An Improved Yaw Control Algorithm for Wind Turbines via Reinforcement Learning
ECML/PKDD (5)(2023)
摘要
Yaw misalignment, measured as the difference between the wind direction and the nacelle position of a wind turbine, has consequences on the power output, the safety and the lifetime of the turbine and its wind park as a whole. We use reinforcement learning to develop a yaw control agent to minimise yaw misalignment and optimally reallocate yaw resources, prioritising high-speed segments, while keeping yaw usage low. To achieve this, we carefully crafted and tested the reward metric to trade-off yaw usage versus yaw alignment (as proportional to power production), and created a novel simulator (environment) based on real-world wind logs obtained from a REpower MM82 2MW turbine. The resulting algorithm decreased the yaw misalignment by 5.5% and 11.2% on two simulations of 2.7 hours each, compared to the conventional active yaw control algorithm. The average net energy gain obtained was 0.31% and 0.33% respectively, compared to the traditional yaw control algorithm. On a single 2MW turbine, this amounts to a 1.5k-2.5k euros annual gain, which sums up to very significant profits over an entire wind park.
更多查看译文
关键词
Wind turbine control, Multi-objective reinforcement learning, Yaw control
AI 理解论文
溯源树
样例
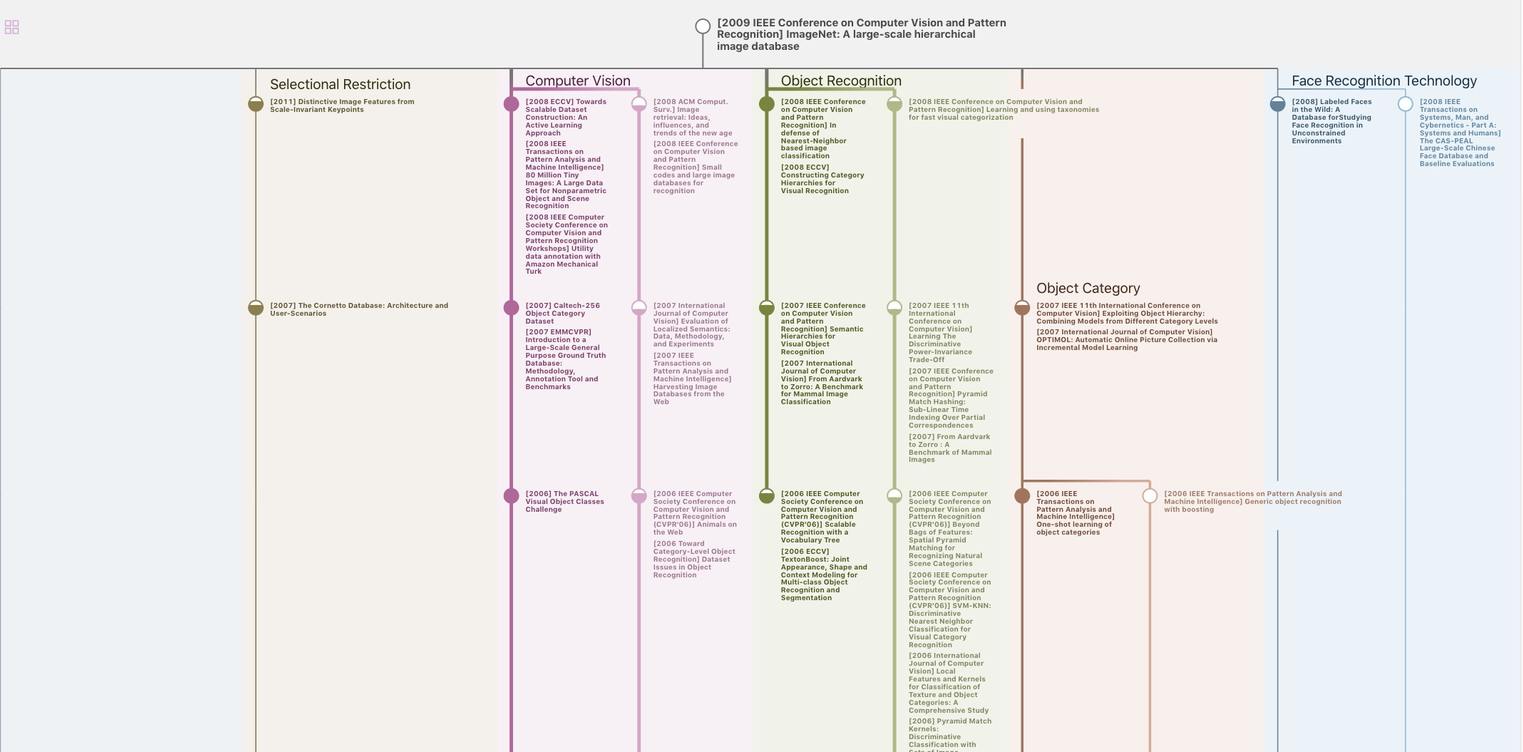
生成溯源树,研究论文发展脉络
Chat Paper
正在生成论文摘要