Heterogeneous-Branch Collaborative Learning for Dialogue Generation
arxiv(2023)
摘要
With the development of deep learning, advanced dialogue generation methods usually require a greater amount of computational resources. One promising approach to obtaining a high-performance and lightweight model is knowledge distillation, which relies heavily on the pre-trained powerful teacher. Collaborative learning, also known as online knowledge distillation, is an effective way to conduct one-stage group distillation in the absence of a well-trained large teacher model. However, previous work has a severe branch homogeneity problem due to the same training objective and the independent identical training sets. To alleviate this problem, we consider the dialogue attributes in the training of network branches. Each branch learns the attribute-related features based on the selected subset. Furthermore, we propose a dual group-based knowledge distillation method, consisting of positive distillation and negative distillation, to further diversify the features of different branches in a steadily and interpretable way. The proposed approach significantly improves branch heterogeneity and outperforms state-of-the-art collaborative learning methods on two widely used open-domain dialogue datasets.
更多查看译文
关键词
dialogue generation,learning,heterogeneous-branch
AI 理解论文
溯源树
样例
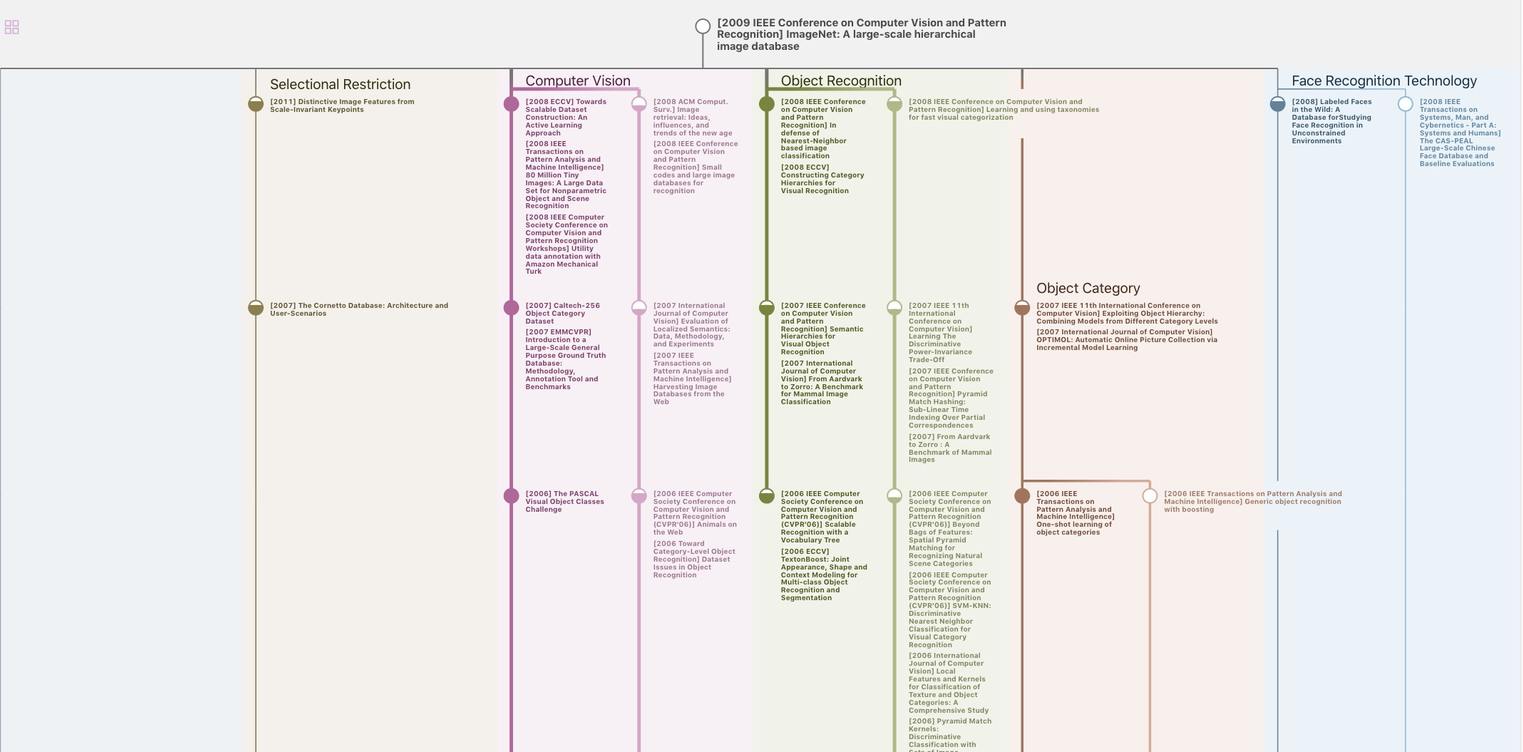
生成溯源树,研究论文发展脉络
Chat Paper
正在生成论文摘要