Machine learning study on time–temperature–transformation diagram of carbon and low-alloy steel
Journal of Iron and Steel Research International(2023)
摘要
Time–temperature–transformation (TTT) diagram plays a critical role in designing appropriate heat treatment process of steels by describing the relationship among holding time, temperature, and quantities of phase transformation. Making predictions for TTT diagrams of new steel rapidly and accurately is therefore of much practical importance, especially for costly and time-consuming experimental determination. Here, TTT diagrams for carbon and low-alloy steels were predicted using machine learning methods. Five commonly used machine learning (ML) algorithms, backpropagation artificial neural network (BP network), LibSVM, k-nearest neighbor, Bagging, and Random tree, were adopted to select appropriate models for the prediction. The results illustrate that Bagging is the optimal model for the prediction of pearlite transformation and bainite transformation, and BP network is the optimal model for martensite transformation. Finally, the ML framework composed of Bagging and BP network models was applied to predict the entire TTT diagram. Additionally, the ML models show superior performance on the prediction of testing samples than the commercial software JMatPro.
更多查看译文
关键词
machine learning study,machine learning,steel,time–temperature–transformation diagram,low-alloy
AI 理解论文
溯源树
样例
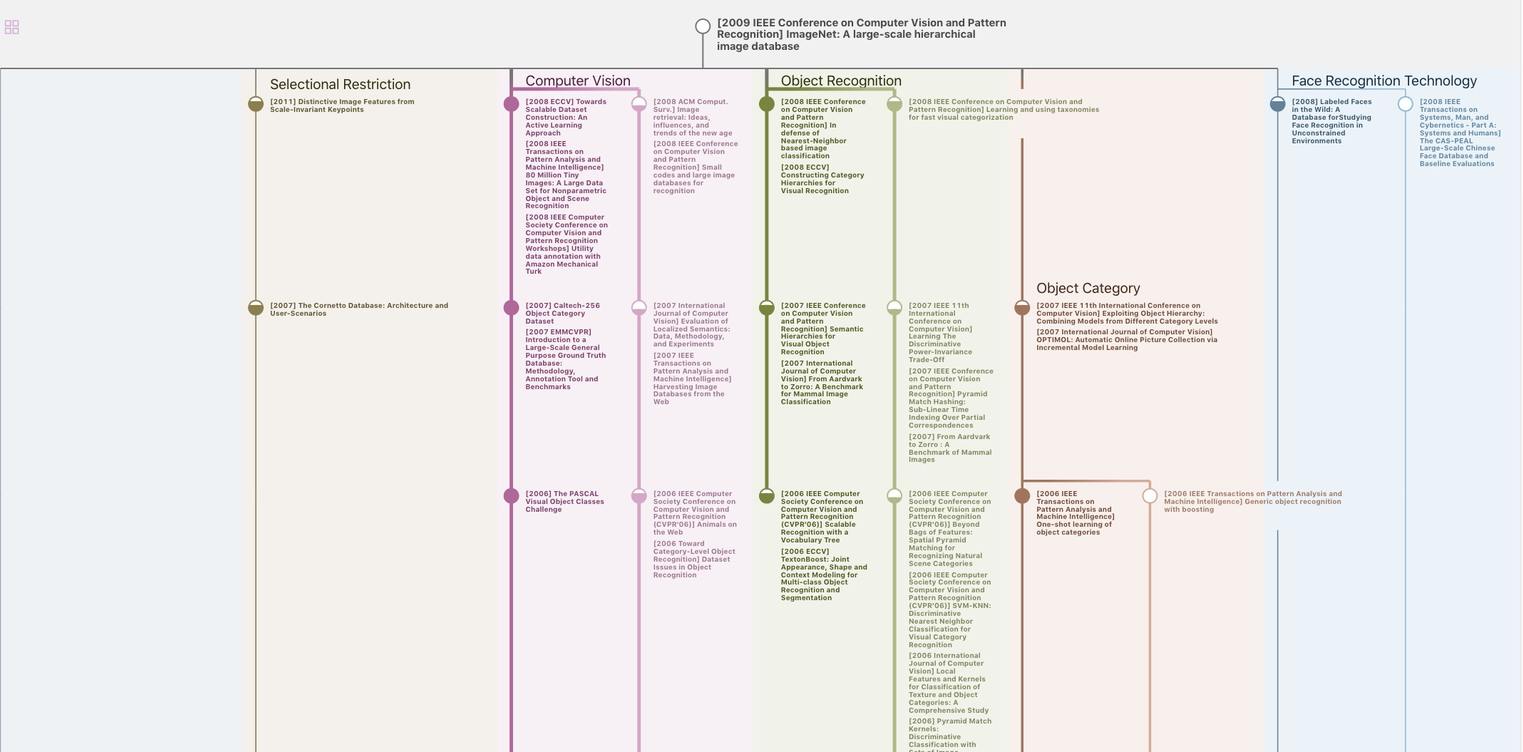
生成溯源树,研究论文发展脉络
Chat Paper
正在生成论文摘要