Infrared and visible image fusion via mutual information maximization
Computer Vision and Image Understanding(2023)
摘要
Traditional image fusion methods based on deep learning generally measure the similarity between the fusion results and the source images, ignoring the harmful information of source images. This paper presents a simple-yet-effective self-supervised image fusion optimization mechanism via directly maximizing the mutual information between the fused image and image samples, including positive and negative samples. The fusion optimization of positive samples has three steps, including visual fidelity item, quality perception item, and semantic perception item loss functions, aiming to reduce the distance between the fused representation and the real image quality. The fusion optimization of negative samples aims to enlarge the distance between the fusion results and the degraded image. Following InfoNCE, our framework is optimized via a surrogate contrastive loss, where the positive and negative selection underpins the real quality and visual fidelity information of fusion representation learning. Therefore, the stumbling blocks of deep learning in image fusion, i.e., similarity fusion optimization problems, are significantly mitigated. Extensive experiments demonstrate that fusion results neatly outperforms the state-of-the-art fusion optimization mechanisms in most metrics.
更多查看译文
关键词
Image fusion,Neural network,Mutual information,Deep learning
AI 理解论文
溯源树
样例
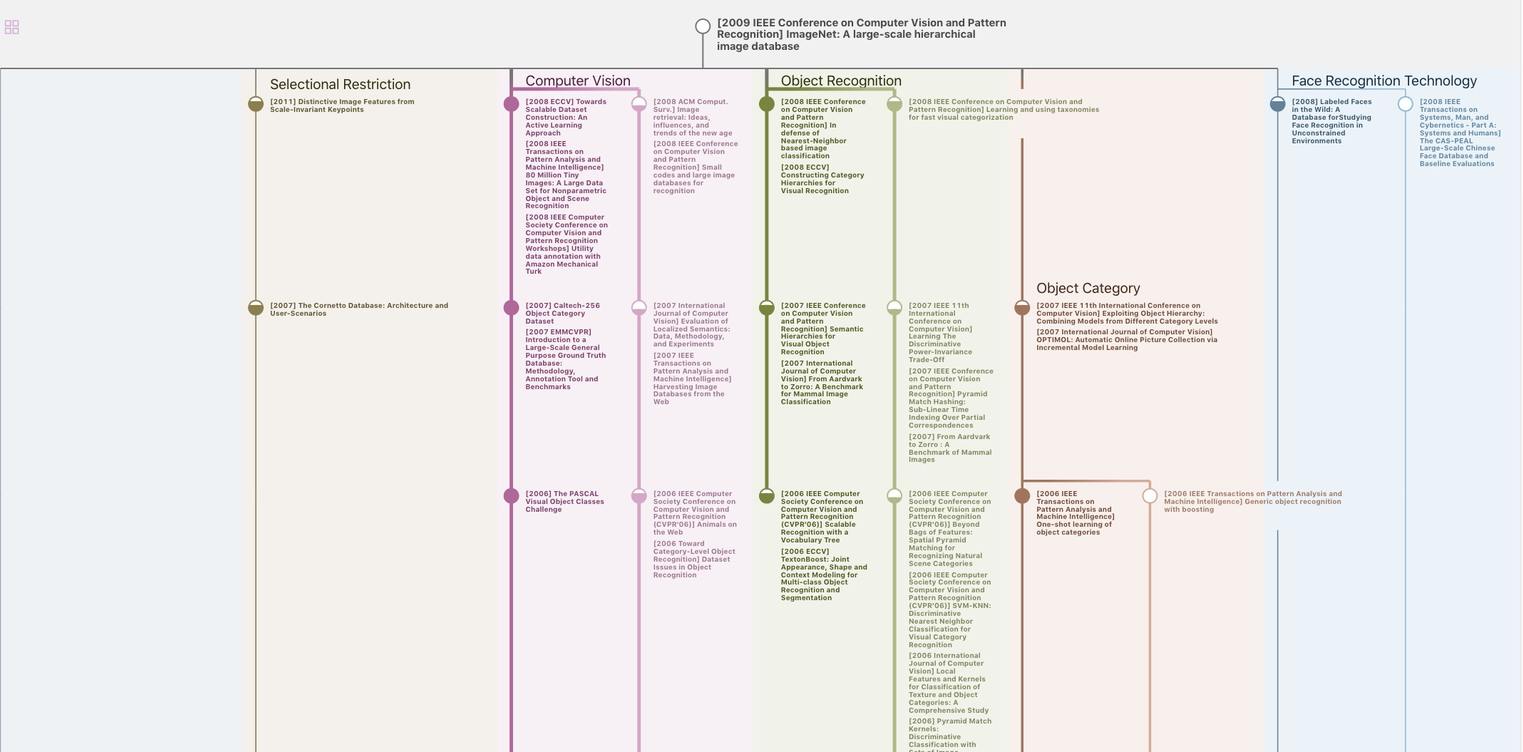
生成溯源树,研究论文发展脉络
Chat Paper
正在生成论文摘要