GAN-RXA: A Practical Scalable Solution to Receiver-Agnostic Transmitter Fingerprinting
IEEE Transactions on Cognitive Communications and Networking(2023)
摘要
Radio frequency fingerprinting has been proposed for device identification.
However, experimental studies also demonstrated its sensitivity to deployment
changes. Recent works have addressed channel impacts by developing robust
algorithms accounting for time and location variability, but the impacts of
receiver impairments on transmitter fingerprints are yet to be solved. In this
work, we investigate the receiver-agnostic transmitter fingerprinting problem,
and propose a novel two-stage supervised learning framework (RXA) to address
it. In the first stage, our approach calibrates a receiver-agnostic transmitter
feature-extractor. We also propose two deep-learning approaches (SD-RXA and
GAN-RXA) in this first stage to improve the receiver-agnostic property of the
RXA framework. In the second stage, the calibrated feature-extractor is
utilized to train a transmitter classifier with only one receiver. We evaluate
the proposed approaches on the transmitter identification problem using a
large-scale WiFi dataset. We show that when a trained transmitter-classifier is
deployed on new receivers, the RXA framework can improve the classification
accuracy by 19.5
naive approach without calibration. Moreover, GAN-RXA can further increase the
closed-set classification accuracy by 5.0
by 12.5
更多查看译文
AI 理解论文
溯源树
样例
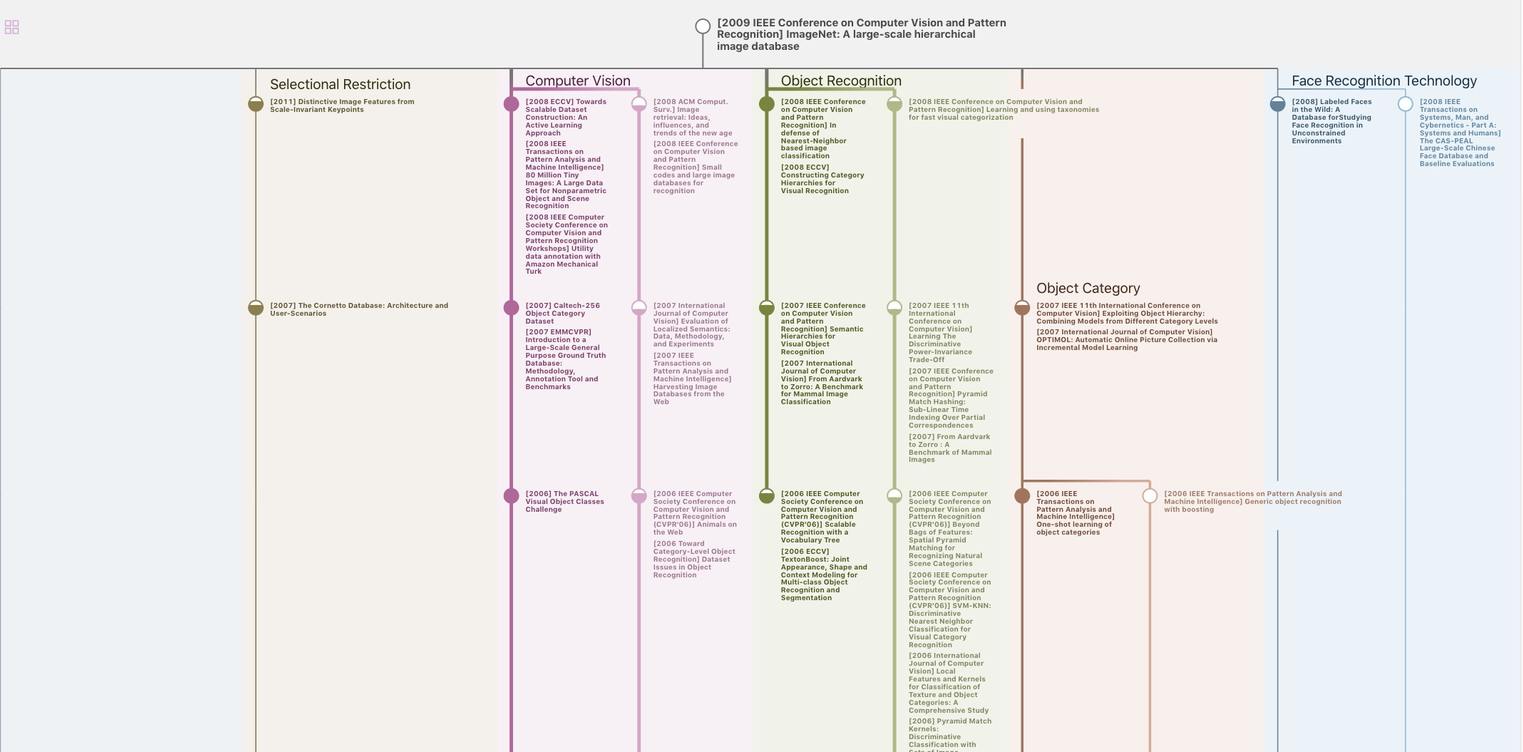
生成溯源树,研究论文发展脉络
Chat Paper
正在生成论文摘要