Self-distillation for Surgical Action Recognition
MEDICAL IMAGE COMPUTING AND COMPUTER ASSISTED INTERVENTION, MICCAI 2023, PT IX(2023)
摘要
Surgical scene understanding is a key prerequisite for context-aware decision support in the operating room. While deep learning-based approaches have already reached or even surpassed human performance in various fields, the task of surgical action recognition remains a major challenge. With this contribution, we are the first to investigate the concept of self-distillation as a means of addressing class imbalance and potential label ambiguity in surgical video analysis. Our proposed method is a heterogeneous ensemble of three models that use Swin Transformers as backbone and the concepts of self-distillation and multi-task learning as core design choices. According to ablation studies performed with the CholecT45 challenge data via cross-validation, the biggest performance boost is achieved by the usage of soft labels obtained by self-distillation. External validation of our method on an independent test set was achieved by providing a Docker container of our inference model to the challenge organizers. According to their analysis, our method outperforms all other solutions submitted to the latest challenge in the field. Our approach thus shows the potential of self-distillation for becoming an important tool in medical image analysis applications. Code available at https://github.com/IMSY-DKFZ/self-distilled-swin.
更多查看译文
关键词
Surgical action recognition,Self-distillation,Laparoscopic surgery,Surgical workflow
AI 理解论文
溯源树
样例
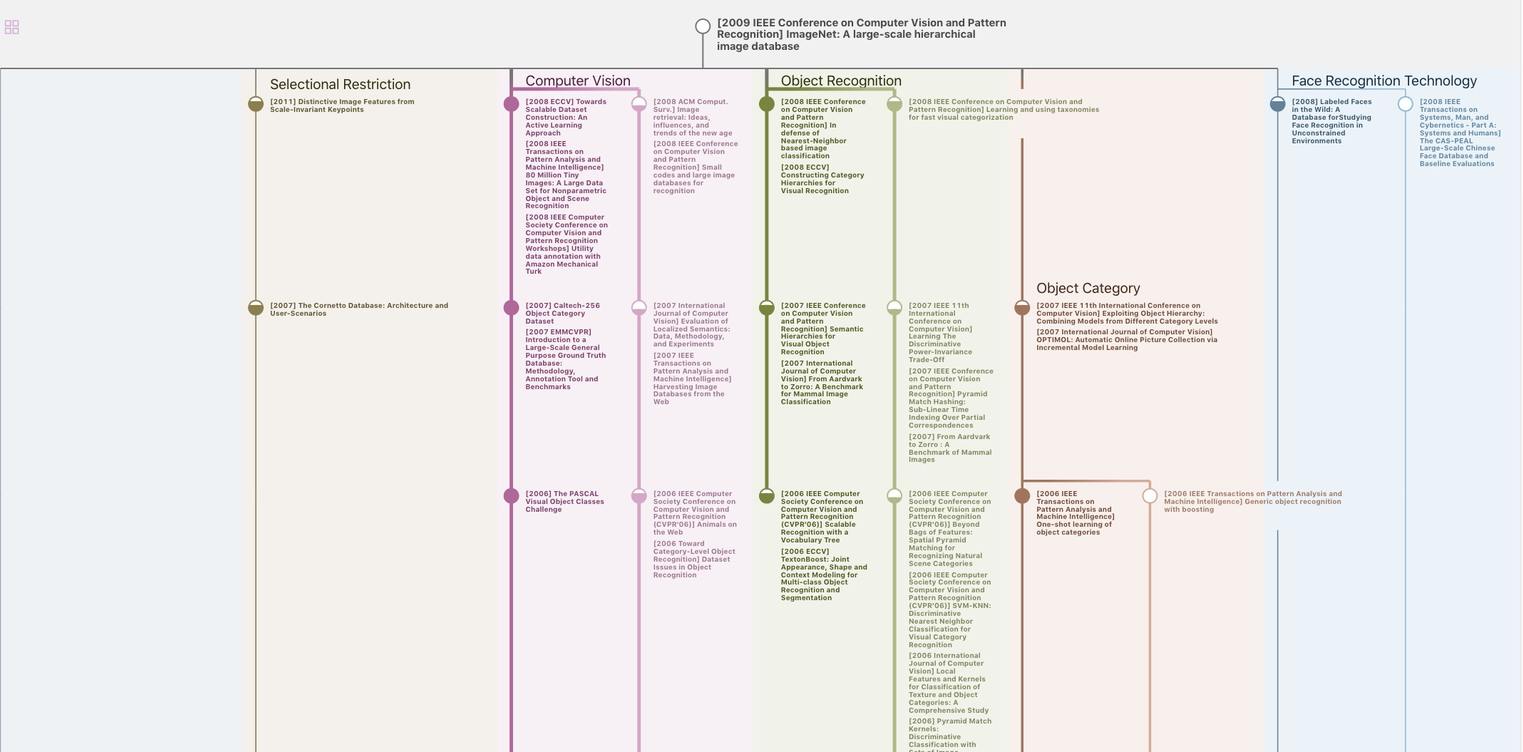
生成溯源树,研究论文发展脉络
Chat Paper
正在生成论文摘要