Stochastic Decision Petri Nets
arxiv(2023)
摘要
We introduce stochastic decision Petri nets (SDPNs), which are a form of stochastic Petri nets equipped with rewards and a control mechanism via the deactivation of controllable transitions. Such nets can be translated into Markov decision processes (MDPs), potentially leading to a combinatorial explosion in the number of states due to concurrency. Hence we restrict ourselves to instances where nets are either safe, free-choice and acyclic nets (SAFC nets) or even occurrence nets and policies are defined by a constant deactivation pattern. We obtain complexity-theoretic results for such cases via a close connection to Bayesian networks, in particular we show that for SAFC nets the question whether there is a policy guaranteeing a reward above a certain threshold is $\mathsf{NP}^\mathsf{PP}$-complete. We also introduce a partial-order procedure which uses an SMT solver to address this problem.
更多查看译文
关键词
stochastic decision petri nets
AI 理解论文
溯源树
样例
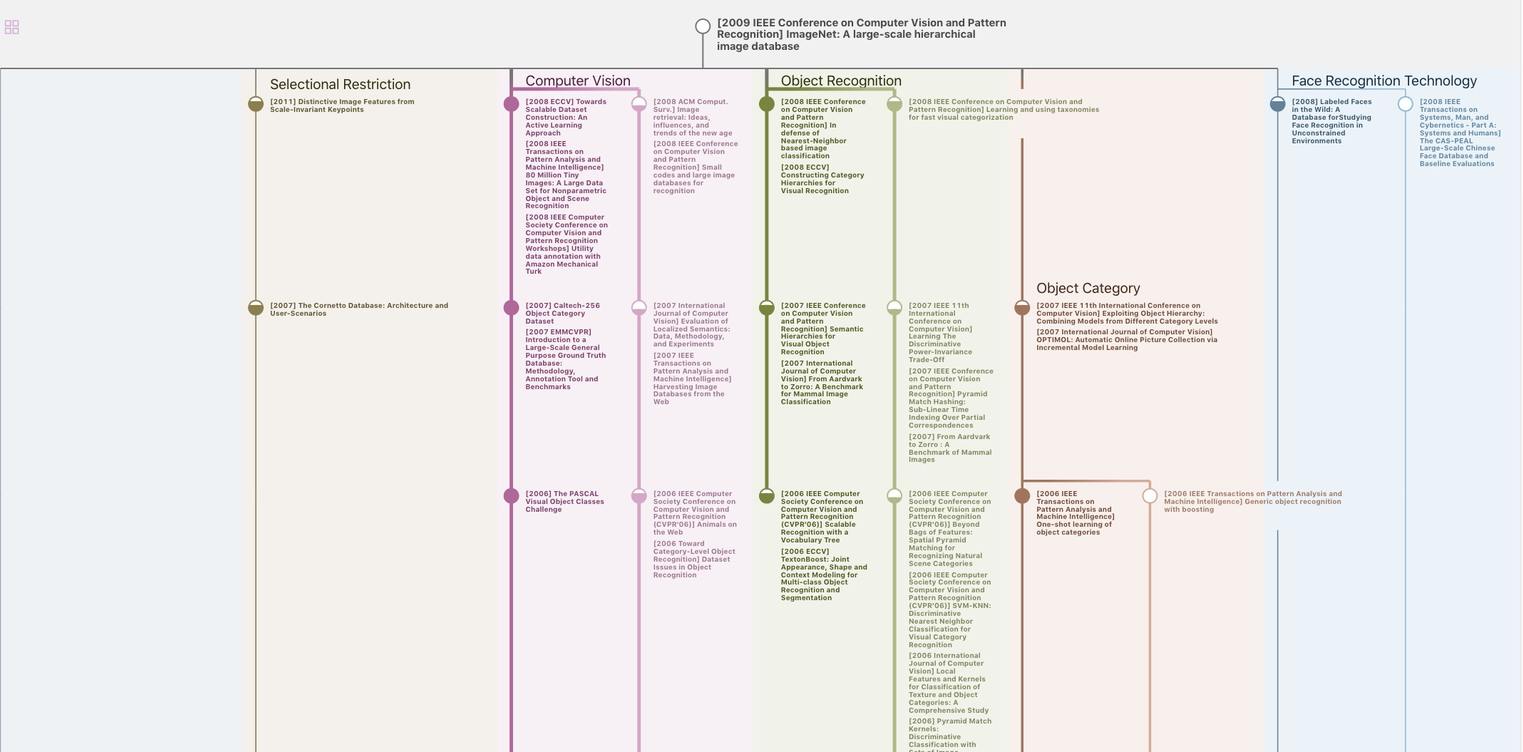
生成溯源树,研究论文发展脉络
Chat Paper
正在生成论文摘要