Matryoshka Policy Gradient for Entropy-Regularized RL: Convergence and Global Optimality
arxiv(2023)
摘要
A novel Policy Gradient (PG) algorithm, called Matryoshka Policy Gradient (MPG), is introduced and studied, in the context of max-entropy reinforcement learning, where an agent aims at maximising entropy bonuses additional to its cumulative rewards. MPG differs from standard PG in that it trains a sequence of policies to learn finite horizon tasks simultaneously, instead of a single policy for the single standard objective. For softmax policies, we prove convergence of MPG and global optimality of the limit by showing that the only critical point of the MPG objective is the optimal policy; these results hold true even in the case of continuous compact state space. MPG is intuitive, theoretically sound and we furthermore show that the optimal policy of the standard max-entropy objective can be approximated arbitrarily well by the optimal policy of the MPG framework. Finally, we justify that MPG is well suited when the policies are parametrized with neural networks and we provide an simple criterion to verify the global optimality of the policy at convergence. As a proof of concept, we evaluate numerically MPG on standard test benchmarks.
更多查看译文
关键词
gradient,entropy-regularized
AI 理解论文
溯源树
样例
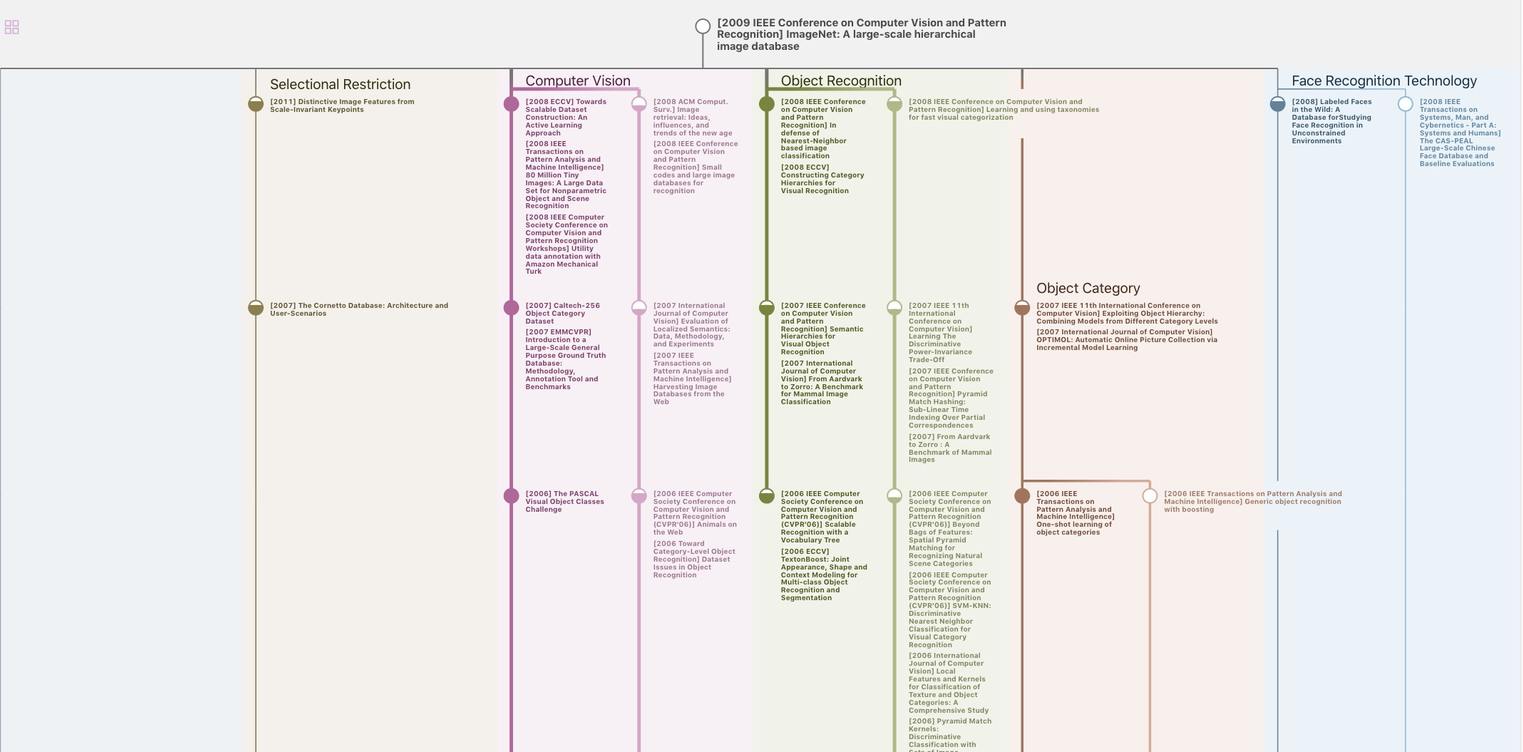
生成溯源树,研究论文发展脉络
Chat Paper
正在生成论文摘要