Towards Fair Patient-Trial Matching via Patient-Criterion Level Fairness Constraint.
CoRR(2024)
摘要
Clinical trials are indispensable in developing new treatments, but they face obstacles in patient recruitment and retention, hindering the enrollment of necessary participants. To tackle these challenges, deep learning frameworks have been created to match patients to trials. These frameworks calculate the similarity between patients and clinical trial eligibility criteria, considering the discrepancy between inclusion and exclusion criteria. Recent studies have shown that these frameworks outperform earlier approaches. However, deep learning models may raise fairness issues in patient-trial matching when certain sensitive groups of individuals are underrepresented in clinical trials, leading to incomplete or inaccurate data and potential harm. To tackle the issue of fairness, this work proposes a fair patient-trial matching framework by generating a patient-criterion level fairness constraint. The proposed framework considers the inconsistency between the embedding of inclusion and exclusion criteria among patients of different sensitive groups. The experimental results on real-world patient-trial and patient-criterion matching tasks demonstrate that the proposed framework can successfully alleviate the predictions that tend to be biased.
更多查看译文
关键词
fairness,patient-trial,patient-criterion
AI 理解论文
溯源树
样例
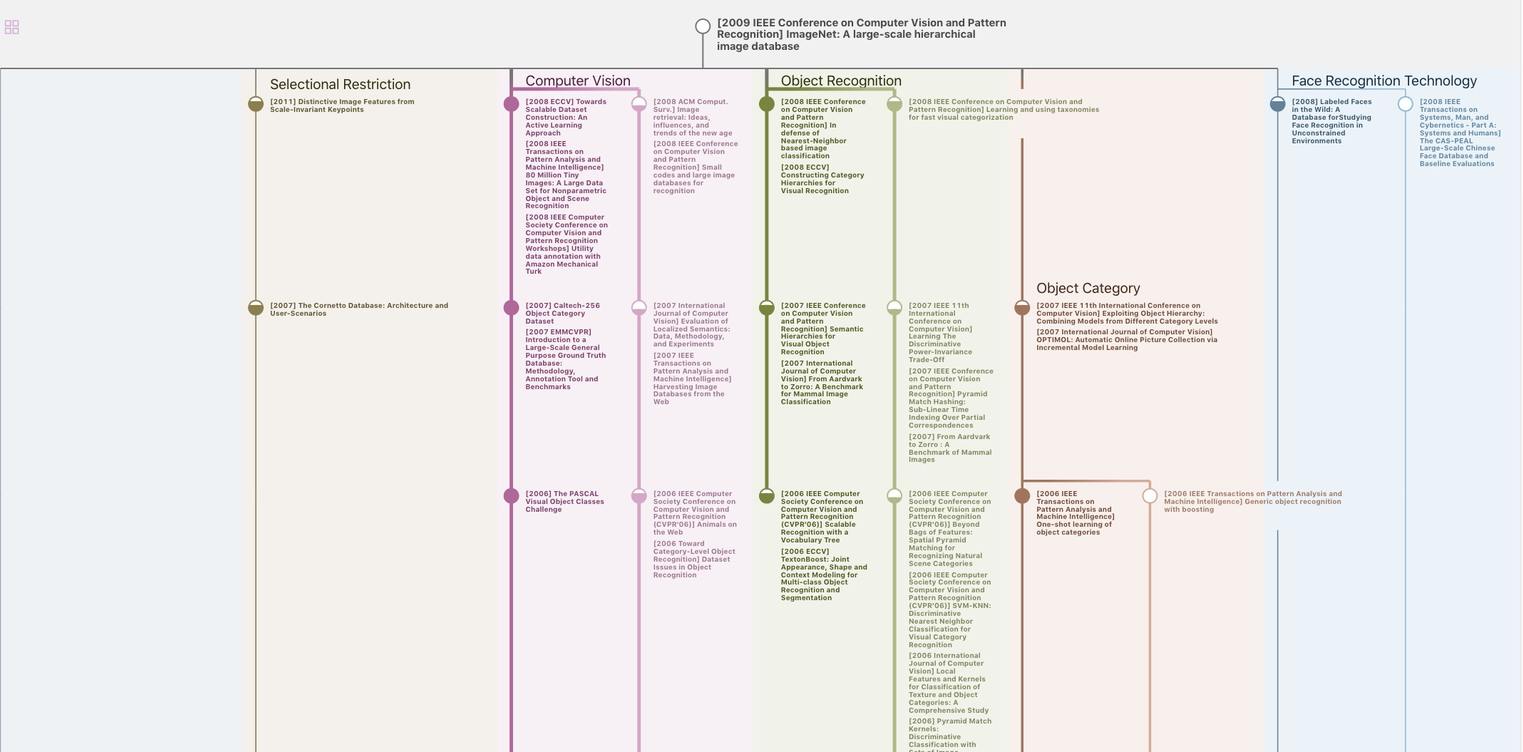
生成溯源树,研究论文发展脉络
Chat Paper
正在生成论文摘要