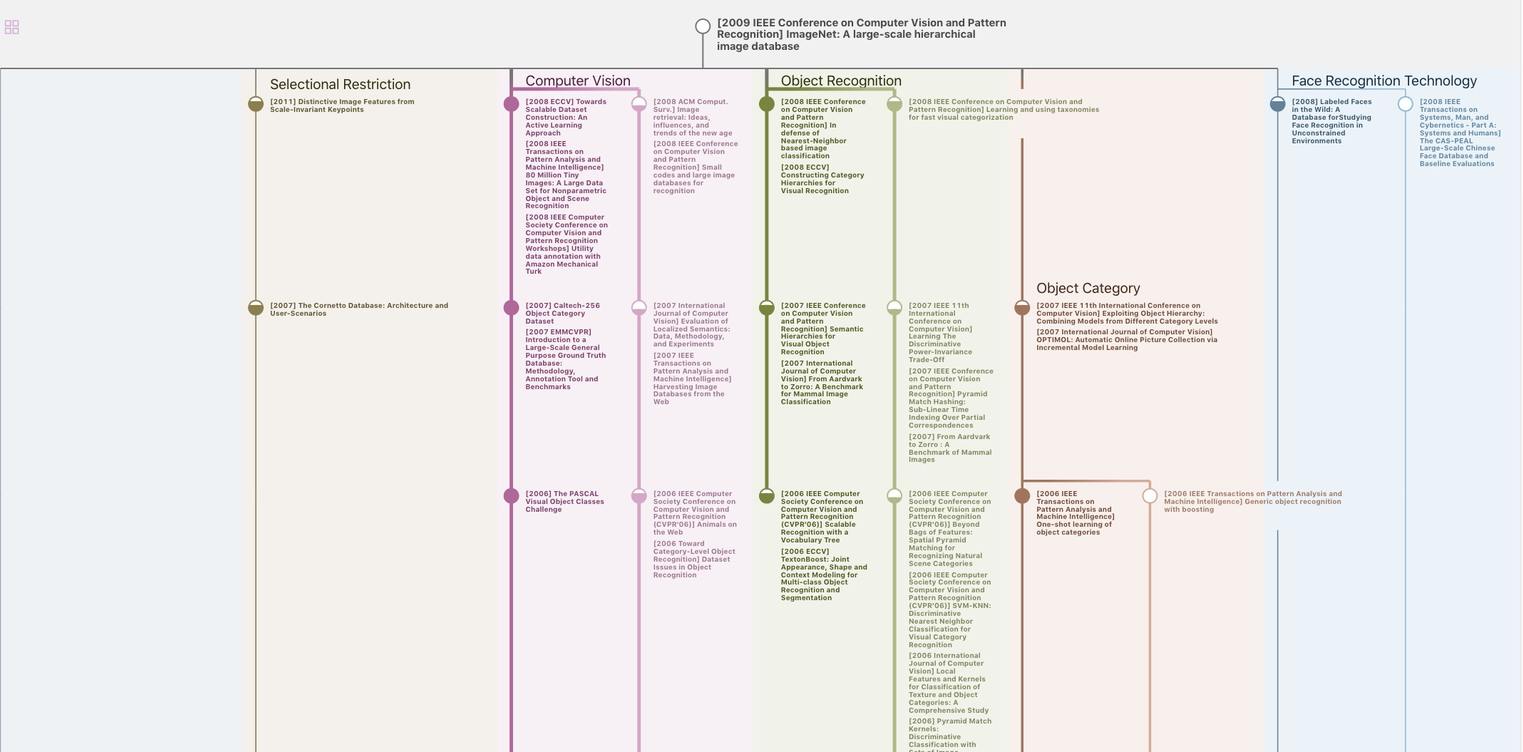
生成溯源树,研究论文发展脉络
Chat Paper
正在生成论文摘要