Multi-view clustering guided by unconstrained non-negative matrix factorization
Knowledge-Based Systems(2023)
摘要
Multi-view clustering based on non-negative matrix factorization (NMFMvC) is a well-known method for handling high-dimensional multi-view data. To satisfy the non-negativity constraint of the matrix, NMFMvC is usually solved using the Karush–Kuhn–Tucker (KKT) conditions. However, this optimization method is poorly scalable. To this end, we propose an unconstrained non-negative matrix factorization multi-view clustering (uNMFMvC) model. First, the objective function was constructed by decoupling the elements of the matrix and combining the elements with a non-linear mapping function in a non-negative value domain. The objective function was then optimized using the stochastic gradient descent (SGD) algorithm. Subsequently, three uNMFMvC methods were constructed based on different mapping functions and detailed reasoning was provided. Finally, experiments were conducted on eight public datasets and compared with cutting-edge multi-view clustering methods. The experimental results demonstrate that the proposed model has significant advantages.
更多查看译文
关键词
Non-negative matrix factorization,Multi-view clustering,Unconstrained,Element updates
AI 理解论文
溯源树
样例
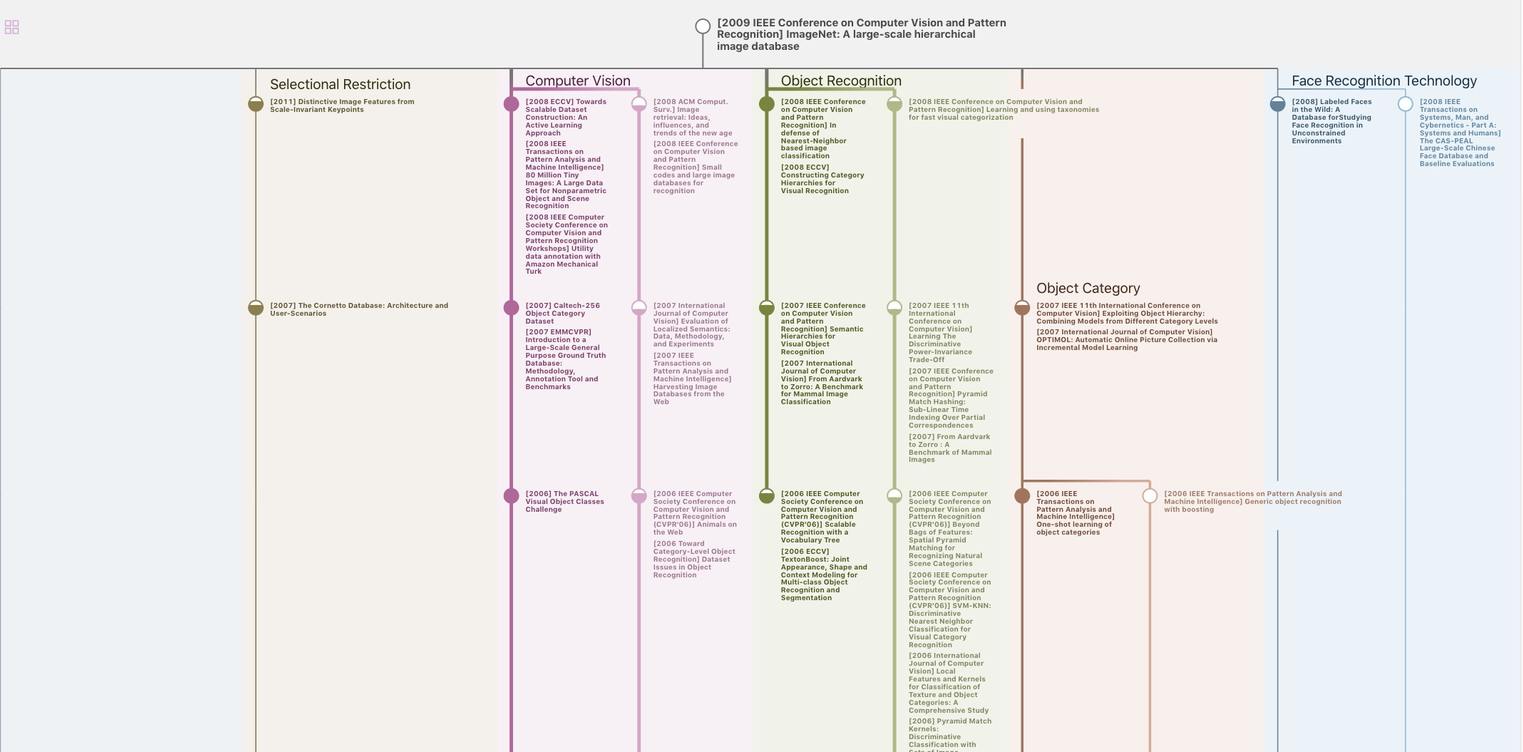
生成溯源树,研究论文发展脉络
Chat Paper
正在生成论文摘要