Deployment of Image Analysis Algorithms Under Prevalence Shifts
MEDICAL IMAGE COMPUTING AND COMPUTER ASSISTED INTERVENTION, MICCAI 2023, PT III(2023)
摘要
Domain gaps are among the most relevant roadblocks in the clinical translation of machine learning (ML)-based solutions for medical image analysis. While current research focuses on new training paradigms and network architectures, little attention is given to the specific effect of prevalence shifts on an algorithm deployed in practice. Such discrepancies between class frequencies in the data used for a method's development/validation and that in its deployment environment(s) are of great importance, for example in the context of artificial intelligence (AI) democratization, as disease prevalences may vary widely across time and location. Our contribution is twofold. First, we empirically demonstrate the potentially severe consequences of missing prevalence handling by analyzing (i) the extent of miscalibration, (ii) the deviation of the decision threshold from the optimum, and (iii) the ability of validation metrics to reflect neural network performance on the deployment population as a function of the discrepancy between development and deployment prevalence. Second, we propose a workflow for prevalence-aware image classification that uses estimated deployment prevalences to adjust a trained classifier to a new environment, without requiring additional annotated deployment data. Comprehensive experiments based on a diverse set of 30 medical classification tasks showcase the benefit of the proposed workflow in generating better classifier decisions and more reliable performance estimates compared to current practice.
更多查看译文
关键词
Prevalence shift,Medical image classification,Generalization,Domain Gap
AI 理解论文
溯源树
样例
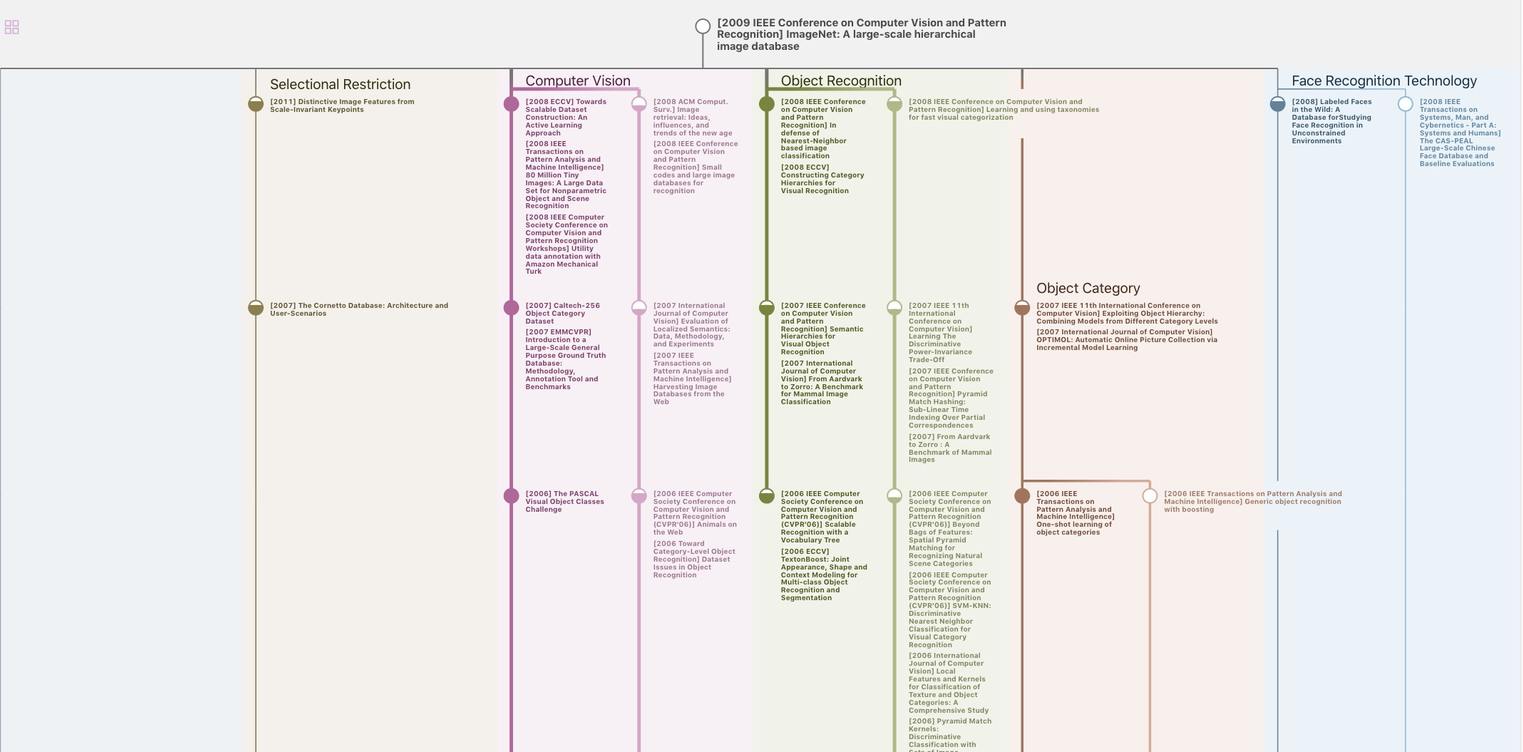
生成溯源树,研究论文发展脉络
Chat Paper
正在生成论文摘要