Robust self-sensing shape memory alloy actuator using a machine learning approach
Sensors and Actuators A: Physical(2023)
摘要
Shape memory alloys are metal alloys that have multiple crystalline states, which can be accessed through heating and cooling. These actuators have several attractive properties, such as a high strength-to-weight ratio, robustness, and compact structure. Added to this, shape memory alloys have a self-sensing property. However, shape memory alloy wires typically have a low contraction of only ∼4%–5%. To overcome this shortcoming, shape memory alloy actuators can use a passive base layer in a morphing configuration to amplify the deformation. By including voltage probes at the base of a unimorph shape memory alloy actuator, self-sensing of the actuator’s configuration can be achieved, without encumbering the actuator with extra mass or wires. Due to the compact nature of this actuator, the voltage probes are necessarily close together, which drastically increases the errors of the estimated deformations. To correct these large errors, a machine learning approach is used to unlock this self-sensing ability for a compact shape memory alloy actuator. Of note, this approach, instead of being a black box method, builds on a self-sensing theory of shape memory alloys. This method of self-sensing with machine learning calibration is capable of predicting the location of the end of the actuator with less than 3% error, even for large deformations.
更多查看译文
关键词
Shape memory alloy,Self-sensing,Machine learning
AI 理解论文
溯源树
样例
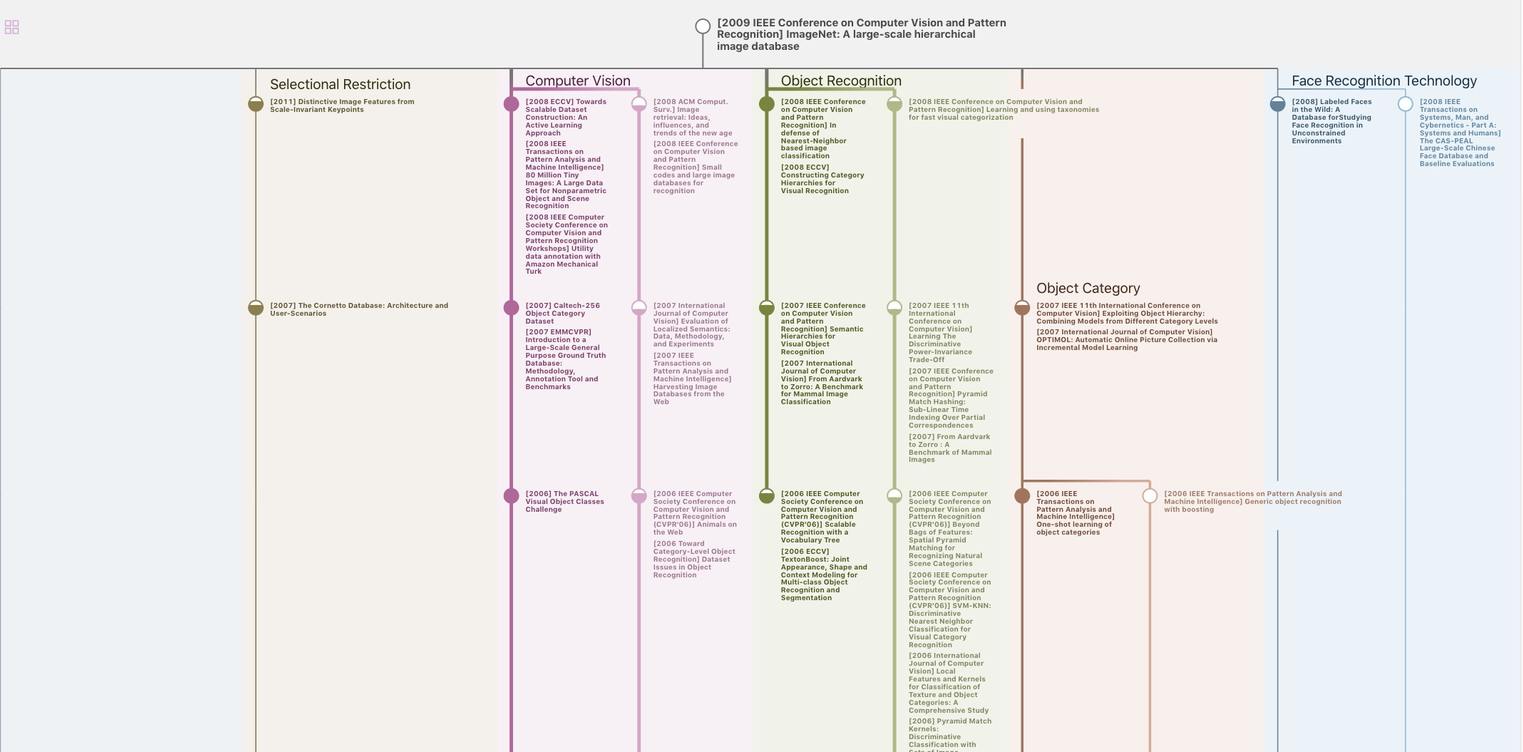
生成溯源树,研究论文发展脉络
Chat Paper
正在生成论文摘要