Interactive Toolbox for Two-Dimensional Gaussian Mixture Modeling
ECML/PKDD (6)(2023)
摘要
Research data obtained during economics or human studies experiments often displays a complex distribution. Even in the two-dimensional case, the statistical identification of subgroups in research data poses an analytical challenge. Here we introduce an interactive R-based tool called "AdaptGauss2D". It enables a valid identification of a meaningful multimodal structure in two-dimensional data. With a human-in-the-loop approach, a Gaussian mixture model (GMM) can be fitted to the data. The interactive interface allows a supervised selection of the number and parameters of the GMM based on various visualizations. Integrating a Human-in-the-loop into the process of modeling two-dimensional gaussian mixtures enables the expectation-maximization (EM) algorithm to adapt to more complex GMM compared to the standard non-interactive approach. The work demonstrates that the interactive modeling process for GMM improves the quality of the model in contrast to non-interactive modeling. The improvement is shown using the datasets of EngyTime and a large flow cytometry dataset. The R package "AdaptGauss2D" is available on GitHub https://github.com/Mthrun/Ada ptGauss2D.
更多查看译文
关键词
Gaussian mixtures, Human-in-the-loop, Interactive ML
AI 理解论文
溯源树
样例
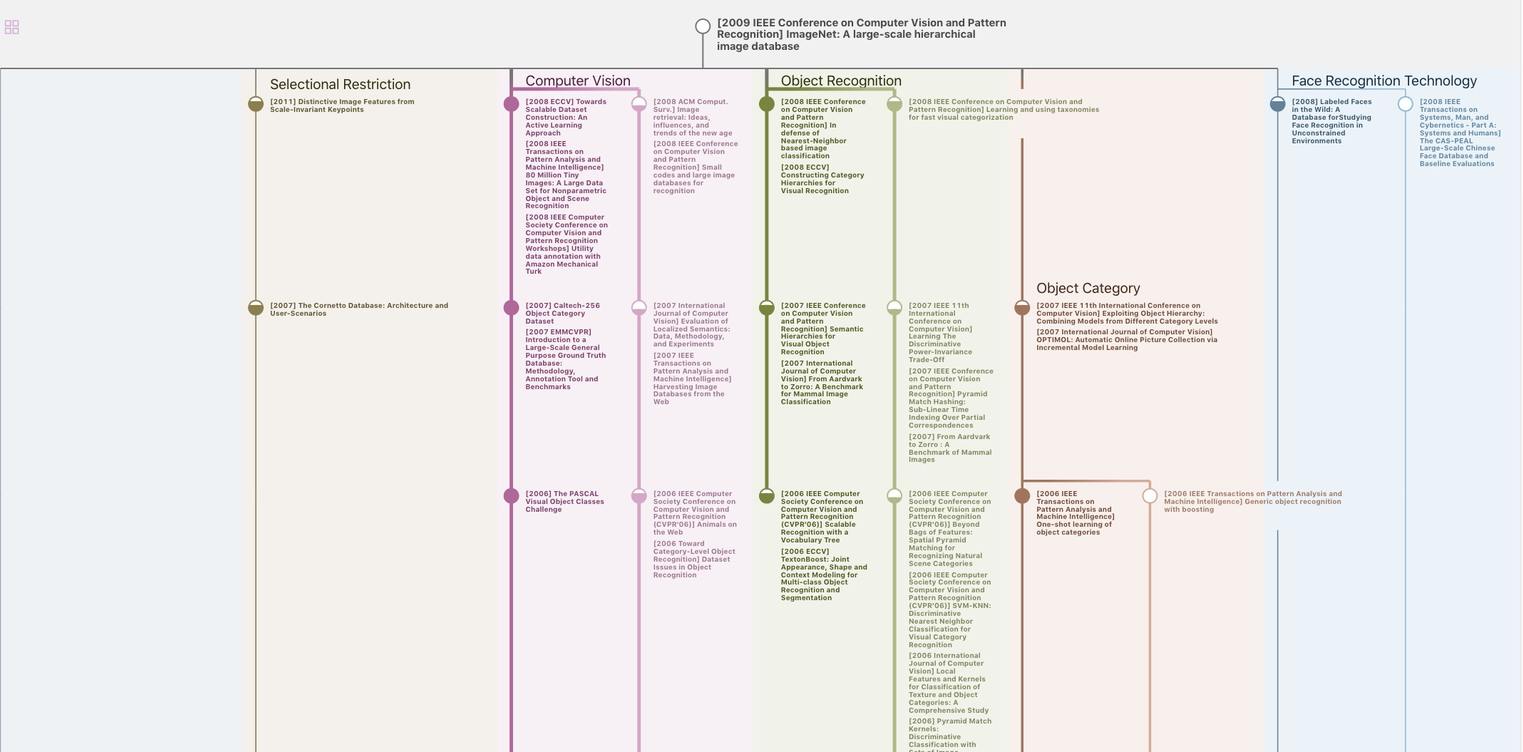
生成溯源树,研究论文发展脉络
Chat Paper
正在生成论文摘要