Online Active Learning Framework for Data Stream Classification With Density-Peaks Recognition.
IEEE Access(2023)
摘要
This paper considers a practical scenario - data stream classification, and uses online active learning (OAL) to assist the classifier. Most active learning are proposed based on label uncertainty, but the methods based on representativeness are faced with difficulties in stream scenarios. The sampling criteria based on representativeness are usually fixed, which makes it difficult for them to work effectively in dynamic sample spaces, especially for concept drift streams. This paper devises a novel OAL framework based on sample representativeness. The framework uses local nearest-neighbor relation to measure the representativeness of unlabeled samples and divides the maximum influence space of the representative sample. We also develop an independent mechanism to identify and store short-term cluster fragments to ensure the integrity of information. The framework is deployed to the online environment and adapts to any incremental learner. Simulation experiments on multiple datasets and classifiers show that our method can help primary classifier deal with data stream environment effectly. Compared with other online active learning, the method can achieve more stable accuracy and better anti-noise ability under fewer budget.
更多查看译文
关键词
Clustering algorithms,Uncertainty,Classification algorithms,Data mining,Training data,Engines,Unsupervised learning,Data stream classification,online active learning,density-peak clustering,cluster fragmentation
AI 理解论文
溯源树
样例
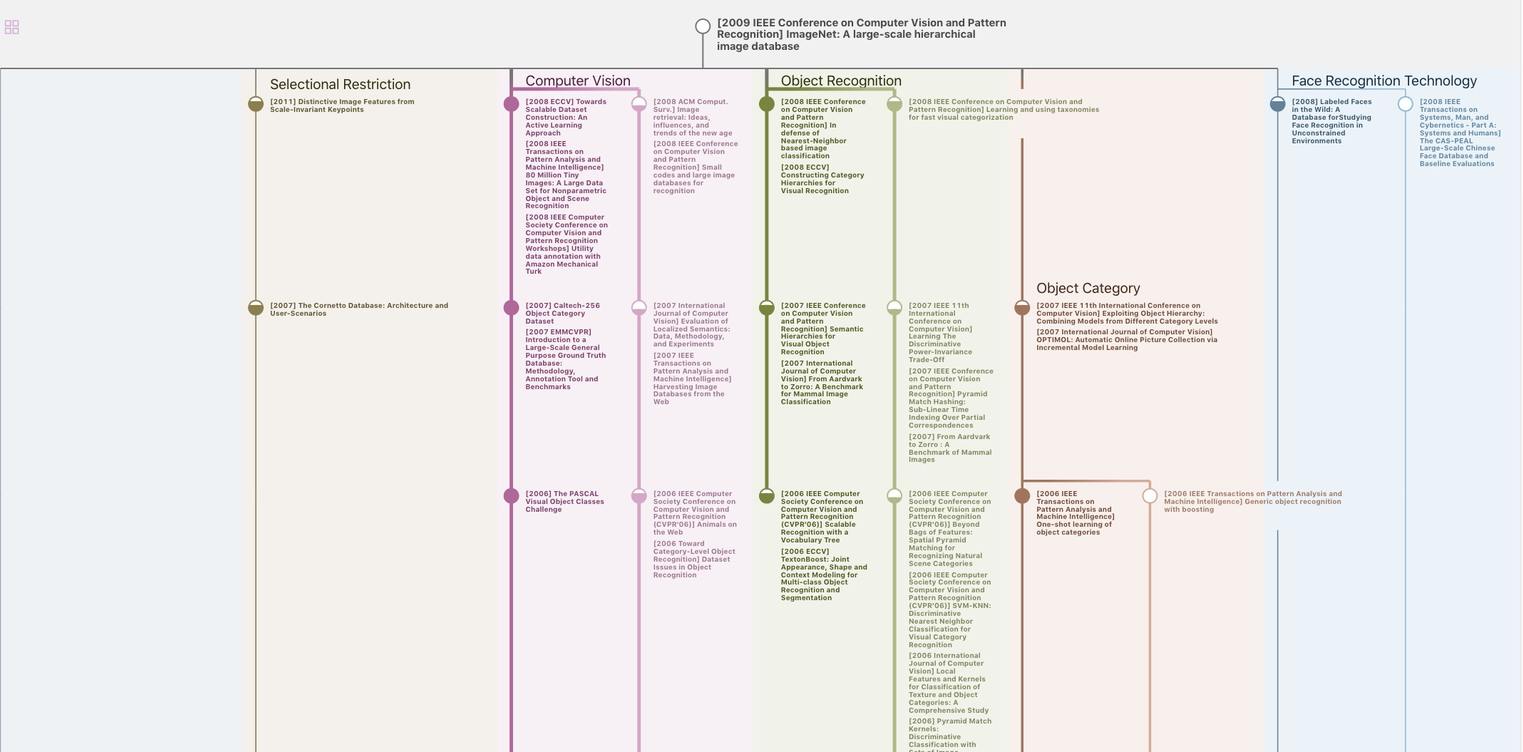
生成溯源树,研究论文发展脉络
Chat Paper
正在生成论文摘要