Topological Reconstruction of Particle Physics Processes using Graph Neural Networks
CoRR(2023)
摘要
We present a new approach, the Topograph, which reconstructs underlying physics processes, including the intermediary particles, by leveraging underlying priors from the nature of particle physics decays and the flexibility of message passing graph neural networks. The Topograph not only solves the combinatoric assignment of observed final state objects, associating them to their original mother particles, but directly predicts the properties of intermediate particles in hard scatter processes and their subsequent decays. In comparison to standard combinatoric approaches or modern approaches using graph neural networks, which scale exponentially or quadratically, the complexity of Topographs scales linearly with the number of reconstructed objects. We apply Topographs to top quark pair production in the all hadronic decay channel, where we outperform the standard approach and match the performance of the state-of-the-art machine learning technique.
更多查看译文
关键词
topological reconstruction,particle physics processes,neural networks
AI 理解论文
溯源树
样例
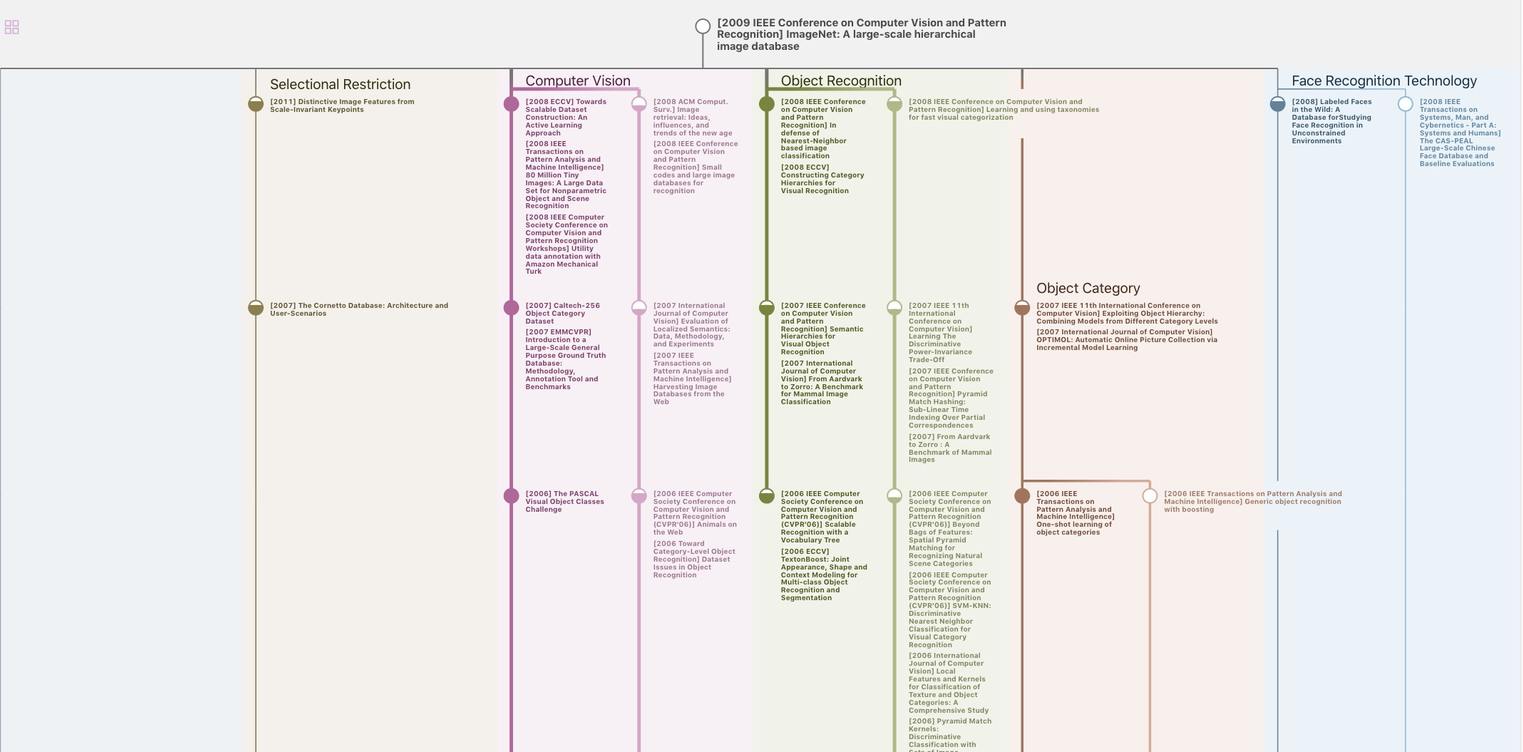
生成溯源树,研究论文发展脉络
Chat Paper
正在生成论文摘要