Dilated causal convolutional neural networks for forecasting zone airflow to estimate short-term energy consumption
Energy and Buildings(2023)
摘要
This paper investigates the use of dilated causal convolutional neural networks for fine-grained temporal forecasting of building zone states. Specifically, we build and evaluate models using a small set of exogenous features (e.g., external temperature) to autoregressively predict zone airflow setpoints every minute for a 24-h prediction window. We carefully explore the trade-off between generality and specificity in these models, training and evaluating them based on zone, zone type, month, season, and combinations thereof. When evaluated for a commercial office building in Eastern Washington with 16 zones served by variable air volume air handling units, we find that the highest performance comes from a zone-specific, season-agnostic approach; with it, we obtain an R2 of 0.704 (averaged over zones) and an average normalized root mean square error (nRMSE) of 0.111. In contrast, the most general model (trained across all zones and seasons) yields an R2 of only 0.416 and a nRMSE of 0.168, while a baseline zone-specific reduced order model obtains 0.443 R2 and 0.159 nRMSE. We also report on factors affecting airflow forecasting performance, on the ability of models trained on a specific zone to generalize to other zones, and on the capability of those models trained on a specific month to generalize to other months.
更多查看译文
关键词
Airflow setpoint,Deep learning,Neural networks,Dilated causal convolution,Time series forecasting,Transactive control and coordination
AI 理解论文
溯源树
样例
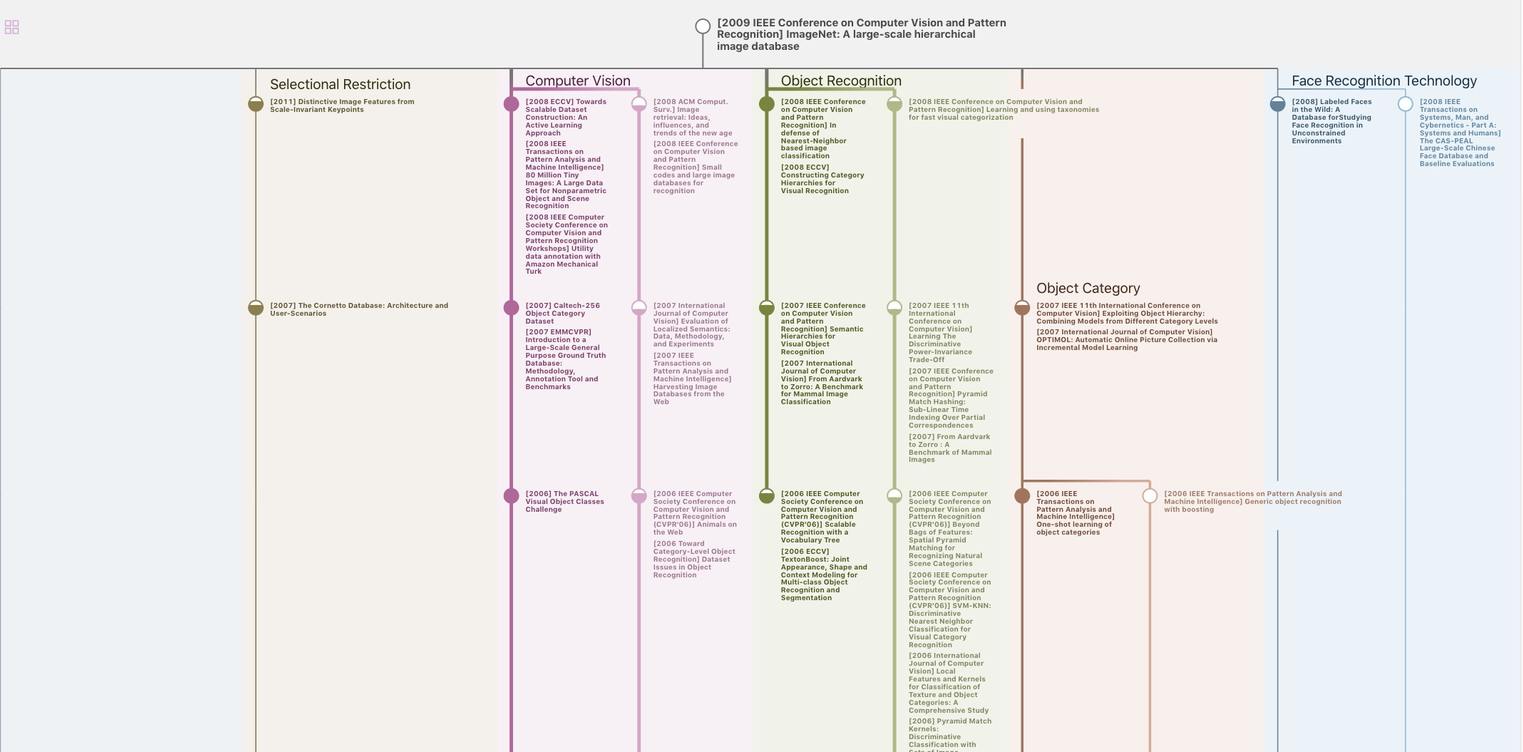
生成溯源树,研究论文发展脉络
Chat Paper
正在生成论文摘要